Controllable Text Generation with Neurally-Decomposed Oracle
NeurIPS 2022(2022)
摘要
We propose a general and efficient framework to control auto-regressive generation models with NeurAlly-Decomposed Oracle (NADO). Given a pre-trained base language model and a sequence-level boolean oracle function, we propose to decompose the oracle function into token-level guidance to steer the base model in text generation. Specifically, the token-level guidance is approximated by a neural model trained with examples sampled from the base model, demanding no additional auxiliary labeled data. Based on posterior regularization, we present the closed-form optimal solution to incorporate the token-level guidance into the base model for controllable generation. We further provide a theoretical analysis of how the approximation quality of NADO affects the controllable generation results. Experiments conducted on two applications: (1) text generation with lexical constraints and (2) machine translation with formality control demonstrate that our framework efficiently guides the base model towards the given oracle while maintaining high generation quality.
更多查看译文
关键词
Controllable text generation,constrained decoding
AI 理解论文
溯源树
样例
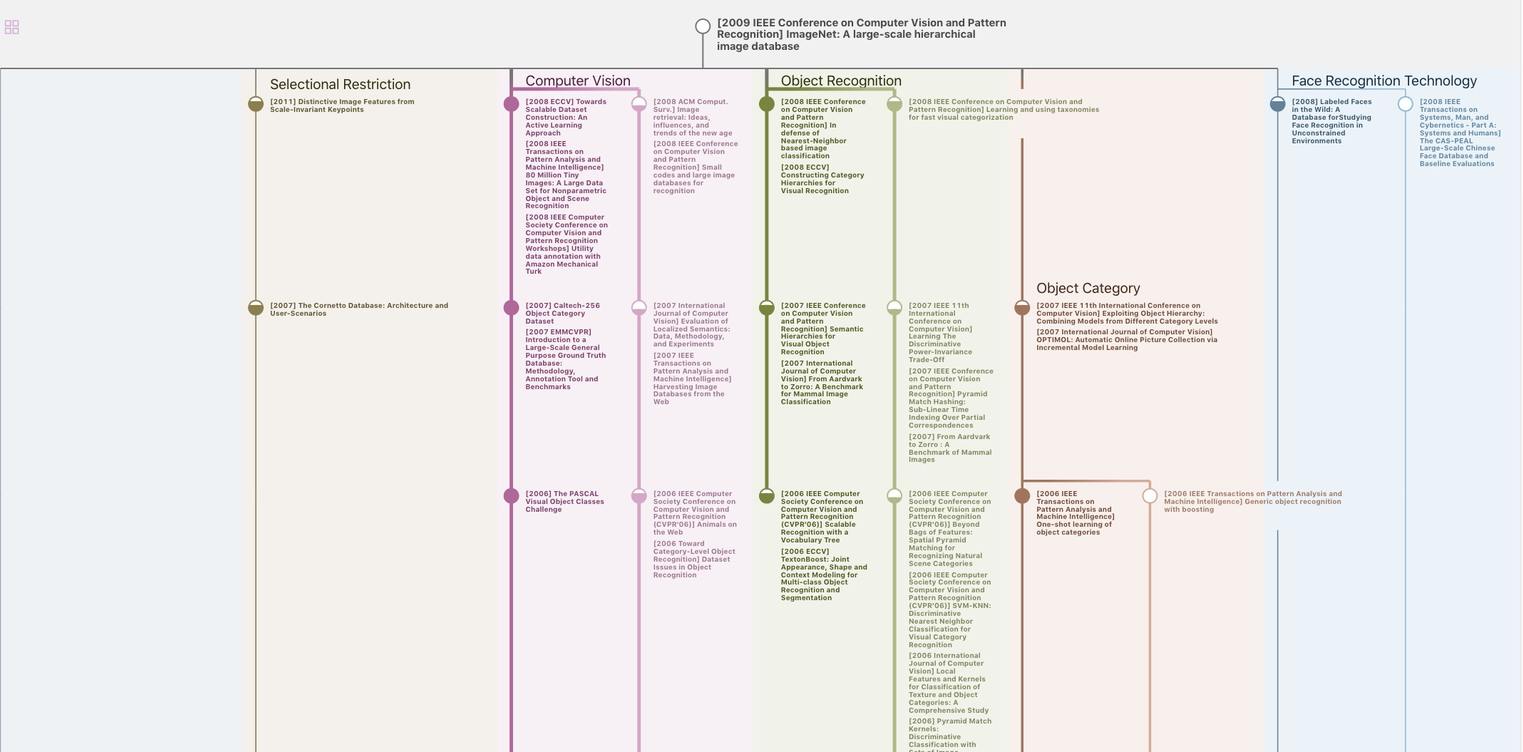
生成溯源树,研究论文发展脉络
Chat Paper
正在生成论文摘要