SIEV-Net: A Structure-Information Enhanced Voxel Network for 3D Object Detection From LiDAR Point Clouds
IEEE TRANSACTIONS ON GEOSCIENCE AND REMOTE SENSING(2022)
摘要
As one of the fundamental tasks in scene understanding, 3D object detection from LiDAR point clouds has drawn extensive attention in the past few years. Although the existing voxel-based methods have achieved remarkable performance, how to effectively exploit geometric structure information of the point clouds to boost the detection performance remains to be explored. In this article, we propose a novel structure-information enhanced voxel network (SIEV-Net) for 3D object detection from LiDAR point clouds. The proposed SIEV-Net learns feature representations of 3D objects by jointly considering uneven spatial distribution and height information of the point clouds. Specifically, considering the uneven spatial distribution characteristics of point clouds, a hierarchical-voxel feature encoding module is proposed to effectively extract features of voxels in both sparse and dense regions. Besides, by utilizing the bird's eye view (BEV) map of point clouds, a height information complement module is designed to minimize the height information lost in the process of point feature aggregation in a voxel network. Experimental results on the widely used KITTI benchmark dataset have demonstrated the efficacy of the proposed SIEV-Net.
更多查看译文
关键词
Three-dimensional displays, Point cloud compression, Feature extraction, Object detection, Proposals, Laser radar, Semantics, 3D object detection, LiDAR point clouds, scene understanding, structure information
AI 理解论文
溯源树
样例
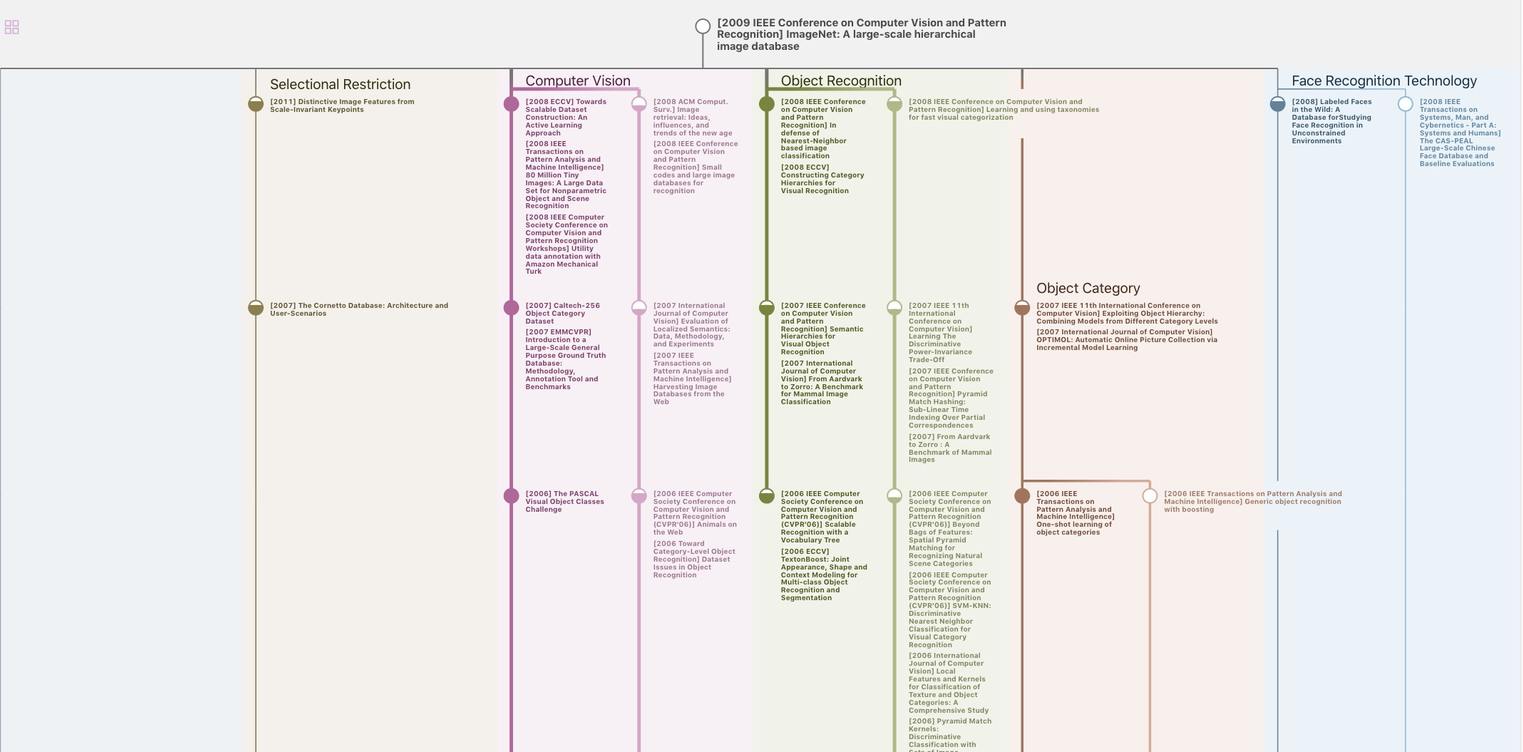
生成溯源树,研究论文发展脉络
Chat Paper
正在生成论文摘要