Classifying subtypes and predicting survival of renal cell carcinoma using histopathology image-based deep learning
user-61447a76e55422cecdaf7d19(2021)
摘要
Abstract Classifying histopathological subtypes and predicting survival of renal cell carcinoma (RCC) patients are critical steps towards treatment. In this work, we first proposed a deep learning method involving patch-based segmentation, intelligent feature extraction and heatmap visualization for classifying RCC into clear cell RCC, papillary RCC, chromophobe RCC, and adjacent benign tissue. This algorithm was trained and validated using 2,374,446 patches, 6,340 whole-slide images, 2,399 patients from The Cancer Genome Atlas and 6 independent centers. The classifiers provided areas under the curves of 0.979 to 0.996 in the internal phase, and 0.914 to 0.995 in the 6-center external phase. Furthermore, a modified deep learning approach comprising automated detection of regions of interest, patch-level learning, and morphological features-based risk scoring was developed for predicting survival of clear cell RCC patients. The prognostication model provided a hazard ratio for poor versus good prognosis of 2.63 [95% confidence interval (CI) 1.53–4.50, P = 4.35e-4] in the testing set, and 2.57 [95% CI 1.43–4.64, P = 1.68e-3] in an independent validation set using multivariable analyses. In conclusion, the developed histopathology image-based deep learning frameworks have the clinical potential to assist pathologists in systematically evaluating histological information of RCC patients.
更多查看译文
关键词
renal cell carcinoma,deep learning,image-based
AI 理解论文
溯源树
样例
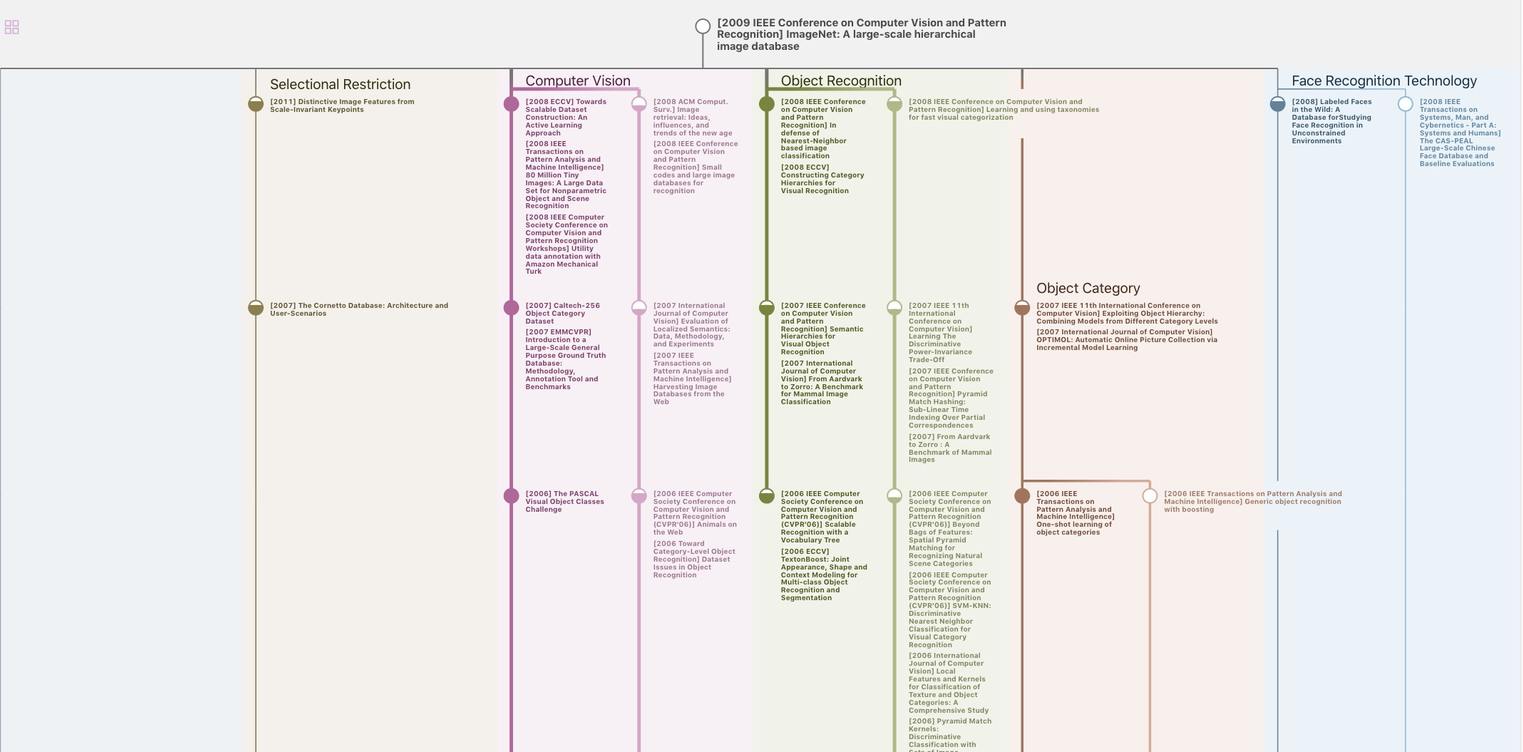
生成溯源树,研究论文发展脉络
Chat Paper
正在生成论文摘要