Evaluating Automated Driving Planner Robustness against Adversarial Influence
arxiv(2022)
摘要
Evaluating the robustness of automated driving planners is a critical and challenging task. Although methodologies to evaluate vehicles are well established, they do not yet account for a reality in which vehicles with autonomous components share the road with adversarial agents. Our approach, based on probabilistic trust models, aims to help researchers assess the robustness of protections for machine learning-enabled planners against adversarial influence. In contrast with established practices that evaluate safety using the same evaluation dataset for all vehicles, we argue that adversarial evaluation fundamentally requires a process that seeks to defeat a specific protection. Hence, we propose that evaluations be based on estimating the difficulty for an adversary to determine conditions that effectively induce unsafe behavior. This type of inference requires precise statements about threats, protections, and aspects of planning decisions to be guarded. We demonstrate our approach by evaluating protections for planners relying on camera-based object detectors.
更多查看译文
关键词
automated driving planner robustness,adversarial
AI 理解论文
溯源树
样例
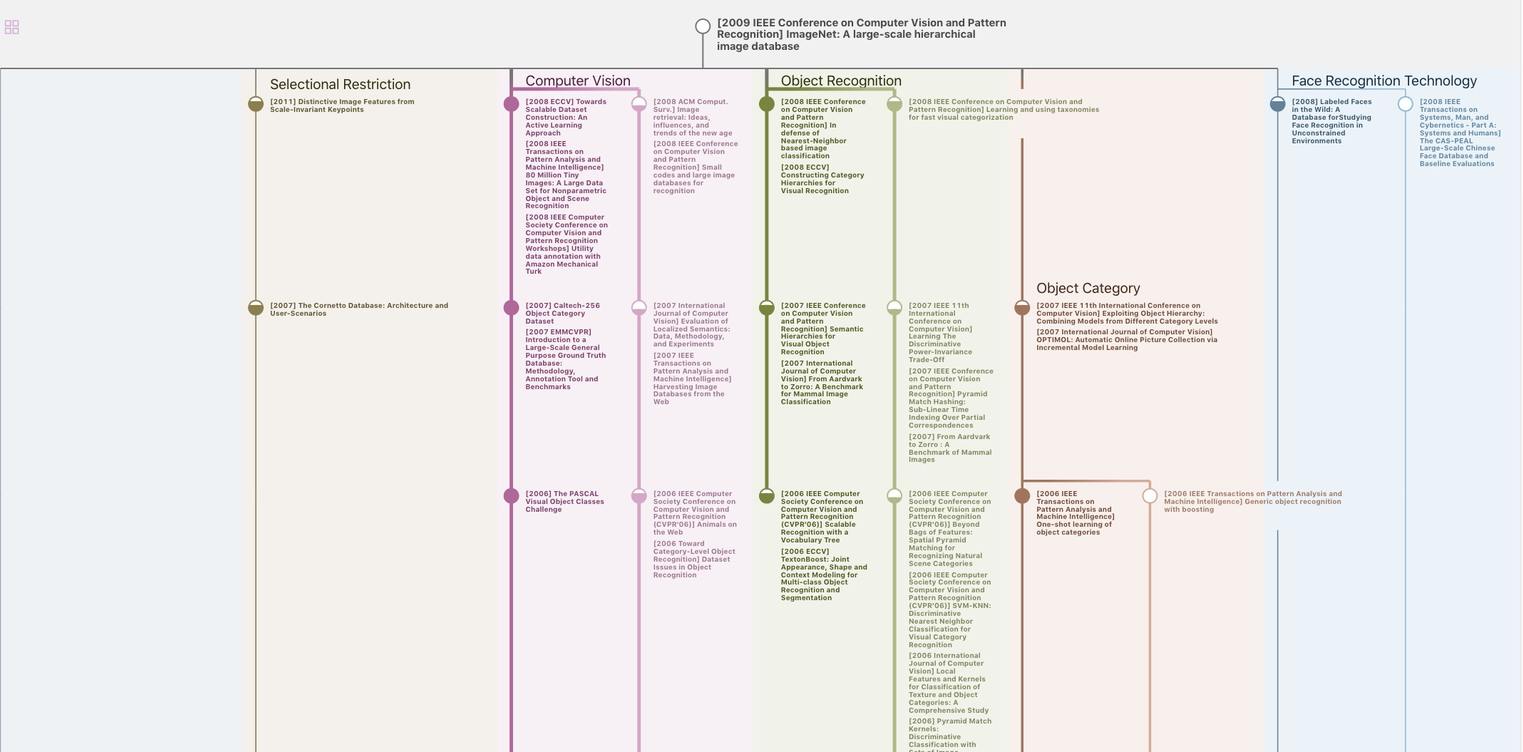
生成溯源树,研究论文发展脉络
Chat Paper
正在生成论文摘要