A robust and lightweight deep attention multiple instance learning algorithm for predicting genetic alterations.
Computerized medical imaging and graphics : the official journal of the Computerized Medical Imaging Society(2023)
摘要
Self-attention mechanism-based algorithms are attractive in digital pathology due to their interpretability, but suffer from computation complexity. This paper presents a novel, lightweight Attention-based Multiple Instance Mutation Learning (AMIML) model to allow small-scale attention operations for predicting gene mutations. Compared to the standard self-attention model, AMIML reduces the number of model parameters by approximately 70%. Using data for 24 clinically relevant genes from four cancer cohorts in TCGA studies (UCEC, BRCA, GBM, and KIRC), we compare AMIML with a standard self-attention model, five other deep learning models, and four traditional machine learning models. The results show that AMIML has excellent robustness and outperforms all the baseline algorithms in the vast majority of the tested genes. Conversely, the performance of the reference deep learning and machine learning models vary across different genes, and produce suboptimal prediction for certain genes. Furthermore, with the flexible and interpretable attention-based pooling mechanism, AMIML can further zero in and detect predictive image patches.
更多查看译文
关键词
Attention mechanism,Deep learning,Gene Mutation,Mutiple Instance Learning,Whole slide images
AI 理解论文
溯源树
样例
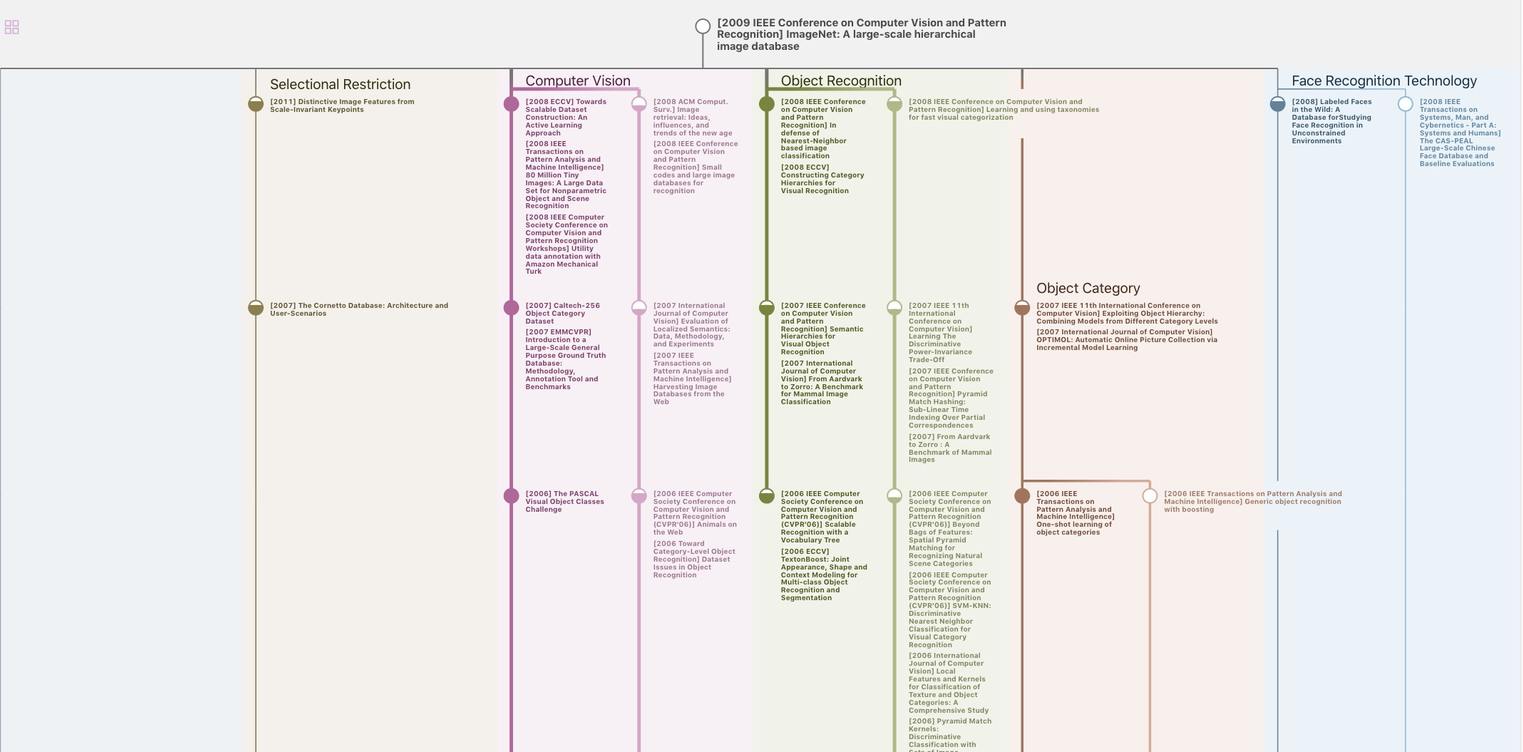
生成溯源树,研究论文发展脉络
Chat Paper
正在生成论文摘要