EvoVGM: A Deep Variational Generative Model for Evolutionary Parameter Estimation.
CoRR(2022)
摘要
BSTRACTMost evolutionary-oriented deep generative models do not explicitly consider the underlying evolutionary dynamics of biological sequences as it is performed within the Bayesian phylogenetic inference framework. In this study, we propose a method for a deep variational Bayesian generative model (EvoVGM) that jointly approximates the true posterior of local evolutionary parameters and generates sequence alignments. Moreover, it is instantiated and tuned for continuous-time Markov chain substitution models such as JC69, K80 and GTR. We train the model via a low-variance stochastic estimator and a gradient ascent algorithm. Here, we analyze the consistency and effectiveness of EvoVGM on synthetic sequence alignments simulated with several evolutionary scenarios and different sizes. Finally, we highlight the robustness of a fine-tuned EvoVGM model using a sequence alignment of gene S of coronaviruses.
更多查看译文
AI 理解论文
溯源树
样例
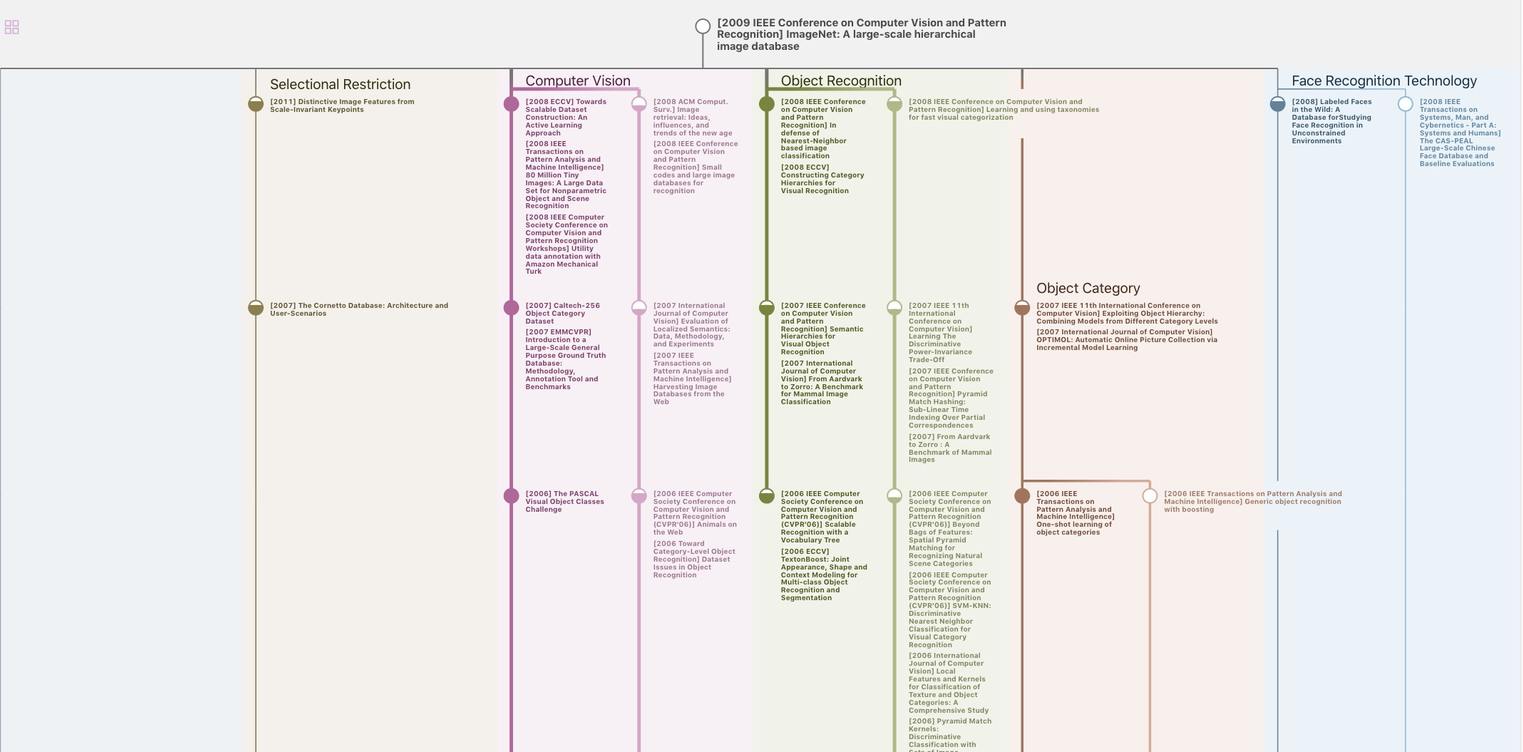
生成溯源树,研究论文发展脉络
Chat Paper
正在生成论文摘要