Heterogeneous Data-Centric Architectures for Modern Data-Intensive Applications: Case Studies in Machine Learning and Databases
2022 IEEE Computer Society Annual Symposium on VLSI (ISVLSI)(2022)
摘要
Today's computing systems require moving data back-and-forth between computing resources (e.g., CPUs, GPUs, accelerators) and off-chip main memory so that computation can take place on the data. Unfortunately, this data movement is a major bottleneck for system performance and energy consumption [1], [2]. One promising execution paradigm that alleviates the data movement bottleneck in modern and emerging applications is processing-in-memory (PIM) [2]–[12], where the cost of data movement to/from main memory is reduced by placing computation capabilities close to memory. In the data-centric PIM paradigm, the logic close to memory has access to data with significantly higher memory bandwidth, lower latency, and lower energy consumption than processors/accelerators in existing processor-centric systems.
更多查看译文
关键词
processing in memory,databases,machine learning,neural networks,accelerator
AI 理解论文
溯源树
样例
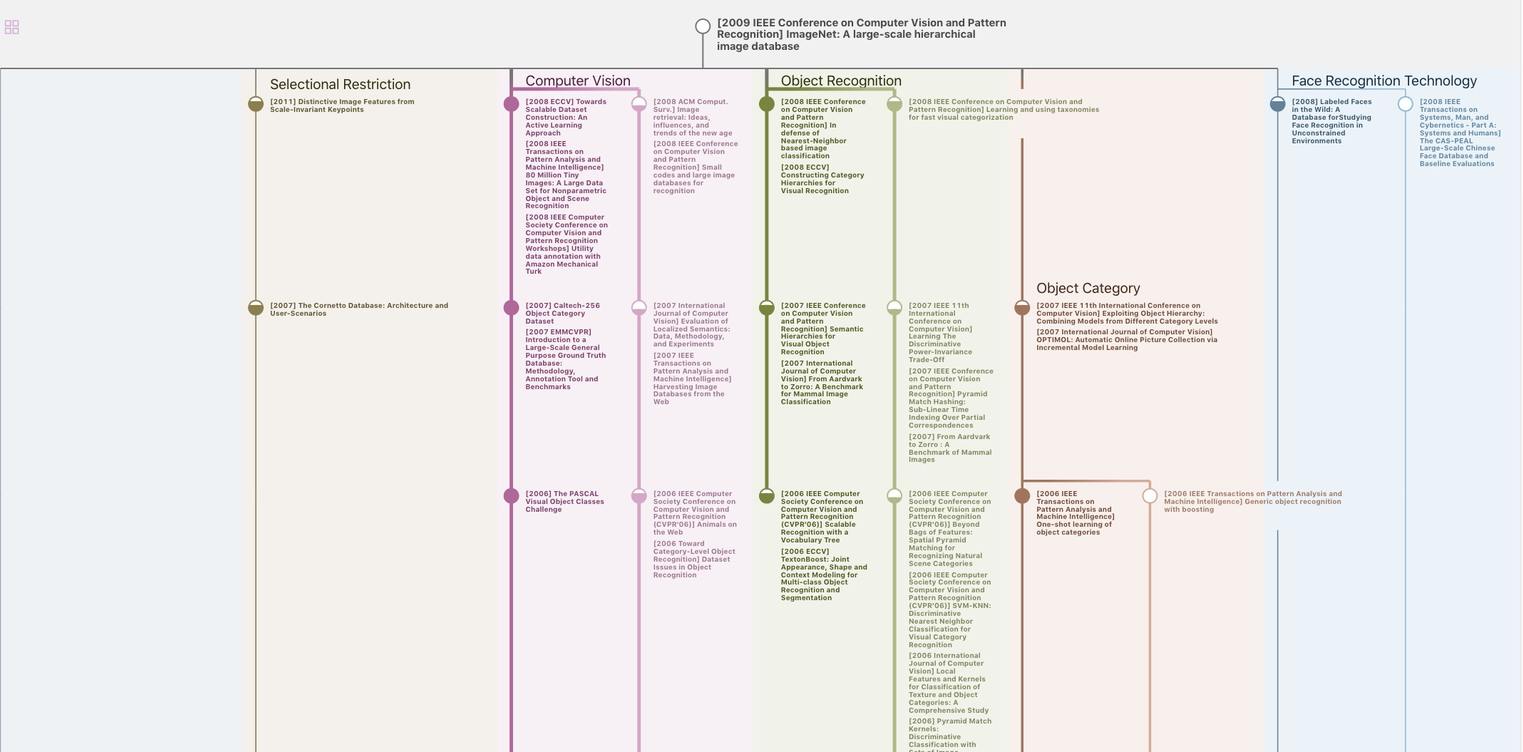
生成溯源树,研究论文发展脉络
Chat Paper
正在生成论文摘要