Pruning has a disparate impact on model accuracy
NeurIPS 2022(2022)
摘要
Network pruning is a widely-used compression technique that is able to significantly scale down overparameterized models with minimal loss of accuracy. This paper shows that pruning may create or exacerbate disparate impacts. The paper sheds light on the factors to cause such disparities, suggesting differences in gradient norms and distance to decision boundary across groups to be responsible for this critical issue. It analyzes these factors in detail, providing both theoretical and empirical support, and proposes a simple, yet effective, solution that mitigates the disparate impacts caused by pruning.
更多查看译文
关键词
Model pruning,Fairness
AI 理解论文
溯源树
样例
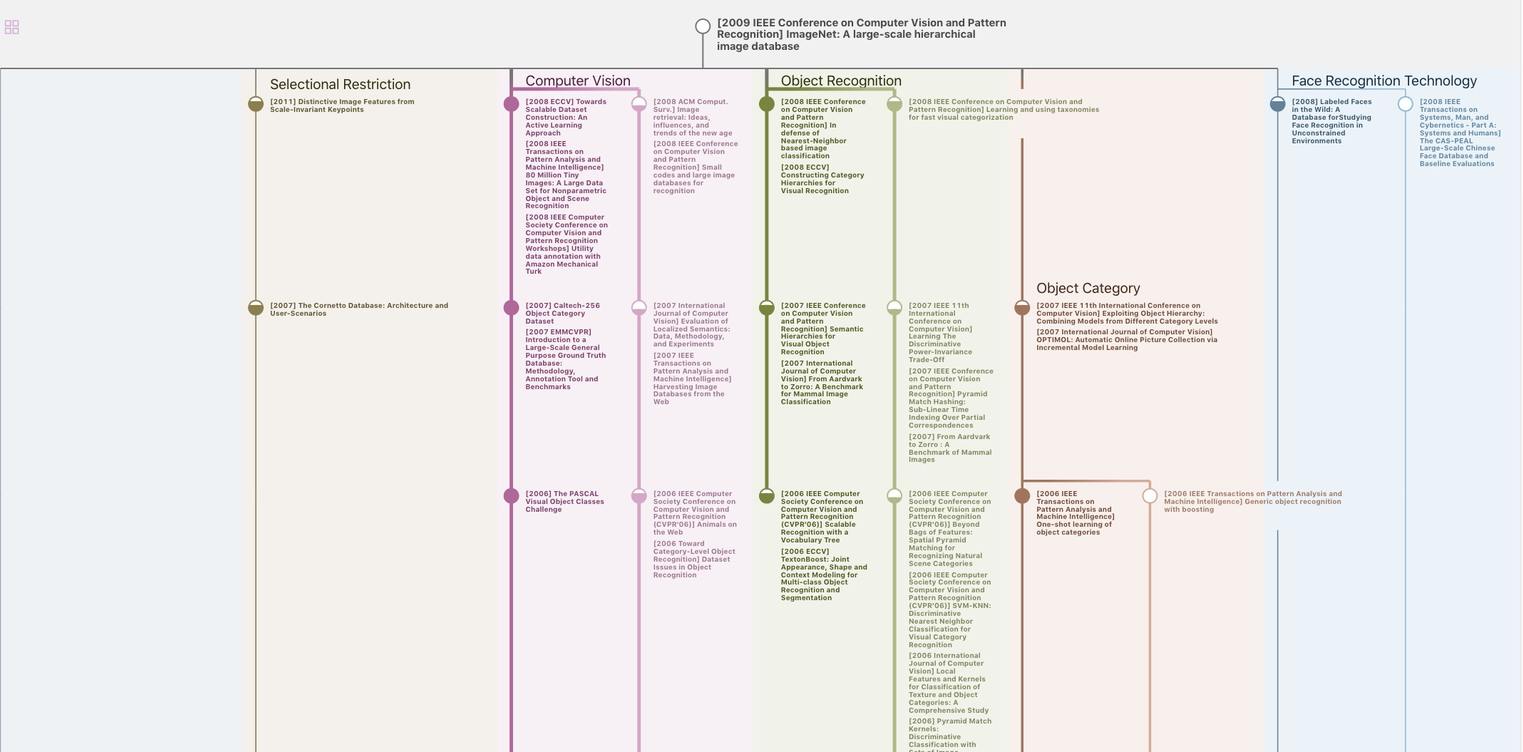
生成溯源树,研究论文发展脉络
Chat Paper
正在生成论文摘要