Differentially Private Decoding in Large Language Models
arxiv(2022)
摘要
Recent large-scale natural language processing (NLP) systems use a pre-trained Large Language Model (LLM) on massive and diverse corpora as a headstart. In practice, the pre-trained model is adapted to a wide array of tasks via fine-tuning on task-specific datasets. LLMs, while effective, have been shown to memorize instances of training data thereby potentially revealing private information processed during pre-training. The potential leakage might further propagate to the downstream tasks for which LLMs are fine-tuned. On the other hand, privacy-preserving algorithms usually involve retraining from scratch, which is prohibitively expensive for LLMs. In this work, we propose a simple, easy to interpret, and computationally lightweight perturbation mechanism to be applied to an already trained model at the decoding stage. Our perturbation mechanism is model-agnostic and can be used in conjunction with any LLM. We provide theoretical analysis showing that the proposed mechanism is differentially private, and experimental results showing a privacy-utility trade-off.
更多查看译文
关键词
private decoding,large language models
AI 理解论文
溯源树
样例
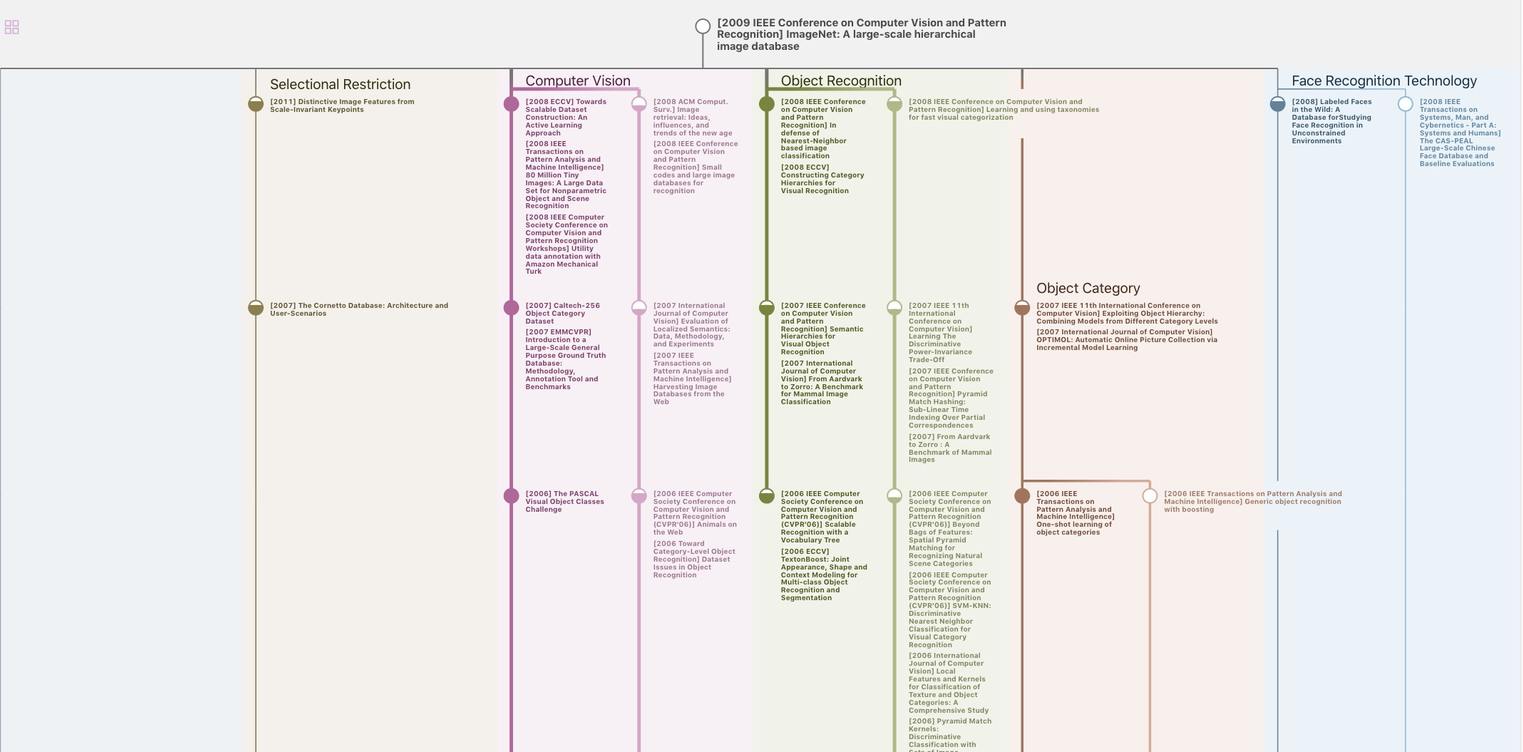
生成溯源树,研究论文发展脉络
Chat Paper
正在生成论文摘要