CASSOCK: Viable Backdoor Attacks against DNN in the Wall of Source-Specific Backdoor Defenses.
arxiv(2023)
摘要
As a critical threat to deep neural networks (DNNs), backdoor attacks can be categorized into two types, i.e., source-agnostic backdoor attacks (SABAs) and source-specific backdoor attacks (SSBAs). Compared to traditional SABAs, SSBAs are more advanced in that they have superior stealthier in bypassing mainstream countermeasures that are effective against SABAs. Nonetheless, existing SSBAs suffer from two major limitations. First, they can hardly achieve a good trade-off between ASR (attack success rate) and FPR (false positive rate). Besides, they can be effectively detected by the state-of-the-art (SOTA) countermeasures (e.g., SCAn [40]). To address the limitations above, we propose a new class of viable source-specific backdoor attacks coined as CASSOCK. Our key insight is that trigger designs when creating poisoned data and cover data in SSBAs play a crucial role in demonstrating a viable source-specific attack, which has not been considered by existing SSBAs. With this insight, we focus on trigger transparency and content when crafting triggers for poisoned dataset where a sample has an attacker-targeted label and cover dataset where a sample has a ground-truth label. Specifically, we implement CASSOCK(Trans) that designs a trigger with heterogeneous transparency to craft poisoned and cover datasets, presenting better attack performance than existing SSBAs. We also propose CASSOCK(Cont) that extracts salient features of the attacker-targeted label to generate a trigger, entangling the trigger features with normal features of the label, which is stealthier in bypassing the SOTA defenses. While both CASSOCK(Trans) and CASSOCK(Cont) are orthogonal, they are complementary to each other, generating a more powerful attack, called CASSOCK(Comp), with further improved attack performance and stealthiness. To demonstrate their viability, we perform a comprehensive evaluation of the three CASSOCK-based attacks on four popular datasets (i.e., MNIST, CIFAR10, GTSRB and LFW) and three SOTA defenses (i.e., extended Neural Cleanse [45], Februus [8], and SCAn [40]). Compared with a representative SSBA as a baseline (SSBA(Base)), CASSOCK-based attacks have significantly advanced the attack performance, i.e., higher ASR and lower FPR with comparable CDA (clean data accuracy). Besides, CASSOCK-based attacks have effectively bypassed the SOTA defenses, and SSBA(Base) cannot.
更多查看译文
关键词
Deep Neural Network, Source-Specific Backdoor, Trigger Transparency, and Content
AI 理解论文
溯源树
样例
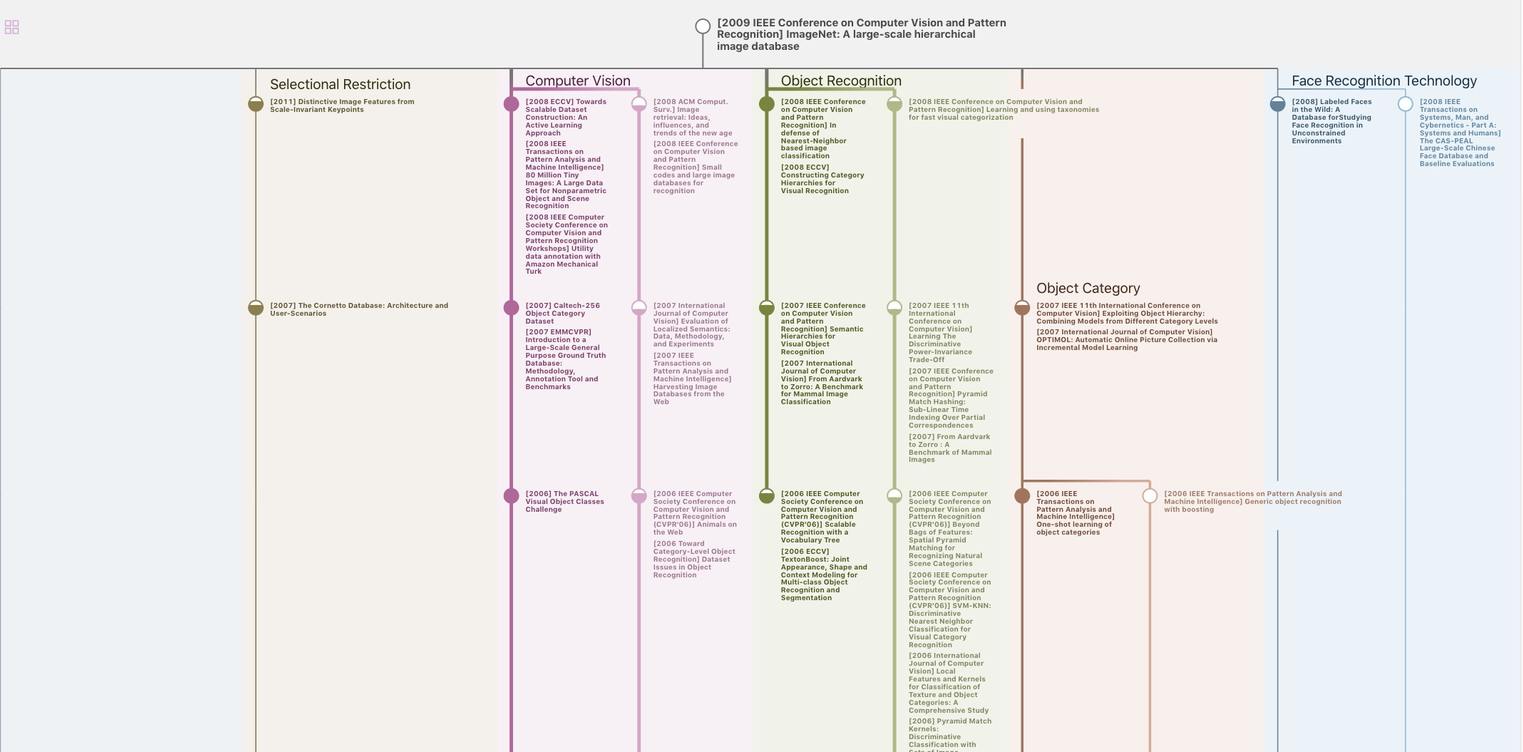
生成溯源树,研究论文发展脉络
Chat Paper
正在生成论文摘要