On Bridging the Gap between Mean Field and Finite Width in Deep Random Neural Networks with Batch Normalization
arxiv(2023)
摘要
Mean field theory is widely used in the theoretical studies of neural networks. In this paper, we analyze the role of depth in the concentration of mean-field predictions, specifically for deep multilayer perceptron (MLP) with batch normalization (BN) at initialization. By scaling the network width to infinity, it is postulated that the mean-field predictions suffer from layer-wise errors that amplify with depth. We demonstrate that BN stabilizes the distribution of representations that avoids the error propagation of mean-field predictions. This stabilization, which is characterized by a geometric mixing property, allows us to establish concentration bounds for mean field predictions in infinitely-deep neural networks with a finite width.
更多查看译文
关键词
batch normalization,deep random,neural networks,mean field
AI 理解论文
溯源树
样例
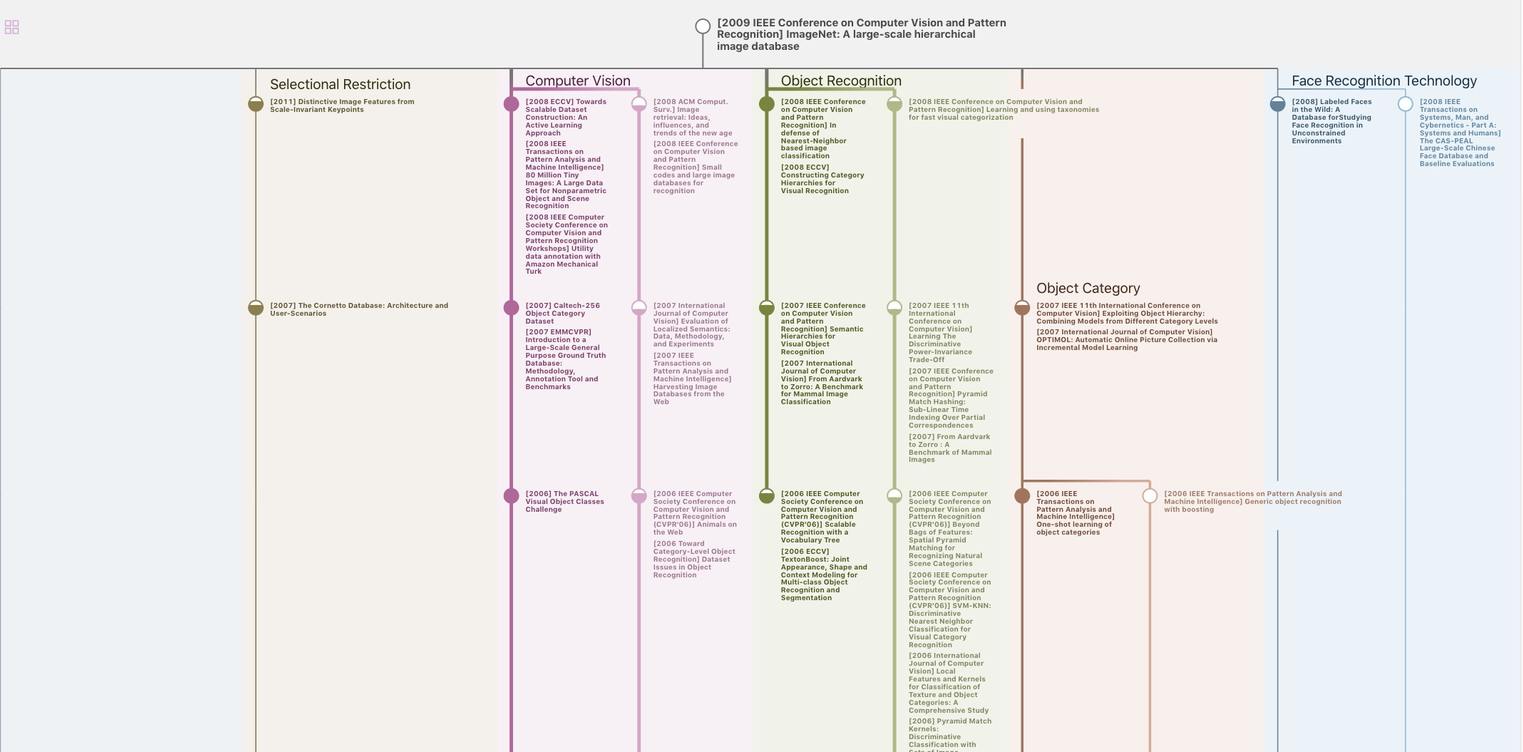
生成溯源树,研究论文发展脉络
Chat Paper
正在生成论文摘要