Correction: Phased progressive learning with coupling-regulation-imbalance loss for imbalanced data classification
Neural Computing and Applications(2024)
摘要
Deep convolutional neural networks often perform poorly when faced with datasets that suffer from quantity imbalances and classification difficulties. Despite advances in the field, existing two-stage approaches still exhibit dataset bias or domain shift. To counter this, a phased progressive learning schedule has been proposed that gradually shifts the emphasis from representation learning to training the upper classifier. This approach is particularly beneficial for datasets with larger imbalances or fewer samples. Another new method a coupling-regulation-imbalance loss function is proposed, which combines three parts: a correction term, focal loss, and LDAM loss. This loss is effective in addressing quantity imbalances and outliers, while regulating the focus of attention on samples with varying classification difficulties. These approaches have yielded satisfactory results on several benchmark datasets, including Imbalanced CIFAR10, Imbalanced CIFAR100, ImageNet-LT, and iNaturalist 2018, and can be easily generalized to other imbalanced classification models. Deep convolutional neural networks often perform poorly when faced with datasets that suffer from quantity imbalances and classification difficulties. Despite advances in the field, existing two-stage approaches still exhibit dataset bias or domain shift. To counter this, a phased progressive learning schedule has been proposed that gradually shifts the emphasis from representation learning to training the upper classifier. This approach is particularly beneficial for datasets with larger imbalances or fewer samples. Another new method a coupling-regulation-imbalance loss function is proposed, which combines three parts: a correction term, focal loss, and LDAM loss. This loss is effective in addressing quantity imbalances and outliers, while regulating the focus of attention on samples with varying classification difficulties. These approaches have yielded satisfactory results on several benchmark datasets, including Imbalanced CIFAR10, Imbalanced CIFAR100, ImageNet-LT, and iNaturalist 2018, and can be easily generalized to other imbalanced classification models.
更多查看译文
关键词
Two-stage approaches,Representation learning,Classifier,Imbalanced classification
AI 理解论文
溯源树
样例
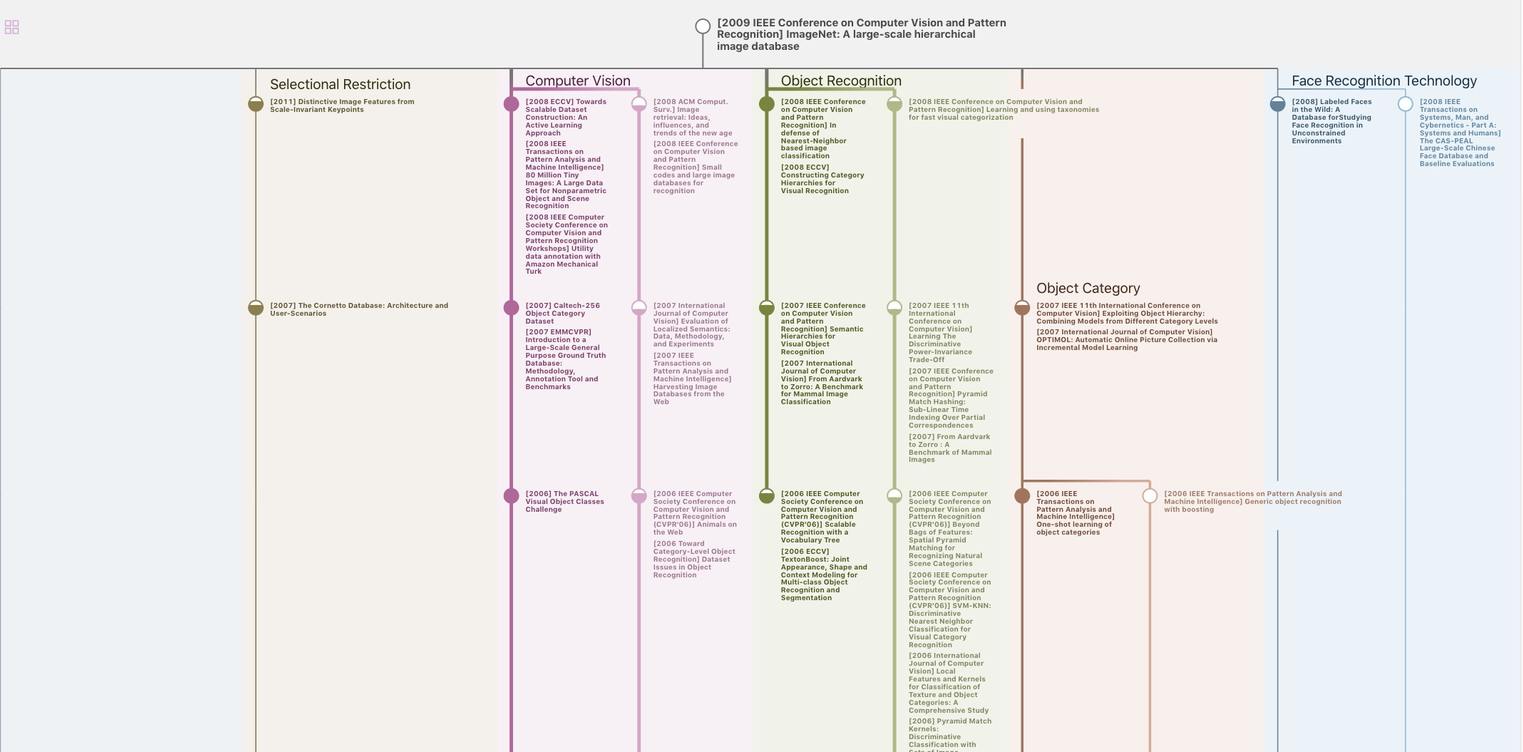
生成溯源树,研究论文发展脉络
Chat Paper
正在生成论文摘要