A Zipf's Law-Driven Method for Extracting Entities from Documents
CoRR(2023)
Abstract
Entity extraction is critical to the intelligent development of various domains and the construction of knowledge agents. Yet, there is category imbalance problem in documents in some specific domains that some categories of entities are common, while some are rare and scattered. This paper proposes to use Zipf's law to tackle this problem and to promote the performance of entity extraction from documents. Using two forms of Zipf's law, words in the documents are classified into common and rare ones, and then sentences are classified into common and rare ones, and are further processed by text generation models respectively. Rare entities in the generated sentences are labeled with human-designed rules, and serve as a supplement to the raw dataset so as to alleviate the category imbalance problem. A case of extracting entities from technical documents on industrial safety is given and the experiments results on two datasets show the effectiveness of the proposed method.
MoreTranslated text
AI Read Science
Must-Reading Tree
Example
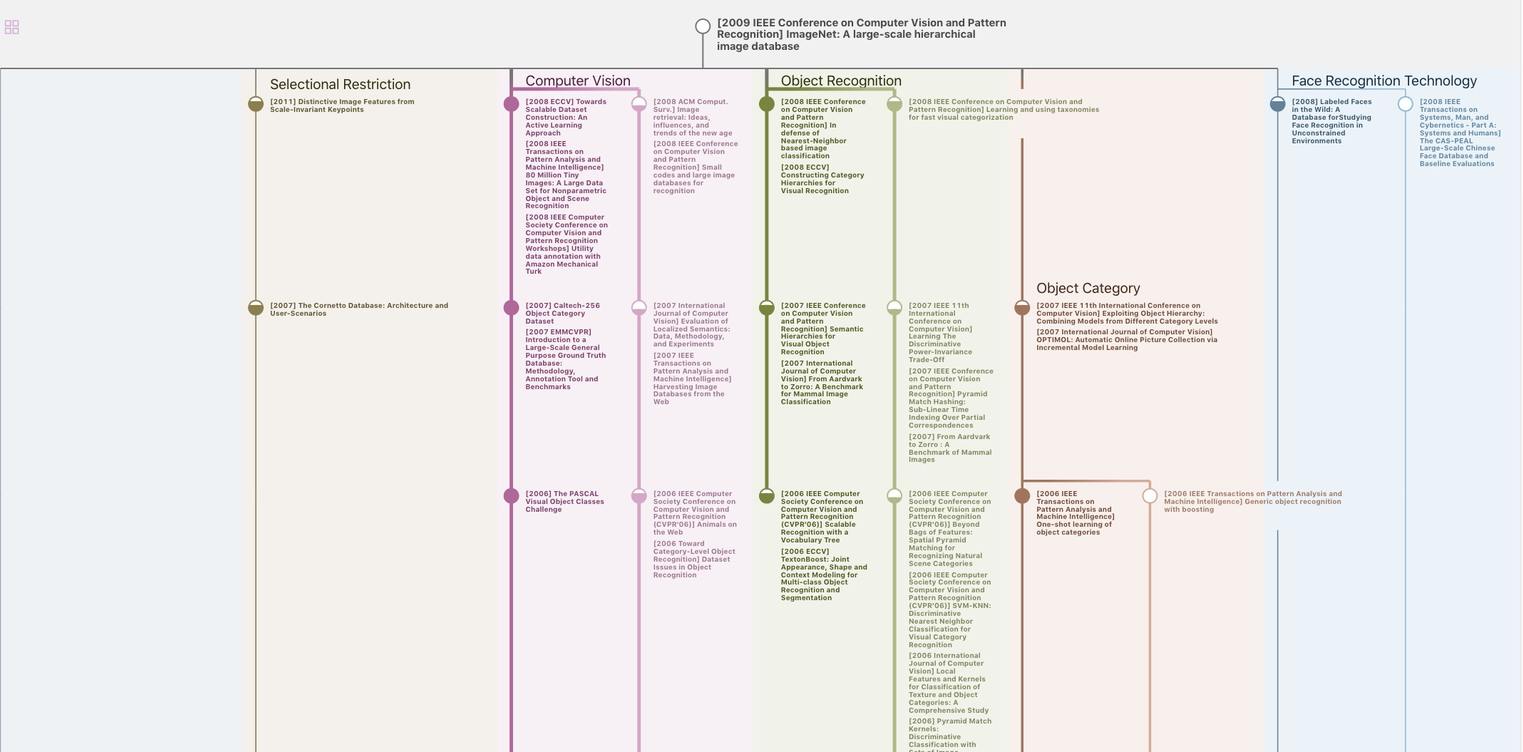
Generate MRT to find the research sequence of this paper
Chat Paper
Summary is being generated by the instructions you defined