Attack-words Guided Sentence Generation for Textual Adversarial Attack
2021 IEEE Sixth International Conference on Data Science in Cyberspace (DSC)(2021)
摘要
Deep neural networks are vulnerable to carefully crafted adversarial examples and many adversarial attack methods have been proposed in computer vision tasks, such as image classification, object detection, etc. Generating adversarial examples for textual tasks is more challenging since the lexical correctness, grammatical correctness and semantics similarity should be maintained. In this paper, we introduce an attack-words guided sentence generation (AGSG) method to attack text classification models. We first determine words' attack ability by the ensemble strategy, then we add perturbation by inserting a short attack sentence. We conduct extensive experiments on two popular datasets IMDB and Amazon Comments against TextCNN, LSTM and RCNN models. The results show that the AGSG method greatly reduces the classification accuracy with a low word substitution rate. Specifically, the accuracy is reduced by 94.5% and 90.1% when disturbance rate is 13.3% and 25.1% for IMDB and Amazon Comments respectively. The similarity evaluation study shows that our adversarial attack method guarantees semantic similarity and grammatical correctness. Compared with two baseline adversarial attack methods, the AGSG method can generate adversarial texts that are harder for humans to perceive.
更多查看译文
关键词
Adversarial examples,text categorization,sentence generation,deep learning
AI 理解论文
溯源树
样例
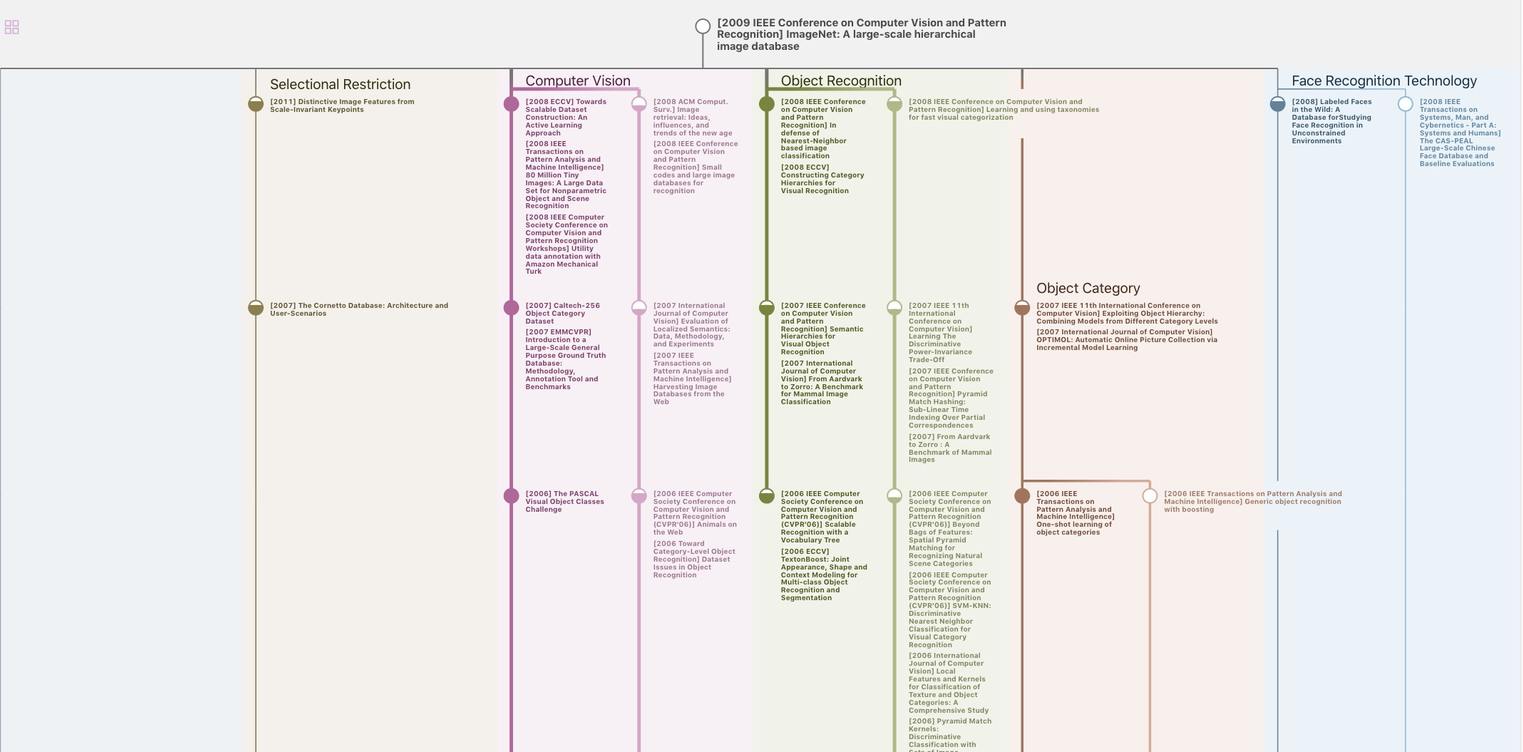
生成溯源树,研究论文发展脉络
Chat Paper
正在生成论文摘要