A systematic approach to Lyapunov analyses of continuous-time models in convex optimization
arXiv (Cornell University)(2022)
摘要
First-order methods are often analyzed via their continuous-time models,
where their worst-case convergence properties are usually approached via
Lyapunov functions. In this work, we provide a systematic and principled
approach to find and verify Lyapunov functions for classes of ordinary and
stochastic differential equations. More precisely, we extend the performance
estimation framework, originally proposed by Drori and Teboulle [10], to
continuous-time models. We retrieve convergence results comparable to those of
discrete methods using fewer assumptions and convexity inequalities, and
provide new results for stochastic accelerated gradient flows.
更多查看译文
关键词
lyapunov analyses,convex optimization,models,continuous-time
AI 理解论文
溯源树
样例
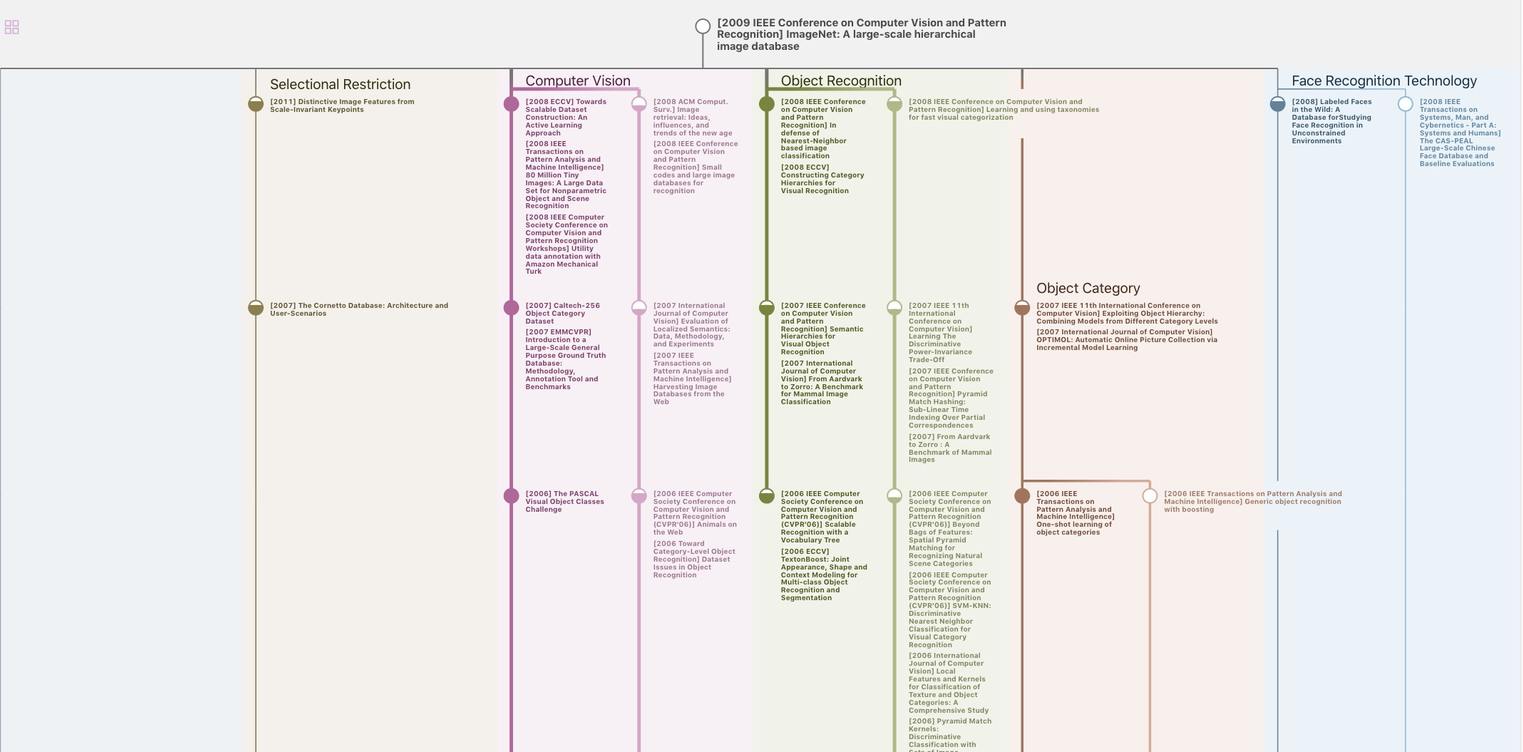
生成溯源树,研究论文发展脉络
Chat Paper
正在生成论文摘要