3D helical CT reconstruction with memory efficient invertible Learned Primal-Dual method
CoRR(2022)
摘要
Helical acquisition geometry is the most common geometry used in computed tomography (CT) scanners for medical imaging. We adapt the invertible Learned Primal-Dual (iLPD) deep neural network architecture so that it can be applied to helical 3D CT reconstruction. We achieve this by splitting the geometry and the data in parts that fit the memory and by splitting images into corresponding sub-volumes. The architecture can be applied to images different in size along the rotation axis. We perform the experiments on tomographic data simulated from realistic helical geometries.
更多查看译文
关键词
helical ct reconstruction,helical ct,primal-dual
AI 理解论文
溯源树
样例
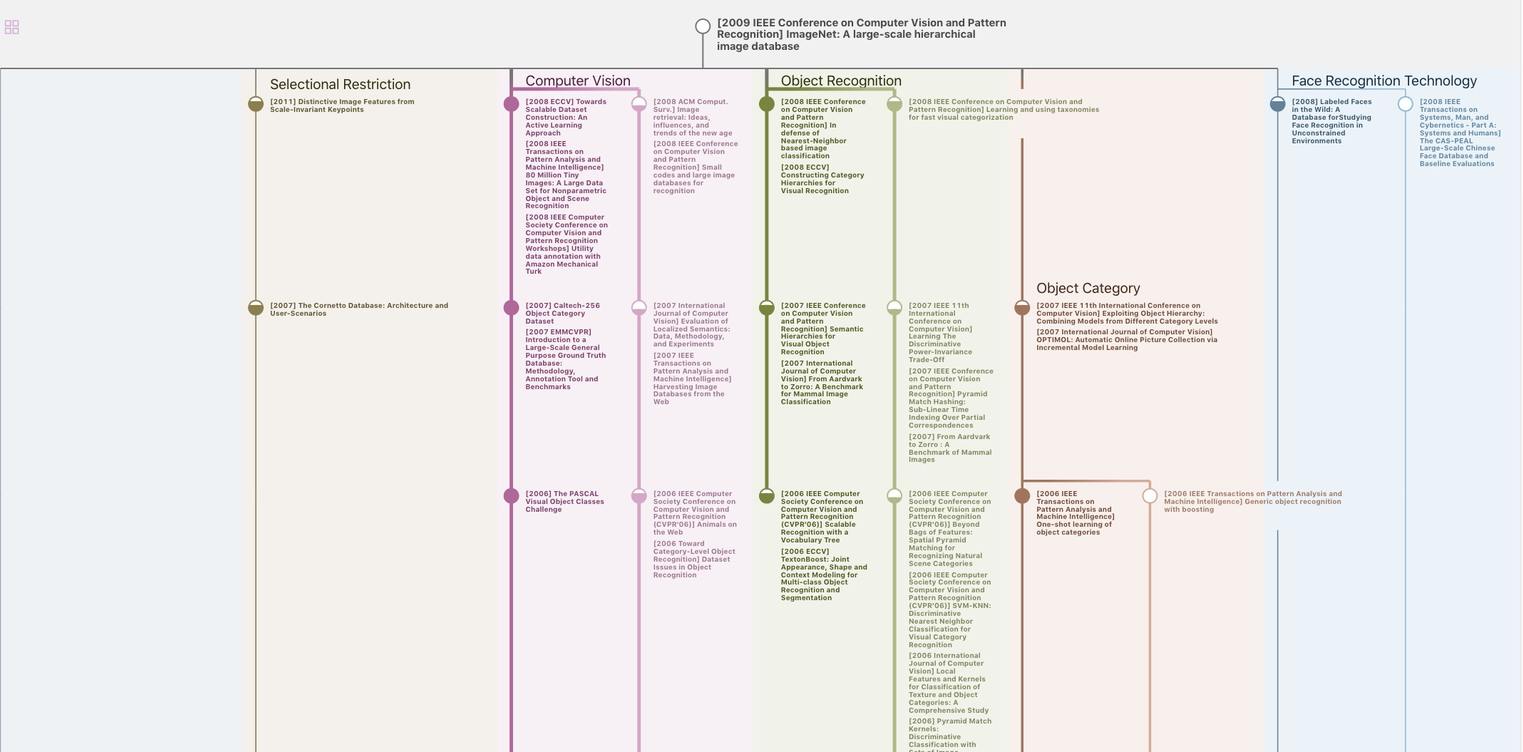
生成溯源树,研究论文发展脉络
Chat Paper
正在生成论文摘要