Global Convergence of Over-parameterized Deep Equilibrium Models
arxiv(2023)
摘要
A deep equilibrium model (DEQ) is implicitly defined through an equilibrium point of an infinite-depth weight-tied model with an input-injection. Instead of infinite computations, it solves an equilibrium point directly with root-finding and computes gradients with implicit differentiation. The training dynamics of over-parameterized DEQs are investigated in this study. By supposing a condition on the initial equilibrium point, we show that the unique equilibrium point always exists during the training process, and the gradient descent is proved to converge to a globally optimal solution at a linear convergence rate for the quadratic loss function. In order to show that the required initial condition is satisfied via mild over-parameterization, we perform a fine-grained analysis on random DEQs. We propose a novel probabilistic framework to overcome the technical difficulty in the non-asymptotic analysis of infinite-depth weight-tied models.
更多查看译文
关键词
global convergence,models,deep,over-parameterized
AI 理解论文
溯源树
样例
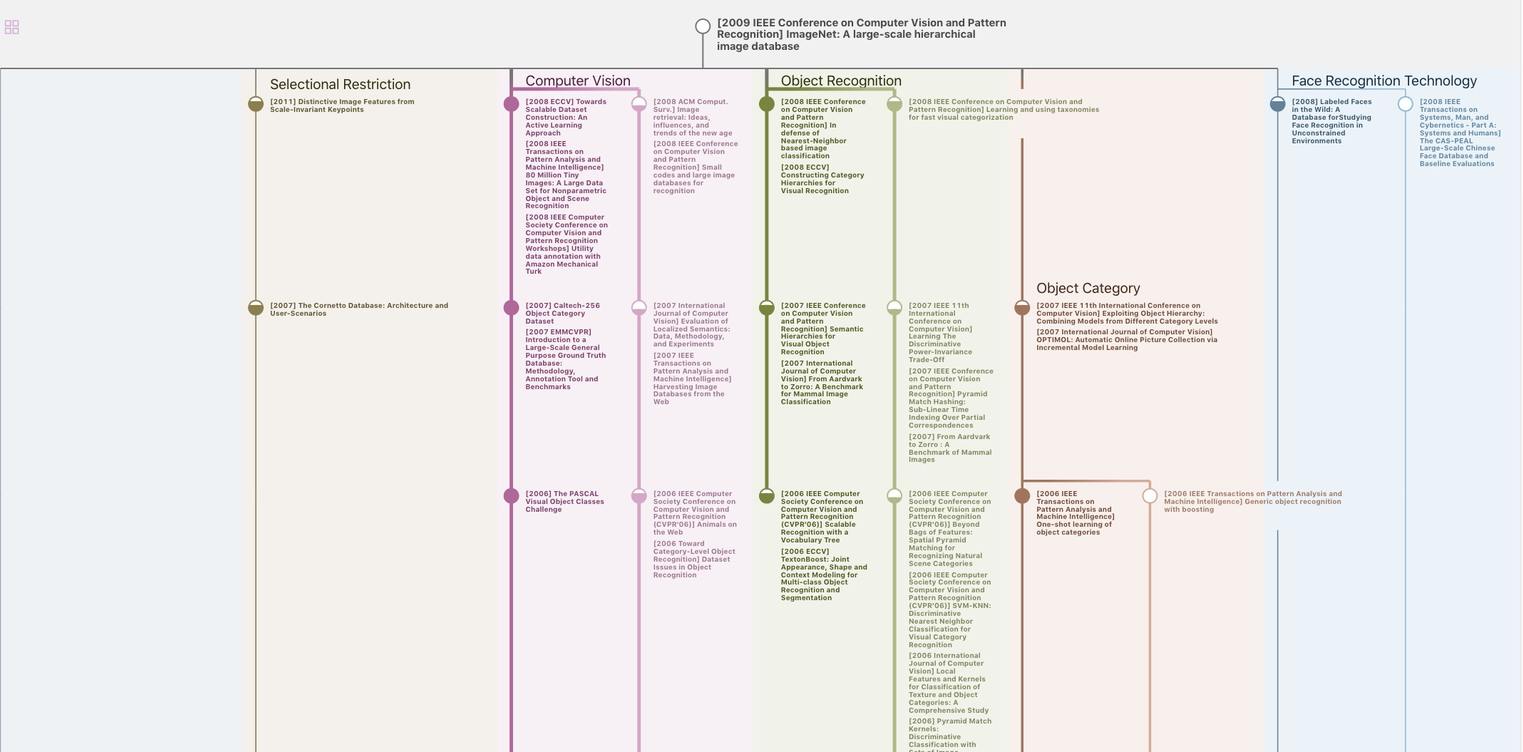
生成溯源树,研究论文发展脉络
Chat Paper
正在生成论文摘要