A deep learning-based model improves diagnosis of early gastric cancer under narrow band imaging endoscopy
Surgical Endoscopy(2022)
摘要
Background Diagnosis of early gastric cancer (EGC) under narrow band imaging endoscopy (NBI) is dependent on expertise and skills. We aimed to elucidate whether artificial intelligence (AI) could diagnose EGC under NBI and evaluate the diagnostic assistance of the AI system. Methods In this retrospective diagnostic study, 21,785 NBI images and 20 videos from five centers were divided into a training dataset (13,151 images, 810 patients), an internal validation dataset (7057 images, 283 patients), four external validation datasets (1577 images, 147 patients), and a video validation dataset (20 videos, 20 patients). All the images were labeled manually and used to train an AI system using You look only once v3 (YOLOv3). Next, the diagnostic performance of the AI system and endoscopists were compared and the diagnostic assistance of the AI system was assessed. The accuracy, sensitivity, specificity, and AUC were primary outcomes. Results The AI system diagnosed EGCs on validation datasets with AUCs of 0.888–0.951 and diagnosed all the EGCs (100.0%) in video dataset. The AI system achieved better diagnostic performance (accuracy, 93.2%, 95% CI, 90.0–94.9%) than senior (85.9%, 95% CI, 84.2–87.4%) and junior (79.5%, 95% CI, 77.8–81.0%) endoscopists. The AI system significantly enhanced the performance of endoscopists in senior (89.4%, 95% CI, 87.9–90.7%) and junior (84.9%, 95% CI, 83.4–86.3%) endoscopists. Conclusion The NBI AI system outperformed the endoscopists and exerted potential assistant impact in EGC identification. Prospective validations are needed to evaluate the clinical reinforce of the system in real clinical practice. Graphical abstract
更多查看译文
关键词
Artificial intelligence, Early gastric cancer, Narrow band imaging, Deep convolutional neural network
AI 理解论文
溯源树
样例
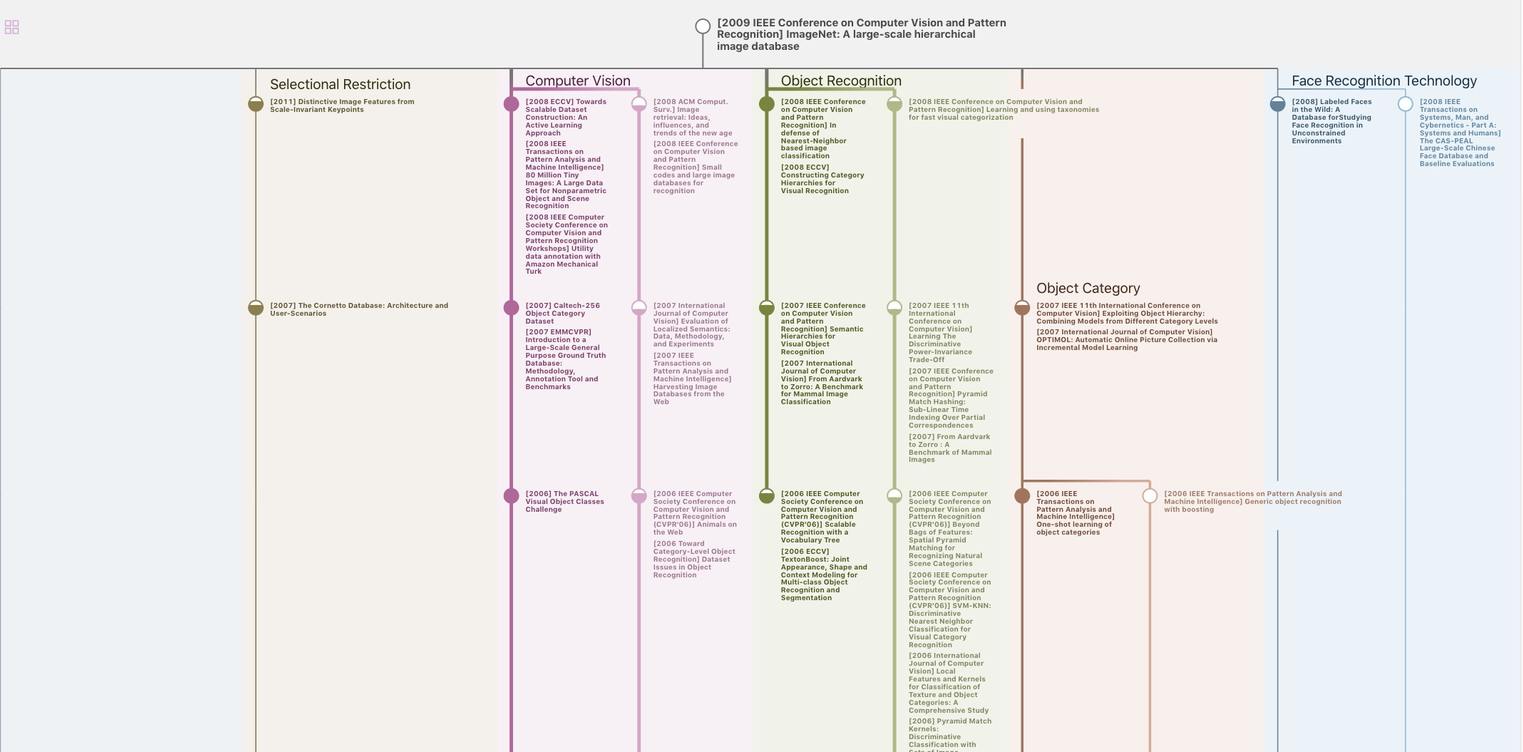
生成溯源树,研究论文发展脉络
Chat Paper
正在生成论文摘要