MissDAG: Causal Discovery in the Presence of Missing Data with Continuous Additive Noise Models
NeurIPS 2022(2022)
摘要
State-of-the-art causal discovery methods usually assume that the observational data is complete. However, the missing data problem is pervasive in many practical scenarios such as clinical trials, economics, and biology. One straightforward way to address the missing data problem is first to impute the data using off-the-shelf imputation methods and then apply existing causal discovery methods. However, such a two-step method may suffer from suboptimality, as the imputation algorithm is unaware of the causal discovery step. In this paper, we develop a general method, which we call MissDAG, to perform causal discovery from data with incomplete observations. Focusing mainly on the assumptions of ignorable missingness and the identifiable additive noise models (ANMs), MissDAG maximizes the expected likelihood of the visible part of observations under the expectation-maximization (EM) framework. In the E-step, in cases where computing the posterior distributions of parameters in closed-form is not feasible, Monte Carlo EM is leveraged to approximate the likelihood. In the M-step, MissDAG leverages the density transformation to model the noise distributions with simpler and specific formulations by virtue of the ANMs and uses a likelihood-based causal discovery algorithm with directed acyclic graph prior as an inductive bias. We demonstrate the flexibility of MissDAG for incorporating various causal discovery algorithms and its efficacy through extensive simulations and real data experiments.
更多查看译文
关键词
Causal discovery,Incomplete data,Additive noise models,EM method,Monte Carlo sampling
AI 理解论文
溯源树
样例
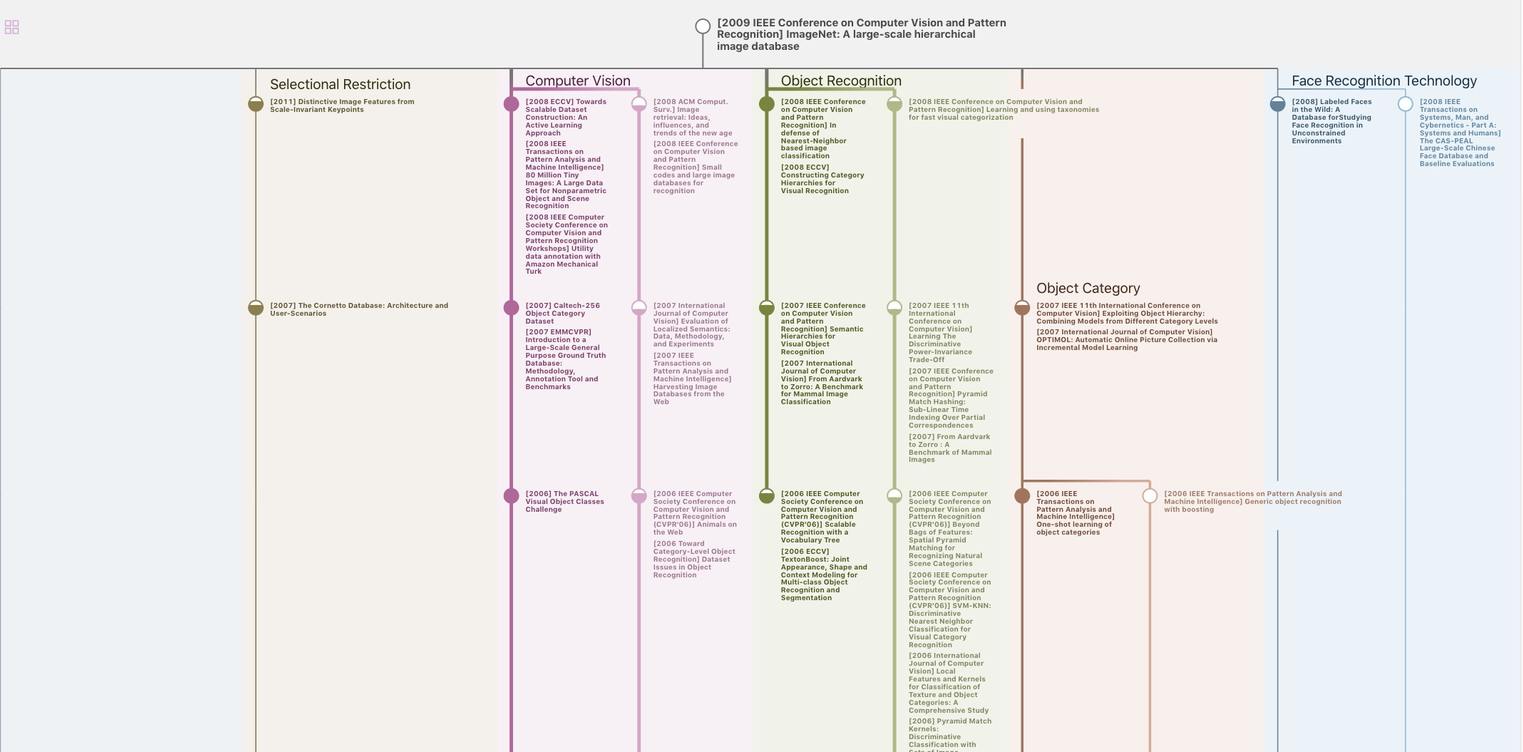
生成溯源树,研究论文发展脉络
Chat Paper
正在生成论文摘要