Multi-Stream Dense View Reconstruction Network for Light Field Image Compression.
user-61447a76e55422cecdaf7d19(2023)
摘要
Recently, many view synthesis-based methods are proposed for high-efficiency light field (LF) image compression. However, most existing methods fail to recover more texture details on occlusion regions, which reduces the compression efficiency. In this paper, we propose a multi-stream dense view reconstruction network to further improve LF image compression performance. In our method, only sparsely-sampled LF views are transmitted and the rest of the views are reconstructed at the decoder side. During the reconstruction process, we firstly constitute a multi-disparity geometry (MDG) structure based on the decoded sparse LF views, which can reflect abundant disparity characteristics. Subsequently, a multi-stream view reconstruction network (MSVRNet) is put forward to reconstruct a high-quality dense LF image, which consists of a multi-scale feature fusion sub-network, a fusion reconstruction sub-network, and a detail refinement sub-network. The multi-scale feature fusion sub-network can implicitly lean abundant multiscale geometric structure features from the constituted MDG structure. The fusion reconstruction sub-network and the detail refinement sub-network are respectively utilized to fuse the learned multiscale geometric features and restore more texture details, especially for occlusion regions. Moreover, 3D convolutional operations are adopted in the whole reconstruction process, which allow information propagation among the learned multiscale geometric features. Comprehensive experimental results demonstrate the effectiveness of the proposed method. The perceptual quality of reconstructed views and application on depth estimation also demonstrate that the proposed method can keep structural consistency of the reconstructed LF image and recover more texture details.
更多查看译文
关键词
Deep learning,dense view reconstruction,light field image compression,multi-stream network
AI 理解论文
溯源树
样例
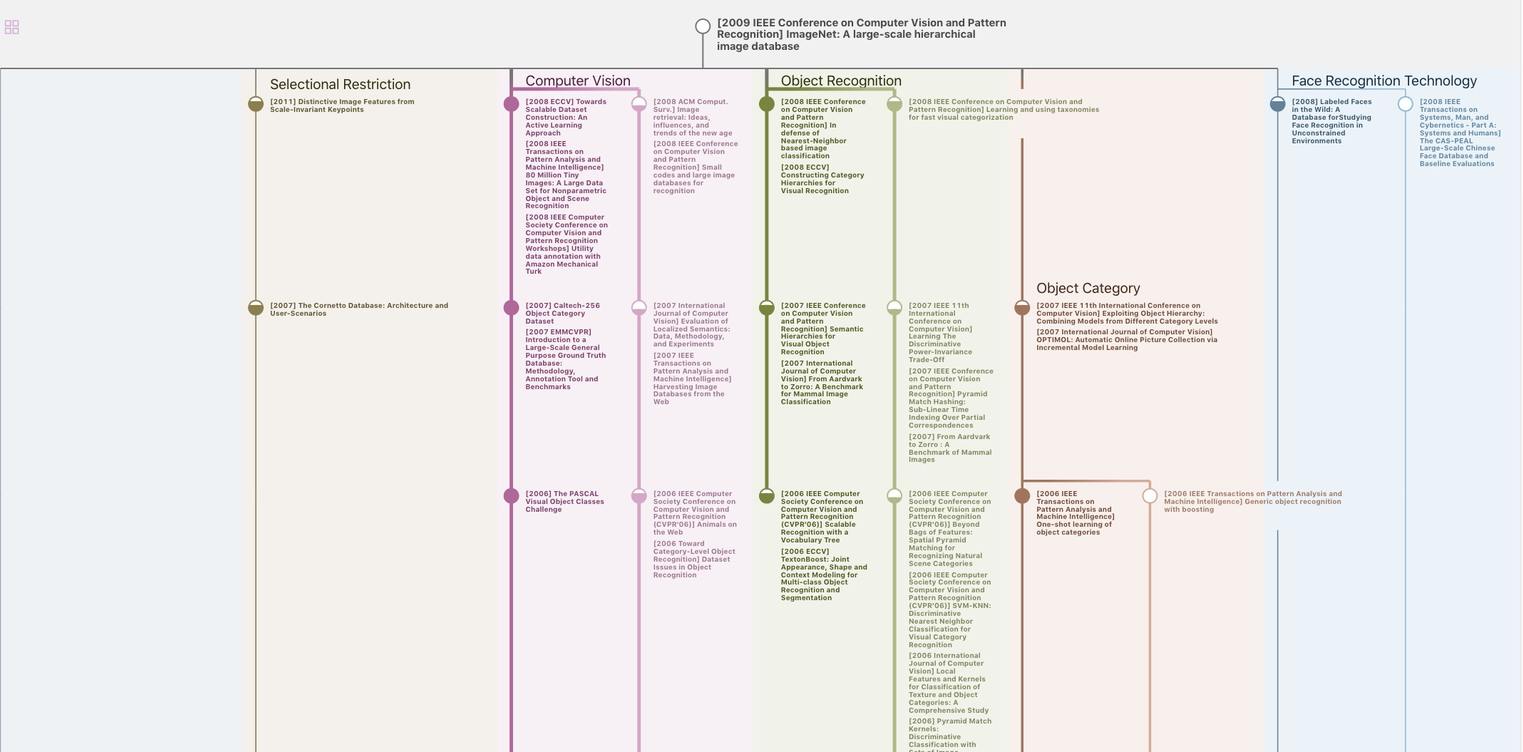
生成溯源树,研究论文发展脉络
Chat Paper
正在生成论文摘要