Transformers for Multi-Object Tracking on Point Clouds
2022 IEEE INTELLIGENT VEHICLES SYMPOSIUM (IV)(2022)
Abstract
We present TransMOT, a novel transformer-based end-to-end trainable online tracker and detector for point cloud data. The model utilizes a cross- and a self-attention mechanism and is applicable to lidar data in an automotive context, as well as other data types, such as radar. Both track management and the detection of new tracks are performed by the same transformer decoder module and the tracker state is encoded in feature space. With this approach, we make use of the rich latent space of the detector for tracking rather than relying on low-dimensional bounding boxes. Still, we are able to retain some of the desirable properties of traditional Kalman-filter based approaches, such as an ability to handle sensor input at arbitrary timesteps or to compensate frame skips. This is possible due to a novel module that transforms the track information from one frame to the next on feature-level and thereby fulfills a similar task as the prediction step of a Kalman filter. Results are presented on the challenging real-world dataset nuScenes, where the proposed model outperforms its Kalman filter-based tracking baseline.
MoreTranslated text
Key words
transformers,multiobject tracking,point clouds,TransMOT,novel transformer-based end-to-end trainable,point cloud data,self-attention mechanism,lidar data,automotive context,data types,track management,transformer decoder module,tracker state,feature space,rich latent space,low-dimensional bounding boxes,traditional Kalman-filter,track information,feature-level,Kalman filter-based
AI Read Science
Must-Reading Tree
Example
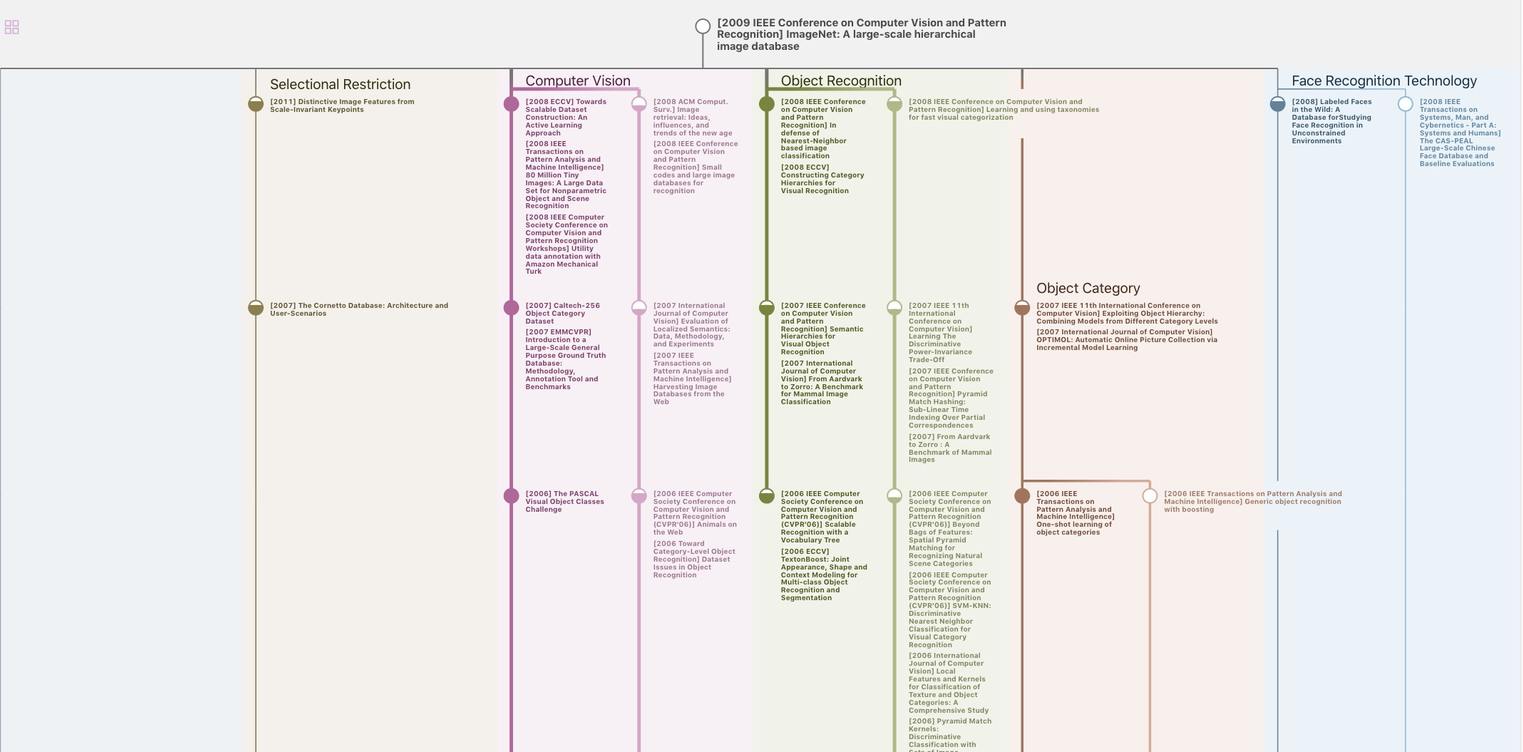
Generate MRT to find the research sequence of this paper
Chat Paper
Summary is being generated by the instructions you defined