Trine: Cloud-Edge-Device Cooperated Real-time Video Analysis for Household Applications
user-61447a76e55422cecdaf7d19(2022)
摘要
Real-time mobile video analysis like object detection and tracking is key to various household applications such as AR, cognitive assistance and smart home. Such applications rely on heavy DNN models, which are not suitable for mobile devices due to resource limitation. The long latency of cloud offloading is unacceptable for the real-time requirements, and the direct edge offloading relies on powerful edge servers, which is impractical for household scenarios. To solve this challenge, we take advantage of the computing devices that are low-cost or already exist in our lives, and propose Trine, a cloud-edge-device cooperated framework, in which complicated computation tasks are offloaded from the device to the cloud with the edge as the key bond to coordinate. In addition, due to the heterogeneity of edge devices, which leads to no one-fits-all algorithm that is optimal in all situations, we propose a profile-based algorithm to customize trackers for various edge devices. We implemented Trine on an android phone and three edge devices. The experiments demonstrate that Trine achieves 8-36% higher real-time accuracy and 25-89% higher robustness than state-of-the-art.
更多查看译文
关键词
Cloud-edge-device,edge computing,mobile video analytics,real-time video analytics
AI 理解论文
溯源树
样例
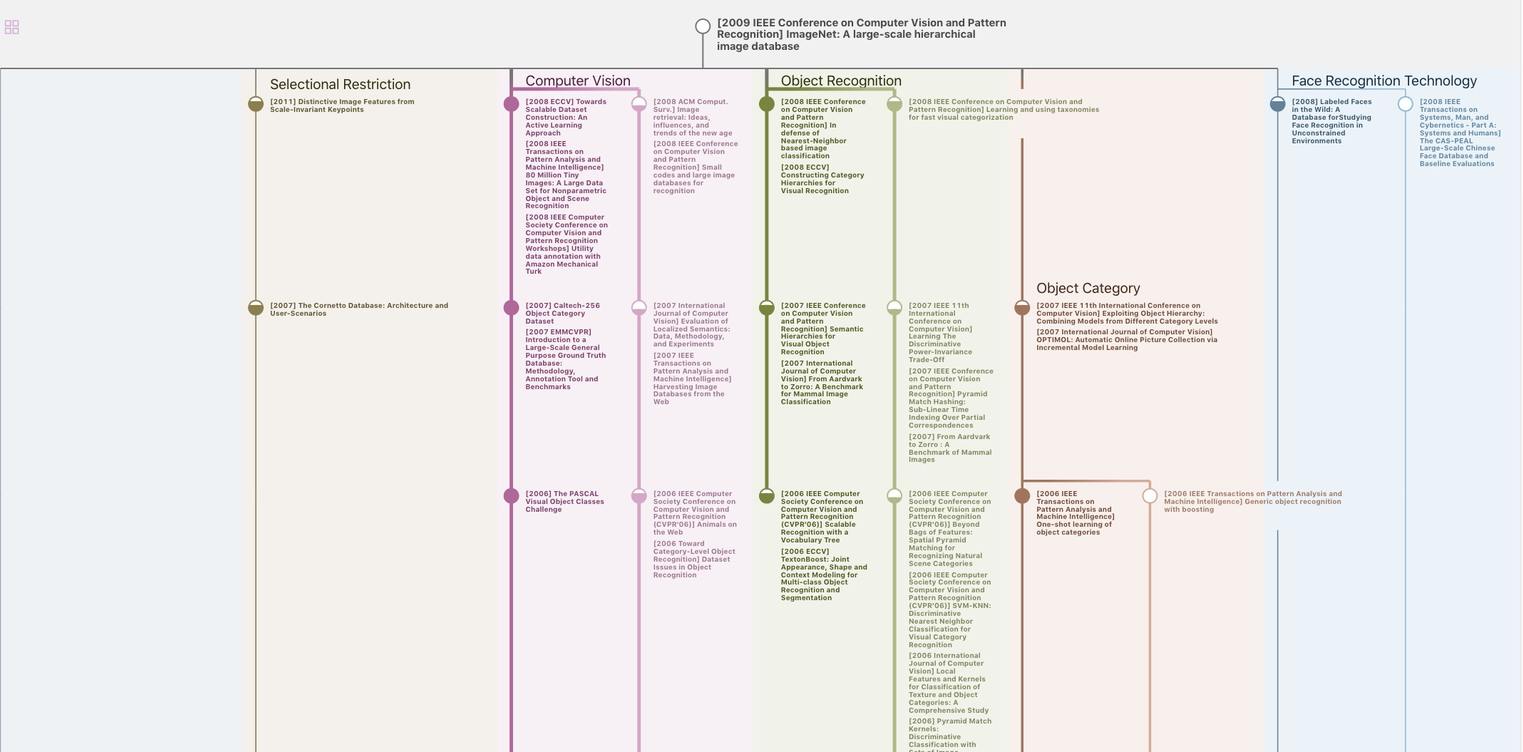
生成溯源树,研究论文发展脉络
Chat Paper
正在生成论文摘要