From Keypoints to Object Landmarks via Self-Training Correspondence: A novel approach to Unsupervised Landmark Discovery
arxiv(2023)
摘要
This paper proposes a novel paradigm for the unsupervised learning of object landmark detectors. Contrary to existing methods that build on auxiliary tasks such as image generation or equivariance, we propose a self-training approach where, departing from generic keypoints, a landmark detector and descriptor is trained to improve itself, tuning the keypoints into distinctive landmarks. To this end, we propose an iterative algorithm that alternates between producing new pseudo-labels through feature clustering and learning distinctive features for each pseudo-class through contrastive learning. With a shared backbone for the landmark detector and descriptor, the keypoint locations progressively converge to stable landmarks, filtering those less stable. Compared to previous works, our approach can learn points that are more flexible in terms of capturing large viewpoint changes. We validate our method on a variety of difficult datasets, including LS3D, BBCPose, Human3.6M and PennAction, achieving new state of the art results.
更多查看译文
关键词
Detectors, Task analysis, Strain, Semantics, Faces, Unsupervised learning, Training, Unsupervised landmark discovery, self-training, clustering, correspondence, keypoints
AI 理解论文
溯源树
样例
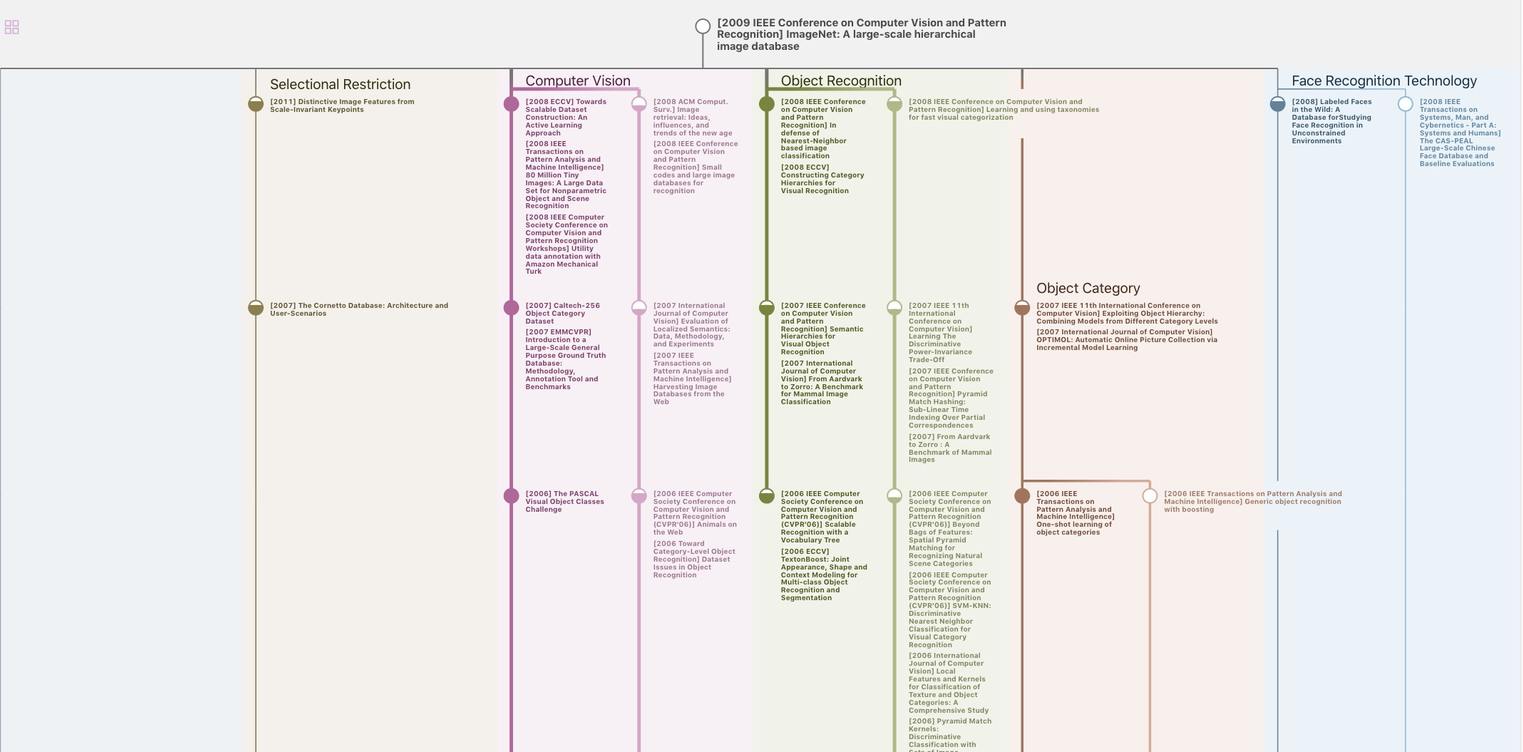
生成溯源树,研究论文发展脉络
Chat Paper
正在生成论文摘要