An Evolutionary Approach to Dynamic Introduction of Tasks in Large-scale Multitask Learning Systems
CoRR(2022)
摘要
Multitask learning assumes that models capable of learning from multiple tasks can achieve better quality and efficiency via knowledge transfer, a key feature of human learning. Though, state of the art ML models rely on high customization for each task and leverage size and data scale rather than scaling the number of tasks. Also, continual learning, that adds the temporal aspect to multitask, is often focused to the study of common pitfalls such as catastrophic forgetting instead of being studied at a large scale as a critical component to build the next generation artificial intelligence.We propose an evolutionary method capable of generating large scale multitask models that support the dynamic addition of new tasks. The generated multitask models are sparsely activated and integrates a task-based routing that guarantees bounded compute cost and fewer added parameters per task as the model expands.The proposed method relies on a knowledge compartmentalization technique to achieve immunity against catastrophic forgetting and other common pitfalls such as gradient interference and negative transfer. We demonstrate empirically that the proposed method can jointly solve and achieve competitive results on 69public image classification tasks, for example improving the state of the art on a competitive benchmark such as cifar10 by achieving a 15% relative error reduction compared to the best model trained on public data.
更多查看译文
关键词
tasks,dynamic introduction,evolutionary approach,learning,large-scale
AI 理解论文
溯源树
样例
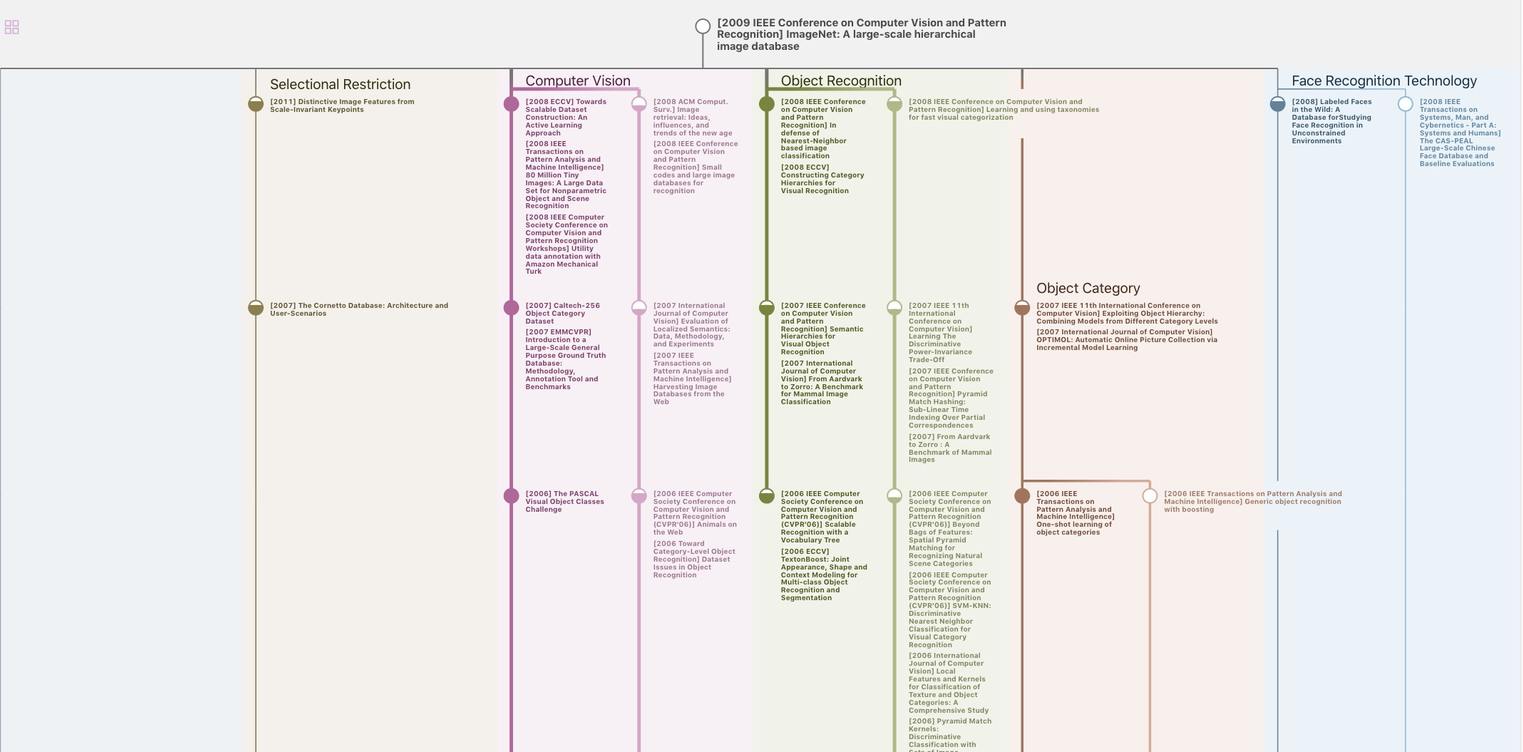
生成溯源树,研究论文发展脉络
Chat Paper
正在生成论文摘要