Learning to segment with limited annotations: Self-supervised pretraining with regression and contrastive loss in MRI
arxiv(2022)
摘要
Obtaining manual annotations for large datasets for supervised training of deep learning (DL) models is challenging. The availability of large unlabeled datasets compared to labeled ones motivate the use of self-supervised pretraining to initialize DL models for subsequent segmentation tasks. In this work, we consider two pre-training approaches for driving a DL model to learn different representations using: a) regression loss that exploits spatial dependencies within an image and b) contrastive loss that exploits semantic similarity between pairs of images. The effect of pretraining techniques is evaluated in two downstream segmentation applications using Magnetic Resonance (MR) images: a) liver segmentation in abdominal T2-weighted MR images and b) prostate segmentation in T2-weighted MR images of the prostate. We observed that DL models pretrained using self-supervision can be finetuned for comparable performance with fewer labeled datasets. Additionally, we also observed that initializing the DL model using contrastive loss based pretraining performed better than the regression loss.
更多查看译文
关键词
mri,contrastive loss,learning,limited annotations,self-supervised
AI 理解论文
溯源树
样例
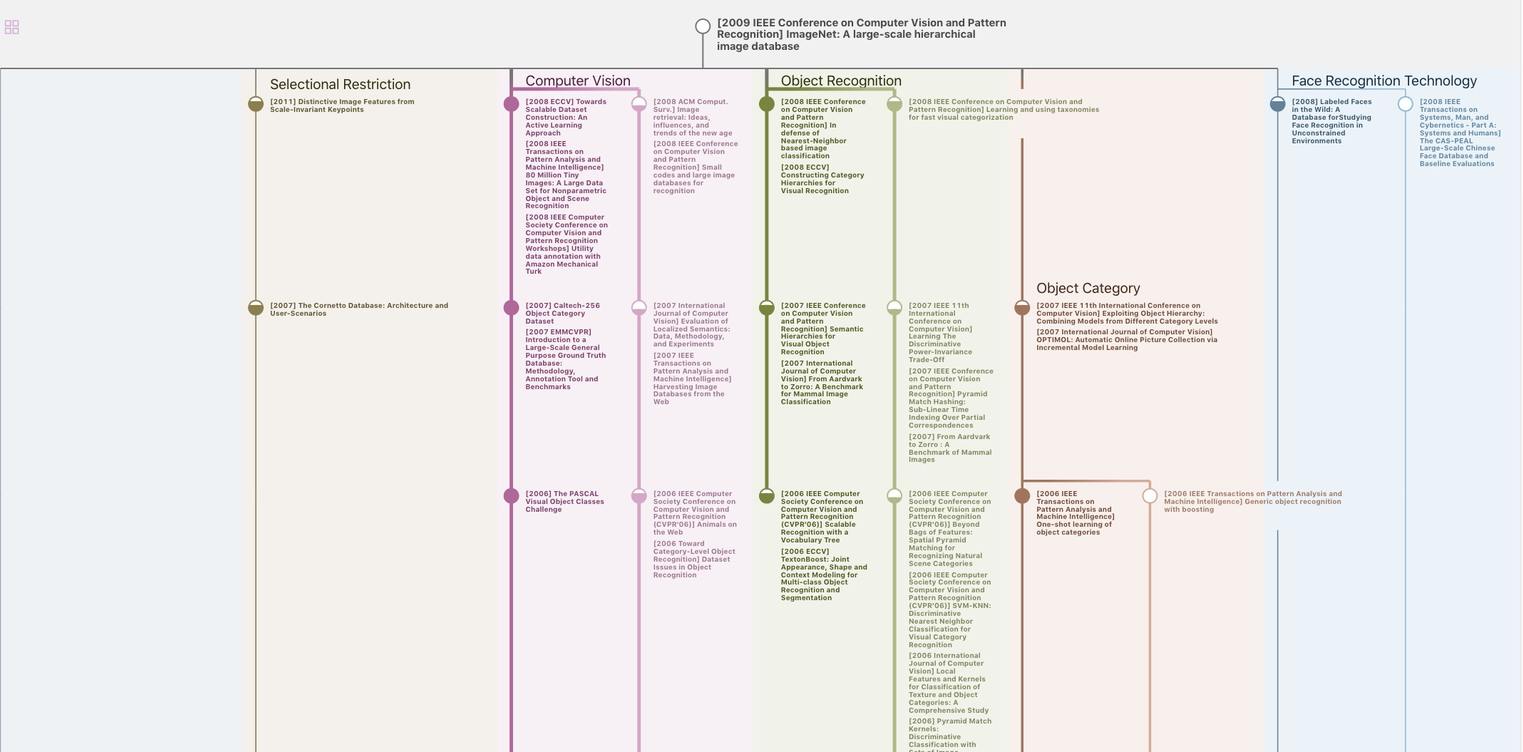
生成溯源树,研究论文发展脉络
Chat Paper
正在生成论文摘要