ThoraciNet: thoracic abnormality detection and disease classification using fusion DCNNs
Physical and Engineering Sciences in Medicine(2022)
摘要
Chest X-rays are arguably the de facto medical imaging technique for diagnosing thoracic abnormalities. Chest X-ray analysis is complex, especially in asymptomatic diseases, and relies heavily on the expertise of radiologists. This work proposes the use of deep learning models to automate the process of thoracic abnormality detection, classification, and segmentation. The advent of large-scale, annotated and public chest X-ray databases have enabled deep learning researchers to build state-of-the-art computer-aided diagnosis systems for such tasks. In this work, a two-stage pipeline is proposed for thoracic abnormality detection and disease classification using chest X-rays. Two fusion-based models are proposed for disease classification, using two asymmetric, deep convolutional neural networks. Results are evaluated over NIH database covering multiple patients’ X-rays with metrics such as accuracy and AUC scores. The proposed architecture outperforms the existing ones, achieving AUC scores of 0.99 for CXR triaging and 0.79 for CXR disease classification. Furthermore, GradCAM visualization is performed to validate the results, rendering model predictions interpretable to experts and end-users.
更多查看译文
关键词
Disease classification, Deep learning, Medical triaging, Chest X-ray, Thoracic abnormalities
AI 理解论文
溯源树
样例
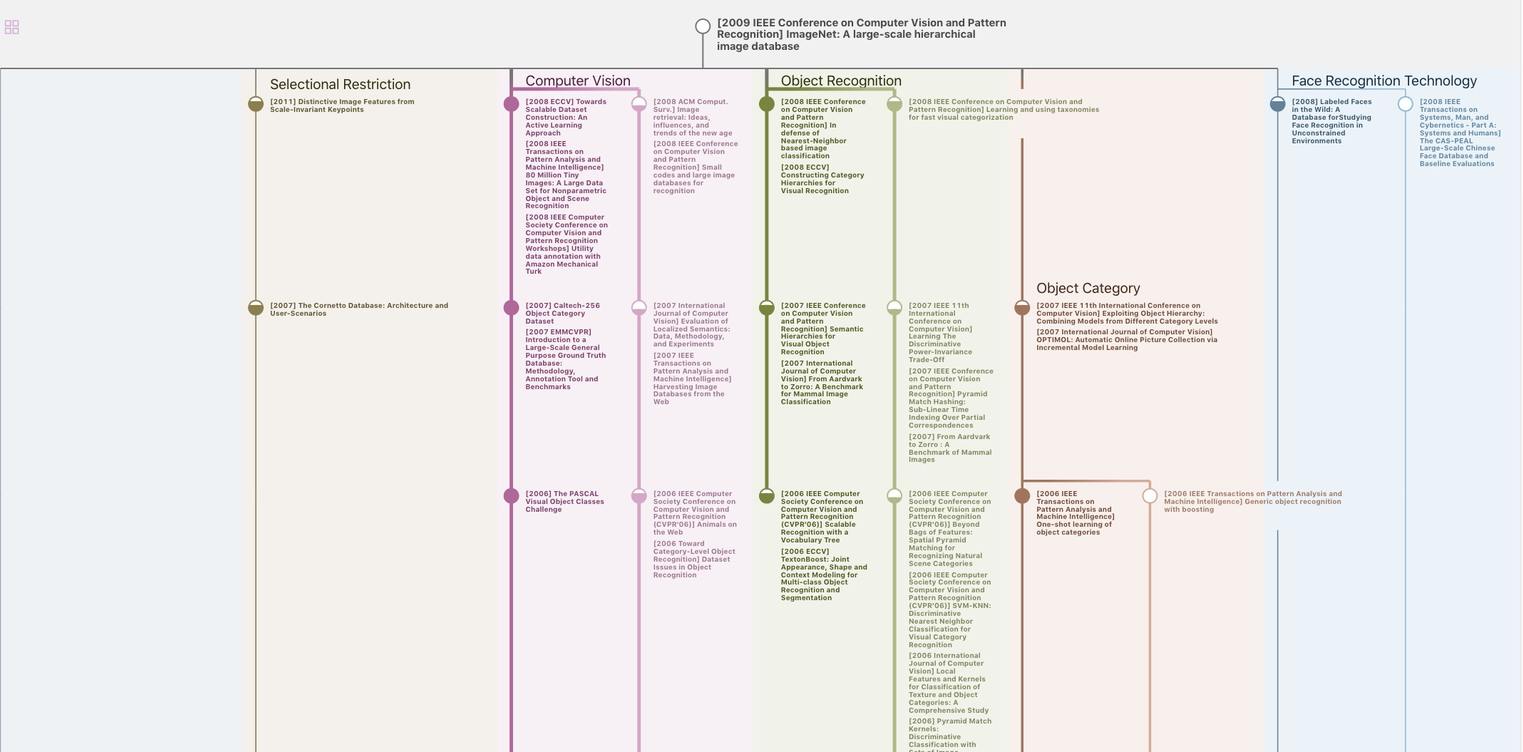
生成溯源树,研究论文发展脉络
Chat Paper
正在生成论文摘要