Incorporating EEG and EMG Patterns to Evaluate BCI-Based Long-Term Motor Training
IEEE Transactions on Human-Machine Systems(2022)
Abstract
Brain-computer interfaces (BCIs) provide users with a direct communication pathway between the brain and the peripheral environment. BCI-controlled devices have the potential to assist disabled patients in regaining motor functions. However, it remains unclear what happens to the functional coupling between the brain and muscle after BCI-based long-term motor training. Therefore, we developed a neurofeedback training method for long-term motor training that combines visual scenes and electrical stimulation. During the experiment, we collected electroencephalography (EEG) and electromyography (EMG) data from 20 subjects to explore their neurophysiological responses and the EEG-EMG coupling relationship. Event-related desynchronization (ERD), root mean square (rms) analysis, transfer entropy (TE) patterns, and other techniques were used to evaluate the cortical muscle response. Compared with the initial states, the ERD and rms significantly improved after long-term motor training. However, there was no significant difference in BCI performance. Directional TE values revealed the cortical muscle mechanism. These results demonstrate that incorporating EEG and EMG patterns to evaluate and establish a BCI-based motor training method is feasible. Furthermore, this article could provide evidence for functional coupling mechanisms for cortical muscles and motor rehabilitation.
MoreTranslated text
Key words
Brain-computer interface,electrical stimulation,electroencephalography and electromyography,event-related desynchronization,motor training,transfer entropy
AI Read Science
Must-Reading Tree
Example
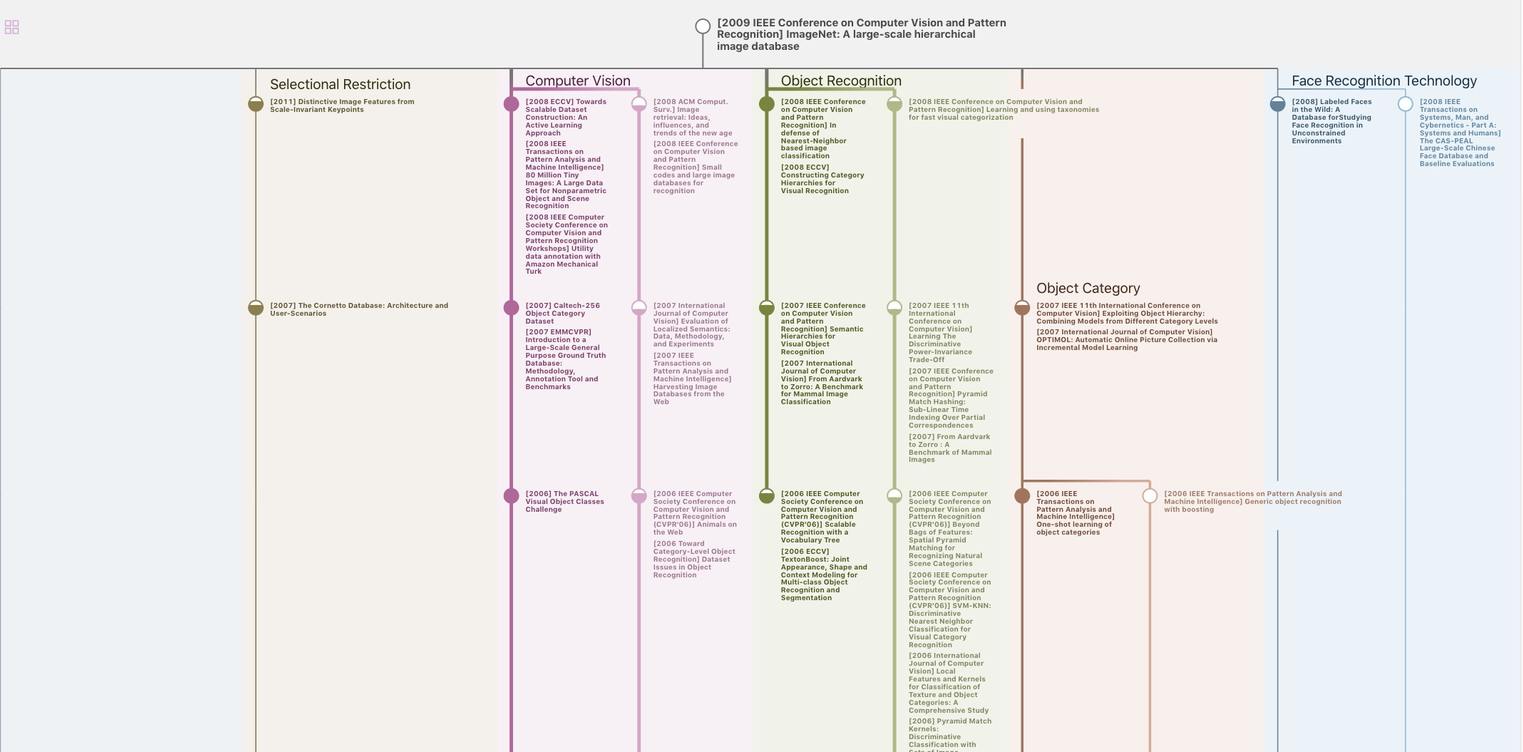
Generate MRT to find the research sequence of this paper
Chat Paper
Summary is being generated by the instructions you defined