WAVE: Edge-Device Cooperated Real-Time Object Detection for Open-Air Applications
user-61447a76e55422cecdaf7d19(2023)
摘要
CNN based real-time object detection can facilitate various AI applications that need to understand the surroundings via camera, such as autonomous package delivery robots, augmented reality, and intelligent drone applications. Currently, due to the high computation cost of CNN, accurate real-time object detection is only possible when mobile devices can upload video frames to powerful edge servers through high-speed wireless networks like WiFi. However, for many open-air AI applications, the network conditions (such as cellular networks) are usually unfavorable, far from satisfying the network demands of state-of-the-art systems. In this paper, we focus on the challenges incurred by mobile communication networks and propose WAVEcontaining three novel techniques, which are Deep RoI Encoding, Prioritized Parallel Offloading and Fine-grained Offloading Strategy, to realize real-time, robust and low-cost object detection for open-air AI applications. The experimental results show that under LTE networks, WAVE realizes high-accuracy real-time object detection and face recognition and significantly outperforms state-of-the-art systems.
更多查看译文
关键词
Edge computing,real-time object detection,mobile communication network
AI 理解论文
溯源树
样例
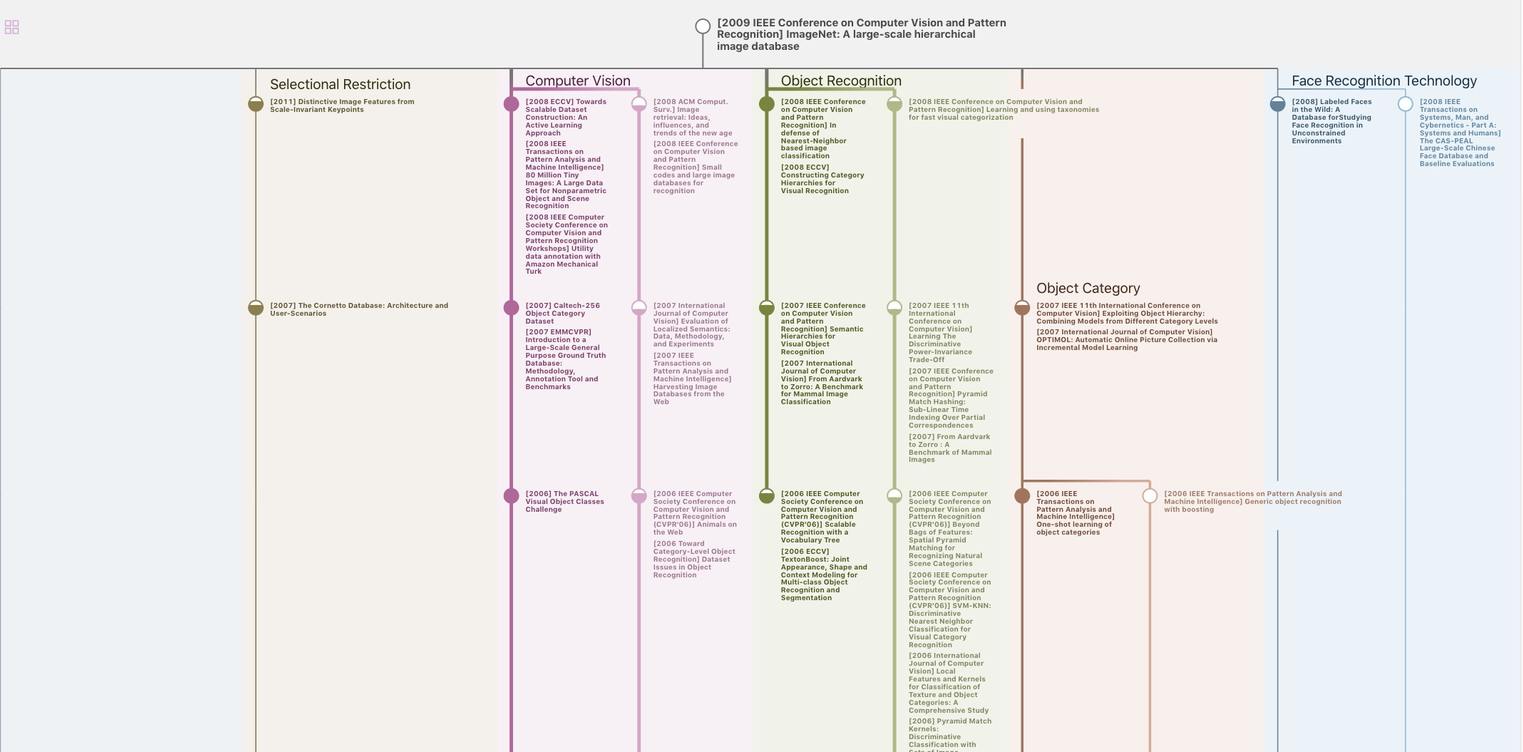
生成溯源树,研究论文发展脉络
Chat Paper
正在生成论文摘要