Bayesian network-based over-sampling method (BOSME) with application to indirect cost-sensitive learning
SCIENTIFIC REPORTS(2022)
摘要
Traditional supervised learning algorithms do not satisfactorily solve the classification problem on imbalanced data sets, since they tend to assign the majority class, to the detriment of the minority class classification. In this paper, we introduce the Bayesian network-based over-sampling method (BOSME), which is a new over-sampling methodology based on Bayesian networks. Over-sampling methods handle imbalanced data by generating synthetic minority instances, with the benefit that classifiers learned from a more balanced data set have a better ability to predict the minority class. What makes BOSME different is that it relies on a new approach, generating artificial instances of the minority class following the probability distribution of a Bayesian network that is learned from the original minority classes by likelihood maximization. We compare BOSME with the benchmark synthetic minority over-sampling technique (SMOTE) through a series of experiments in the context of indirect cost-sensitive learning , with some state-of-the-art classifiers and various data sets, showing statistical evidence in favor of BOSME, with respect to the expected (misclassification) cost.
更多查看译文
关键词
Engineering,Mathematics and computing,Science,Humanities and Social Sciences,multidisciplinary
AI 理解论文
溯源树
样例
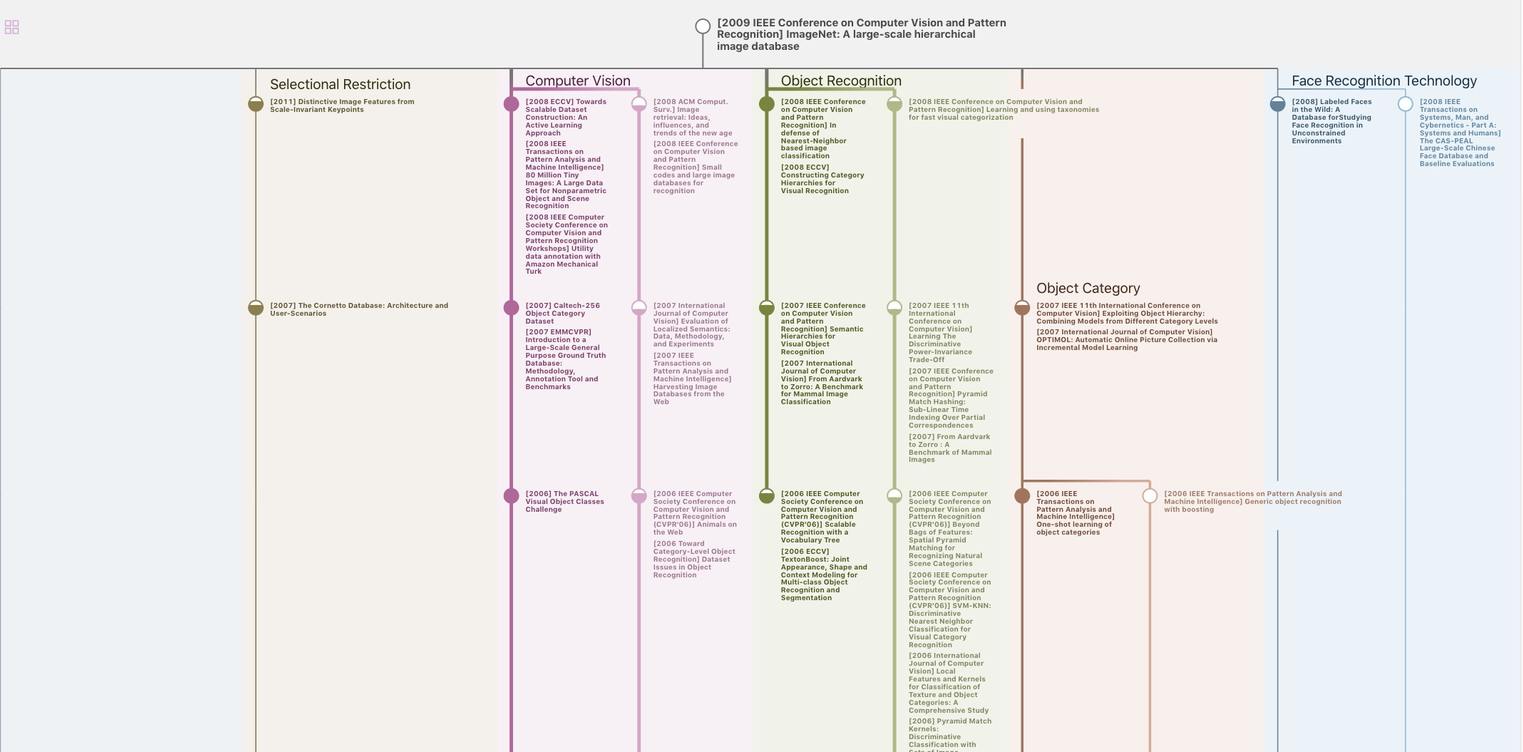
生成溯源树,研究论文发展脉络
Chat Paper
正在生成论文摘要