The Prediction of Fall Circumstances Among Patients in Clinical Care - A Retrospective Observational Study.
Medical Informatics Europe (MIE)(2022)
Abstract
Standardized fall risk scores have not proven to reliably predict falls in clinical settings. Machine Learning offers the potential to increase the accuracy of such predictions, possibly vastly improving care for patients at high fall risks. We developed a boosting algorithm to predict both recurrent falls and the severity of fall injuries. The model was trained on a dataset including extensive information on fall events of patients who had been admitted to Charité - Universitätsmedizin Berlin between August 2016 and July 2020. The data were recorded according to the German expert standard for fall documentation. Predictive power scores were calculated to define optimal feature sets. With an accuracy of 74% for recurrent falls and 86% for injury severity, boosting demonstrated the best overall predictive performance of all models assessed. Given that our data contain initially rated risk scores, our results demonstrate that well trained ML algorithms possibly provide tools to substantially reduce fall risks in clinical care settings.
MoreTranslated text
Key words
clinical care,fall prediction,machine learning,retrospective study
AI Read Science
Must-Reading Tree
Example
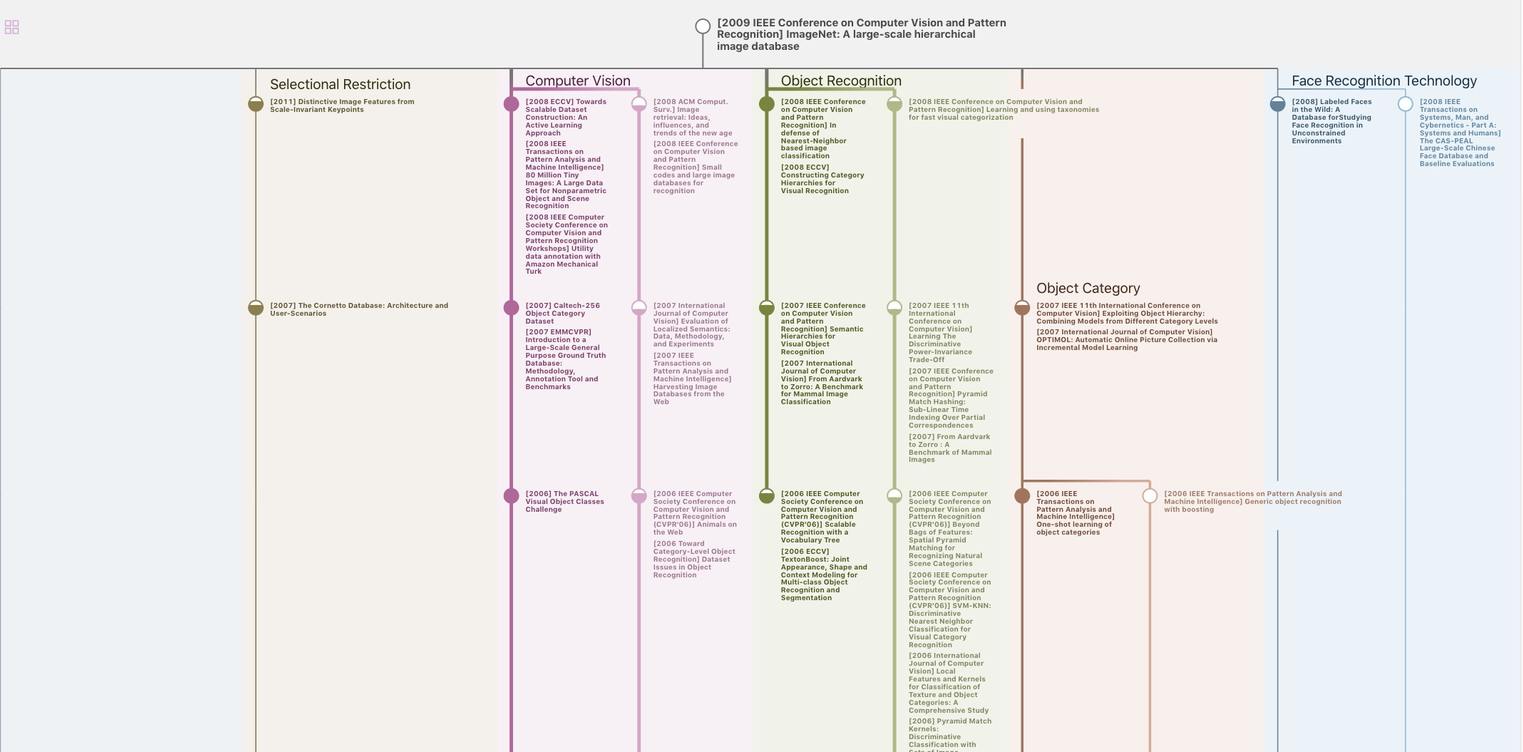
Generate MRT to find the research sequence of this paper
Chat Paper
Summary is being generated by the instructions you defined