Internal Model-Based Online Optimization
IEEE TRANSACTIONS ON AUTOMATIC CONTROL(2024)
摘要
In this article, we propose a model-based approach to the design of online optimization algorithms, with the goal of improving the tracking of the solution trajectory (trajectories) w.r.t. state-of-the-art methods. We focus first on quadratic problems with a time-varying linear term, and use digital control tools (a robust internal model principle) to propose a novel online algorithm that can achieve zero tracking error by modeling the cost with a dynamical system. We prove the convergence of the algorithm for both strongly convex and convex problems. We further discuss the sensitivity of the proposed method to model uncertainties and quantify its performance. We discuss how the proposed algorithm can be applied to general (nonquadratic) problems using an approximate model of the cost, and analyze the convergence leveraging the small gain theorem. We present numerical results that showcase the superior performance of the proposed algorithms over previous methods for both quadratic and nonquadratic problems.
更多查看译文
关键词
Signal processing algorithms,Optimization,Heuristic algorithms,Costs,Trajectory,Convergence,Approximation algorithms,Digital control,online gradient descent,online optimization,robust control,structured algorithms
AI 理解论文
溯源树
样例
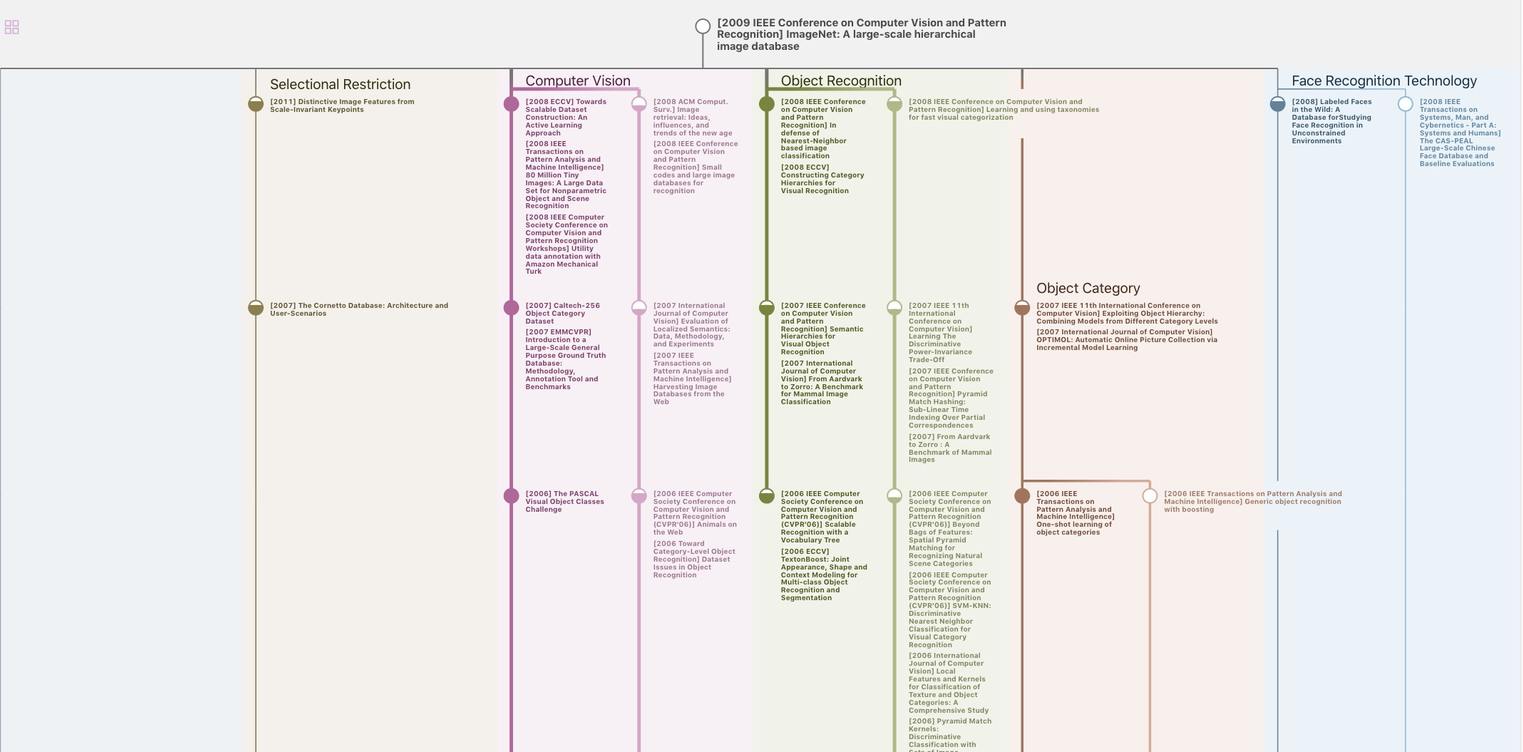
生成溯源树,研究论文发展脉络
Chat Paper
正在生成论文摘要