SupMAE: Supervised Masked Autoencoders Are Efficient Vision Learners
arxiv(2022)
摘要
Recently, self-supervised Masked Autoencoders (MAE) have attracted unprecedented attention for their impressive representation learning ability. However, the pretext task, Masked Image Modeling (MIM), reconstructs the missing local patches, lacking the global understanding of the image. This paper extends MAE to a fully-supervised setting by adding a supervised classification branch, thereby enabling MAE to effectively learn global features from golden labels. The proposed Supervised MAE (SupMAE) only exploits a visible subset of image patches for classification, unlike the standard supervised pre-training where all image patches are used. Through experiments, we demonstrate that not only is SupMAE more training efficient but also it learns more robust and transferable features. Specifically, SupMAE achieves comparable performance with MAE using only 30% of compute when evaluated on ImageNet with the ViT-B/16 model. SupMAE's robustness on ImageNet variants and transfer learning performance outperforms MAE and standard supervised pre-training counterparts. Code will be made publicly available.
更多查看译文
关键词
supervised masked autoencoders,vision,learners
AI 理解论文
溯源树
样例
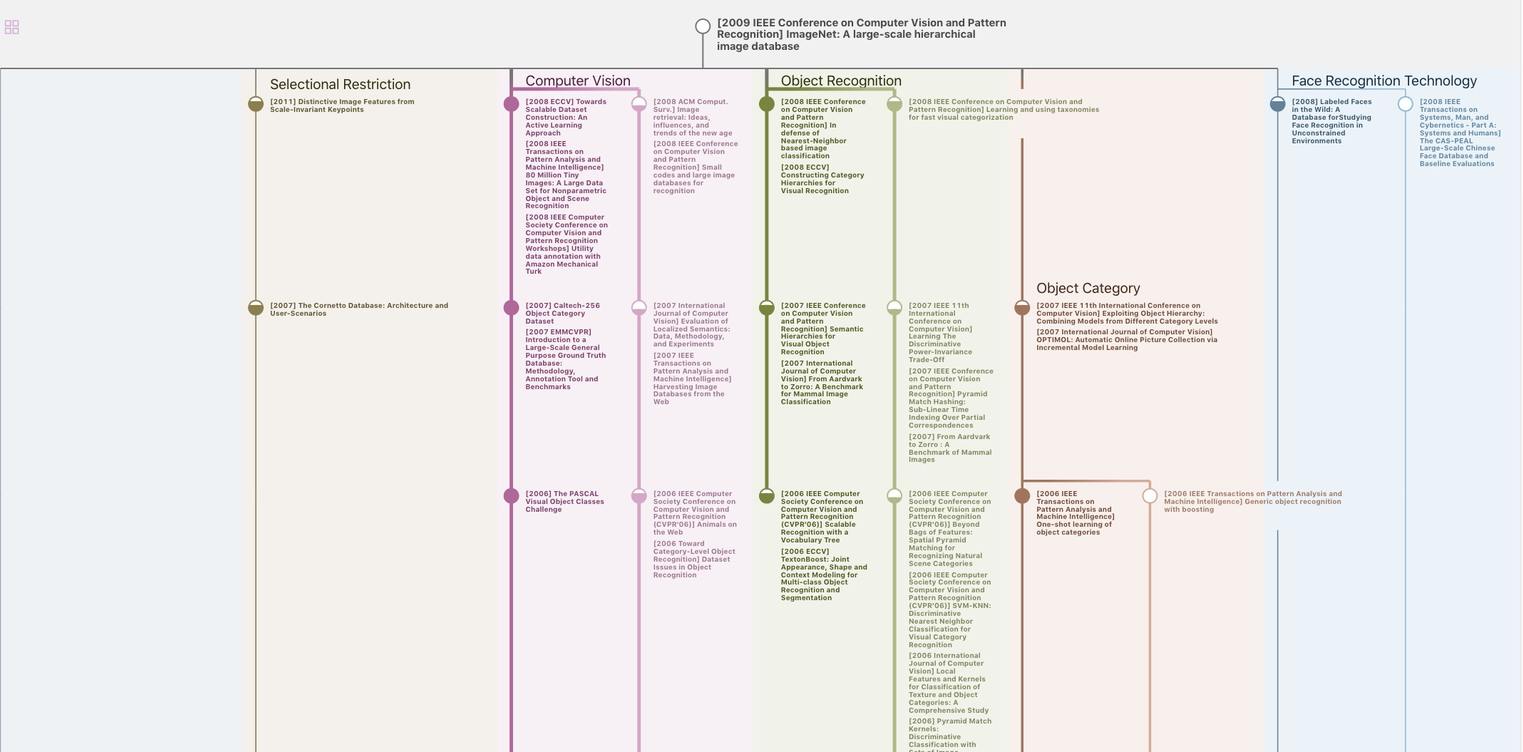
生成溯源树,研究论文发展脉络
Chat Paper
正在生成论文摘要