Deep Reinforcement Learning-based Edge Caching and Multi-link Cooperative Communication in Internet-of-Vehicles
2021 17th International Conference on Mobility, Sensing and Networking (MSN)(2021)
摘要
With the rapid development of 5G technologies, Internet-of-Vehicles (IoV) has become a promising and important research hotspot. The high-speed mobility of vehicles brings great challenges for services with low delay and high stability requirements. To address these challenges, this paper takes the relative movement between vehicles into account and analyzes the mobility in detail based on probability distribution. We propose a proactive caching and multi-link cooperative communication scheme to cope with mobility. According to the driving and content request information of vehicle users, the requested content is cached in the road side units (RSUs) and neighboring vehicles in advance. Furthermore, the optimal bandwidth is allocated for each communication link in order to improve the stability of vehicle communication and data transmission efficiency. We propose a Deep Reinforcement Learning-based Proactive Caching and Bandwidth Allocation Algorithm (DPCBA) by considering the high-dimensional continuity of the state and action space. The extensive simulation results demonstrate that our DPCBA scheme can effectively improve the Quality-of-Experience (QoE) of vehicle users in various situations, and outperforms traditional benchmark algorithms.
更多查看译文
关键词
Internet-of-Vehicles,Proactive Caching,Bandwidth Allocation,Cooperative Communication,Deep Reinforcement Learning.
AI 理解论文
溯源树
样例
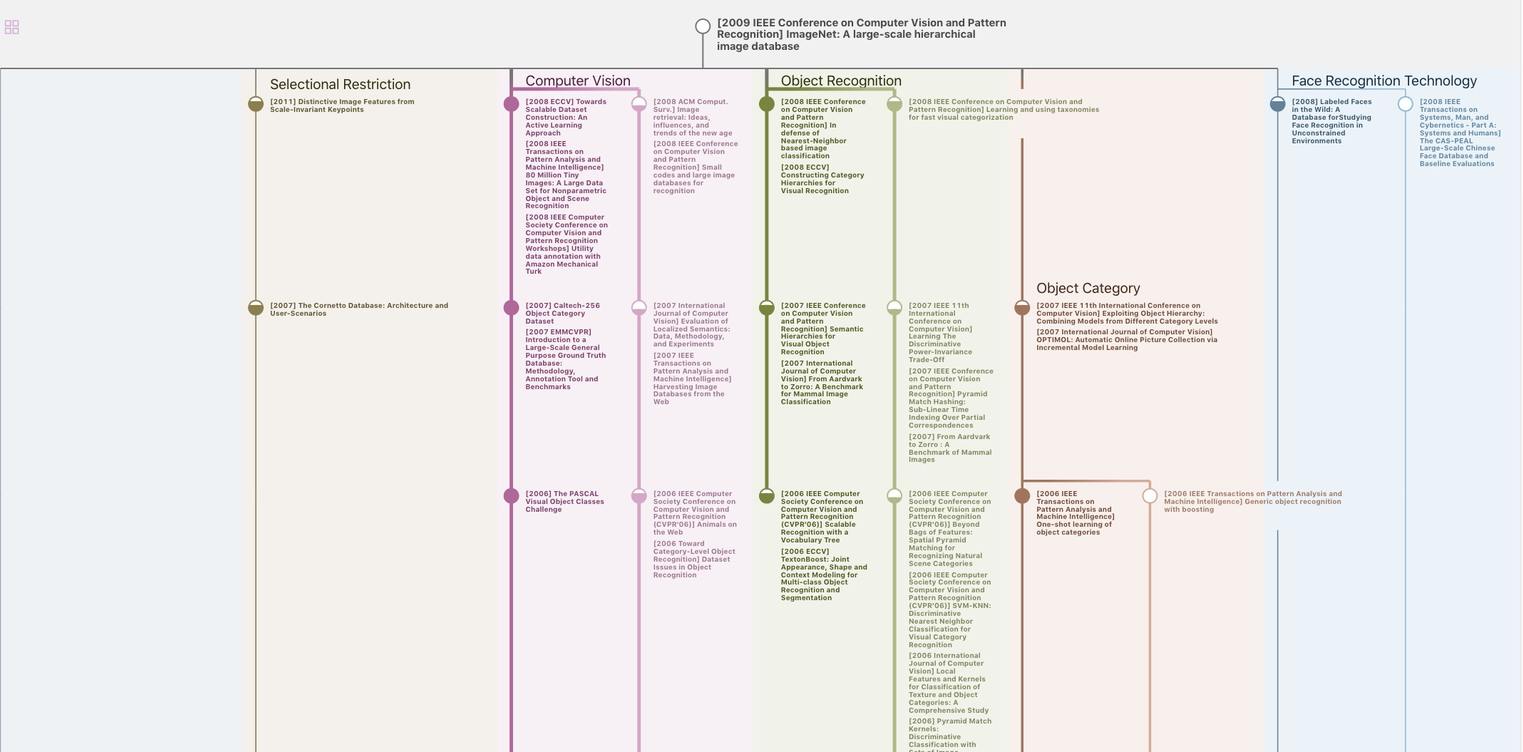
生成溯源树,研究论文发展脉络
Chat Paper
正在生成论文摘要