Constrained Reinforcement Learning for Short Video Recommendation
arxiv(2022)
摘要
The wide popularity of short videos on social media poses new opportunities and challenges to optimize recommender systems on the video-sharing platforms. Users provide complex and multi-faceted responses towards recommendations, including watch time and various types of interactions with videos. As a result, established recommendation algorithms that concern a single objective are not adequate to meet this new demand of optimizing comprehensive user experiences. In this paper, we formulate the problem of short video recommendation as a constrained Markov Decision Process (MDP), where platforms want to optimize the main goal of user watch time in long term, with the constraint of accommodating the auxiliary responses of user interactions such as sharing/downloading videos. To solve the constrained MDP, we propose a two-stage reinforcement learning approach based on actor-critic framework. At stage one, we learn individual policies to optimize each auxiliary response. At stage two, we learn a policy to (i) optimize the main response and (ii) stay close to policies learned at the first stage, which effectively guarantees the performance of this main policy on the auxiliaries. Through extensive simulations, we demonstrate effectiveness of our approach over alternatives in both optimizing the main goal as well as balancing the others. We further show the advantage of our approach in live experiments of short video recommendations, where it significantly outperforms other baselines in terms of watch time and interactions from video views. Our approach has been fully launched in the production system to optimize user experiences on the platform.
更多查看译文
关键词
constrained reinforcement learning,video
AI 理解论文
溯源树
样例
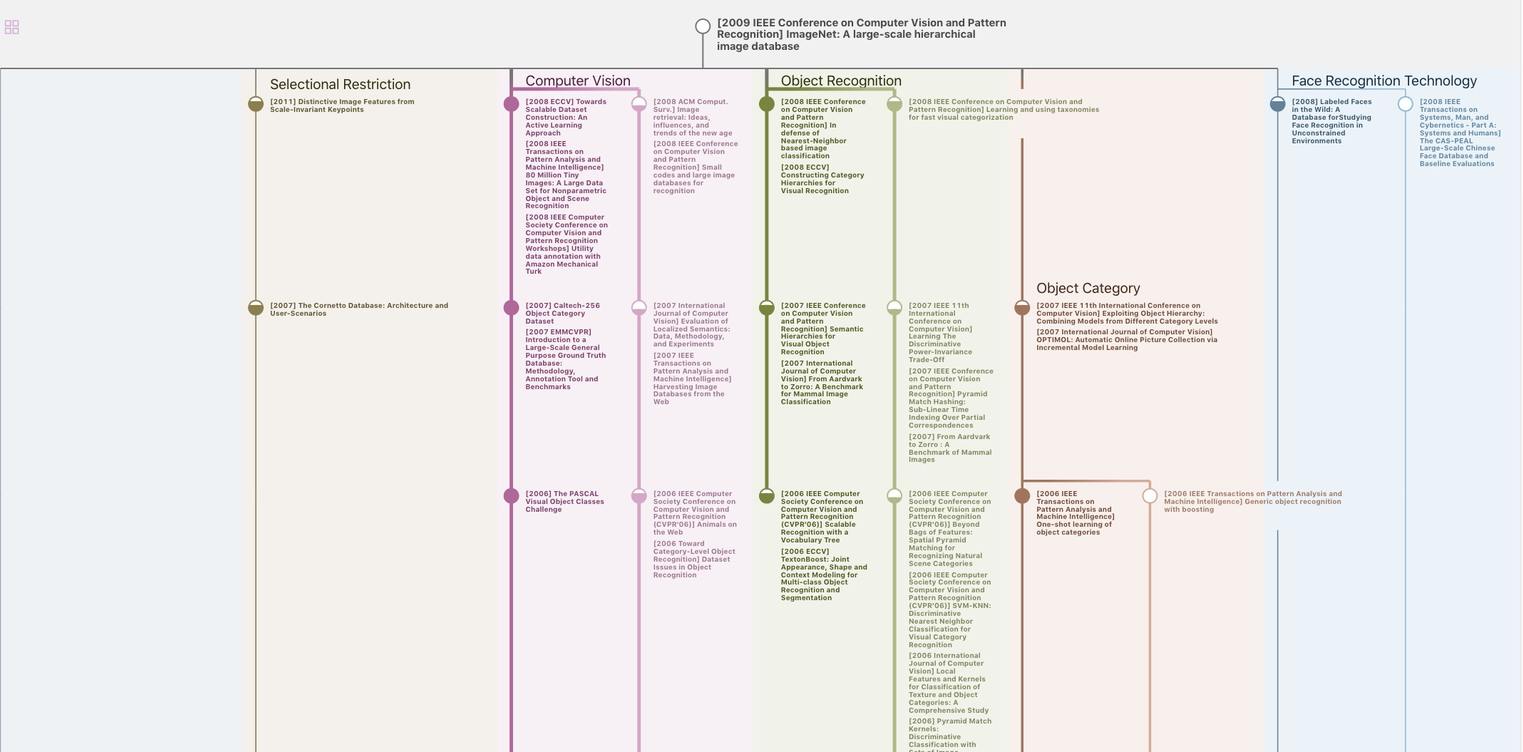
生成溯源树,研究论文发展脉络
Chat Paper
正在生成论文摘要