Precipitable water vapor fusion method based on artificial neural network
Advances in Space Research(2022)
摘要
Precipitable water vapor (PWV) can be retrieved using various techniques, such as global navigation satellite system (GNSS), remote sensing, and numerical prediction model. However, the existing techniques cannot simultaneously obtain water vapor information with high precision and high spatial and temporal resolutions. This study proposes a multisource PWV fusion method based on back propagation artificial neutral network (BP-ANN) called BP-PF model. The global pressure and temperature 3 (GPT3) model is introduced to calculate the initial PWV value, and BP-ANN is applied to establish the model of the PWV difference derived from the GPT3 model and ERA-Interim/GNSS/radiosonde. The key parameters of BP-ANN, namely, learning rate, number of hidden layers, and the number of neurons in each layer, are determined through empirical and experimental methods, and the proposed BP-PF model is validated using the data of four seasons of the Loess Plateau (LP) area in 2014. Results reveal that (1) the proposed model has a good internal accuracy, with respective average root mean squares (RMSs) of 1.31, 1.95, 1.27, and 0.54 mm and biases of 0.02, −0.10, −0.01, 0.01 mm in the spring, summer, autumn and winter, respectively; (2) the external validations in specific stations with long-term time series and in the entire LP verify the superiority of the proposed method, and the average RMS and bias are 1.45 and −0.23 mm, respectively; and (3) the proposed BP-PF model exhibits a good performance under rainy conditions in the four seasons, which verifies its potential application in extreme weather.
更多查看译文
关键词
Multisource data,PWV fusion,GPT3 model,BP-PF model
AI 理解论文
溯源树
样例
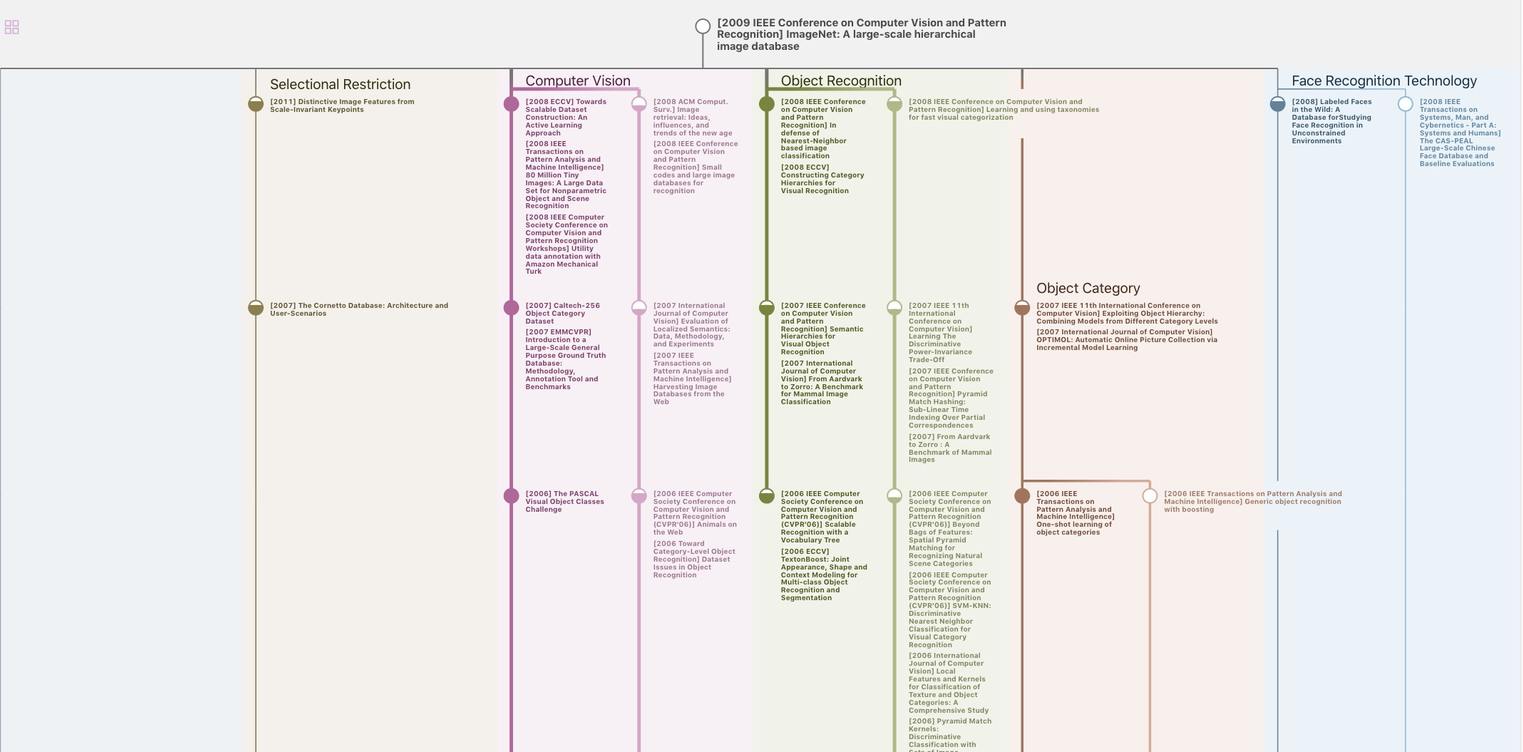
生成溯源树,研究论文发展脉络
Chat Paper
正在生成论文摘要