AdaVITS: Tiny VITS for Low Computing Resource Speaker Adaptation
2022 13th International Symposium on Chinese Spoken Language Processing (ISCSLP)(2022)
摘要
Speaker adaptation in text-to-speech synthesis (TTS) is to fine-tune a pre-trained TTS model to adapt to new target speakers with limited data. While much effort has been conducted towards this task, seldom work has been performed for low computational resource scenarios due to the challenges raised by the requirement of the lightweight model and less computational complexity. In this paper, a tiny VITS-based [1] TTS model, named AdaVITS, for low computing resource speaker adaptation is proposed. To effectively reduce the parameters and computational complexity of VITS, an inverse short-time Fourier transform (iSTFT)-based wave construction decoder is proposed to replace the upsampling-based decoder which is resource-consuming in the original VITS. Besides, NanoFlow is introduced to share the density estimate across flow blocks to reduce the parameters of the prior encoder. Furthermore, to reduce the computational complexity of the textual encoder, scaled-dot attention is replaced with linear attention. To deal with the instability caused by the simplified model, we use phonetic posteriorgram (PPG) as a frame-level linguistic feature for supervising the model process from phoneme to spectrum. Experiments show that AdaVITS can generate stable and natural speech in speaker adaptation with 8. 97M model parameters and 0.72 GFlops computational complexity.
1
更多查看译文
关键词
speaker adaptation,low computing resource,adversarial learning,normalizing flows
AI 理解论文
溯源树
样例
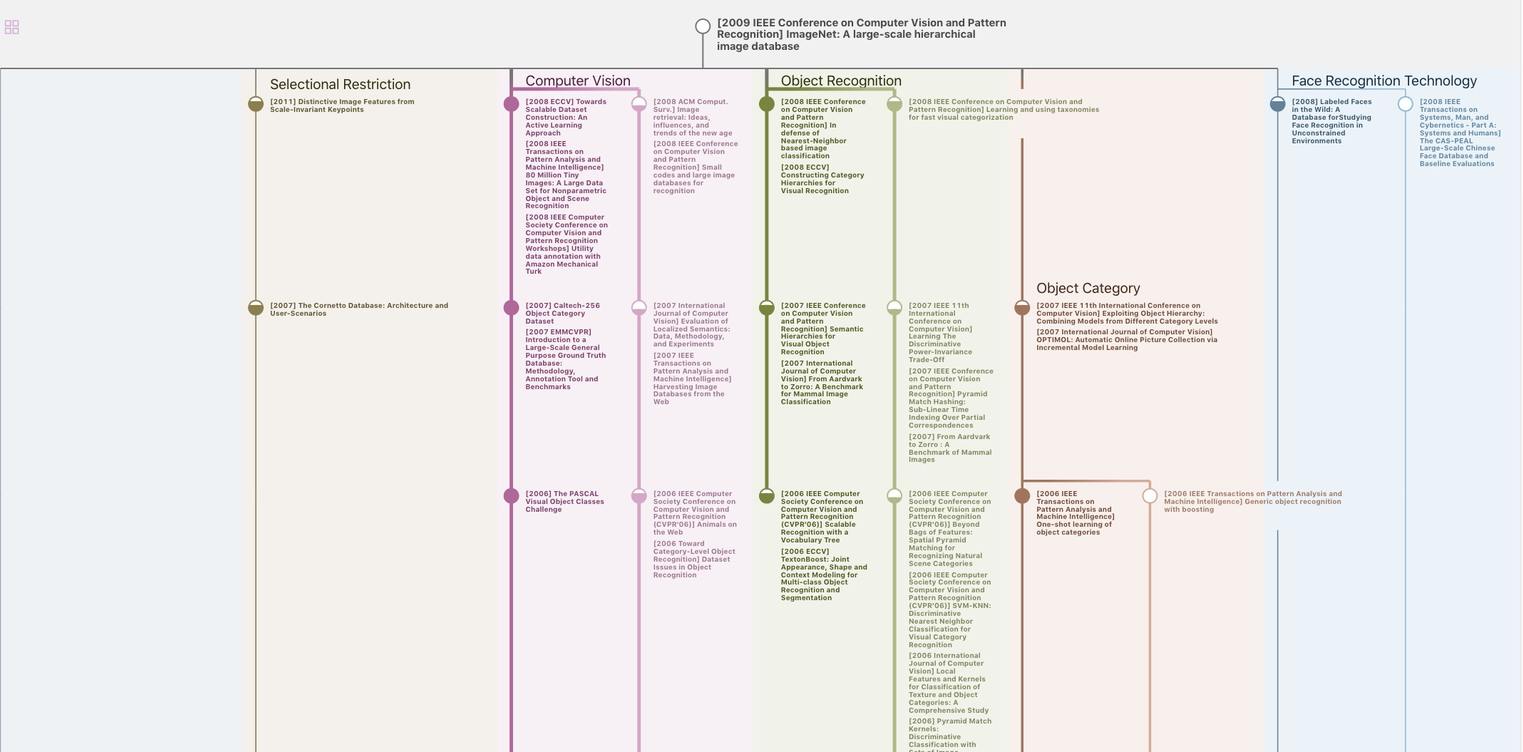
生成溯源树,研究论文发展脉络
Chat Paper
正在生成论文摘要