Comparison of Prediction Models Based on Machine Learning for the Compressive Strength Estimation of Recycled Aggregate Concrete
MATERIALS(2022)
摘要
Numerous tests are used to determine the performance of concrete, but compressive strength (CS) is usually regarded as the most important. The recycled aggregate concrete (RAC) exhibits lower CS compared to natural aggregate concrete. Several variables, such as the water-cement ratio, the strength of the parent concrete, recycled aggregate replacement ratio, density, and water absorption of recycled aggregate, all impact the RAC's CS. Many studies have been carried out to ascertain the influence of each of these elements separately. However, it is difficult to investigate their combined effect on the CS of RAC experimentally. Experimental investigations entail casting, curing, and testing samples, which require considerable work, expense, and time. It is vital to adopt novel methods to the stated aim in order to conduct research quickly and efficiently. The CS of RAC was predicted in this research utilizing machine learning techniques like decision tree, gradient boosting, and bagging regressor. The data set included eight input variables, and their effect on the CS of RAC was evaluated. Coefficient correlation (R-2), the variance between predicted and experimental outcomes, statistical checks, and k-fold evaluations, were carried out to validate and compare the models. With an R-2 of 0.92, the bagging regressor technique surpassed the decision tree and gradient boosting in predicting the strength of RAC. The statistical assessments also validated the superior accuracy of the bagging regressor model, yielding lower error values like mean absolute error (MAE) and root mean square error (RMSE). MAE and RMSE values for the bagging model were 4.258 and 5.693, respectively, which were lower than the other techniques employed, i.e., gradient boosting (MAE = 4.956 and RMSE = 7.046) and decision tree (MAE = 6.389 and RMSE = 8.952). Hence, the bagging regressor is the best suitable technique to predict the CS of RAC.
更多查看译文
关键词
recycled concrete aggregate, compressive strength, green concrete, machine learning, decision tree, gradient boosting, bagging regressor
AI 理解论文
溯源树
样例
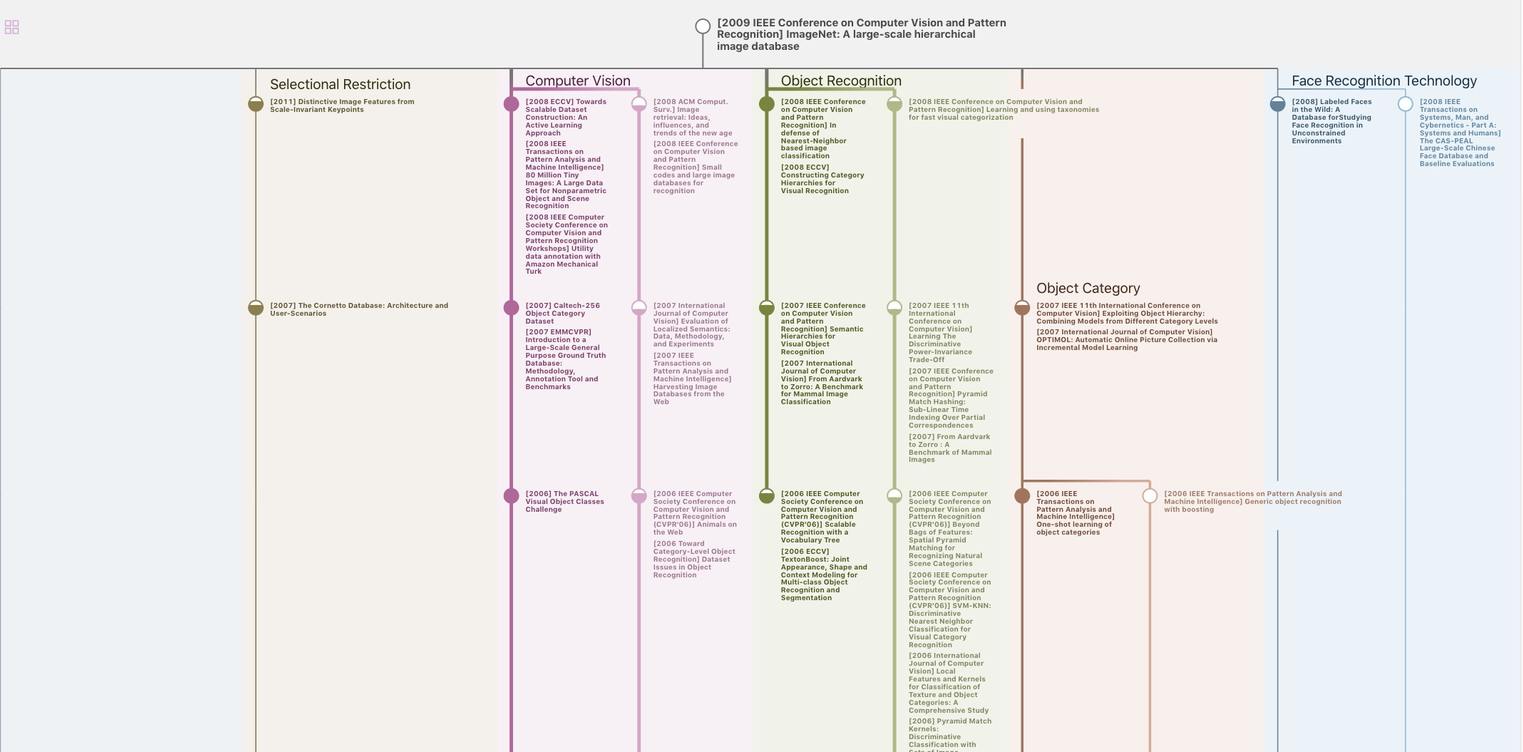
生成溯源树,研究论文发展脉络
Chat Paper
正在生成论文摘要