Identifying patient-specific root causes with the heteroscedastic noise model
arxiv(2023)
摘要
Complex diseases are caused by a multitude of factors that may differ between patients even within the same diagnostic category. A few underlying root causes may nevertheless initiate the development of disease within each patient. We therefore focus on identifying patient-specific root causes of disease, which we equate to the sample-specific predictivity of the exogenous error terms in a structural equation model. We generalize from the linear setting to the heteroscedastic noise model where Y = m(X) + E & sigma; (X) with non-linear functions m(X) and & sigma;(X) representing the conditional mean and mean absolute deviation, respectively. This model preserves identifiability but introduces non-trivial challenges that require a customized algorithm called Generalized Root Causal Inference (GRCI) to extract the error terms correctly. GRCI recovers patient-specific root causes more accurately than existing alternatives.
更多查看译文
关键词
Causal inference,Functional causal model,Heteroscedastic noise,Root cause
AI 理解论文
溯源树
样例
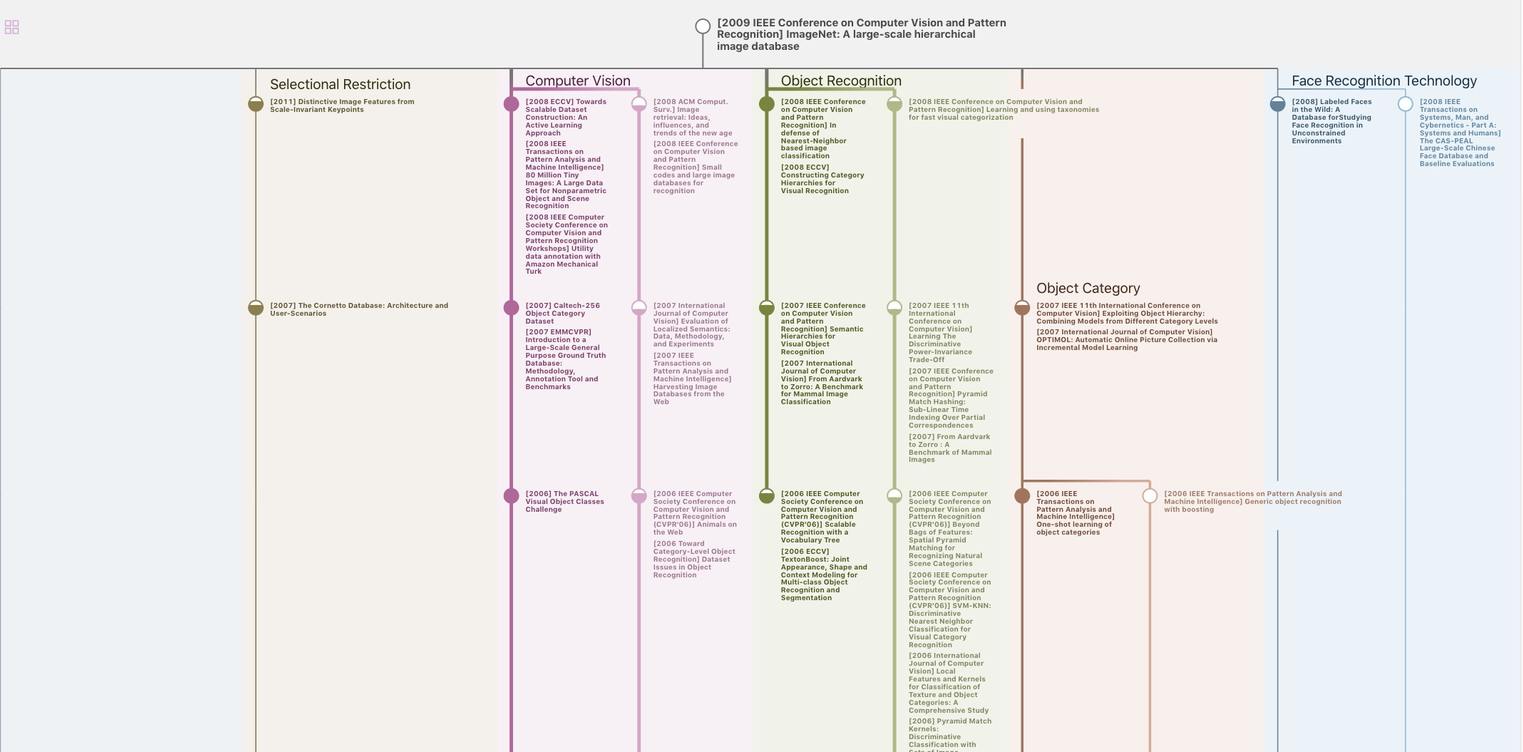
生成溯源树,研究论文发展脉络
Chat Paper
正在生成论文摘要