ItemSage: Learning Product Embeddings for Shopping Recommendations at Pinterest
KDD '22: Proceedings of the 28th ACM SIGKDD Conference on Knowledge Discovery and Data Mining(2022)
摘要
Learned embeddings for products are an important building block for web-scale e-commerce recommendation systems. At Pinterest, we build a single set of product embeddings called ItemSage to provide relevant recommendations in all shopping use cases including user, image and search based recommendations. This approach has led to significant improvements in engagement and conversion metrics, while reducing both infrastructure and maintenance cost. While most prior work focuses on building product embeddings from features coming from a single modality, we introduce a transformer-based architecture capable of aggregating information from both text and image modalities and show that it significantly outperforms single modality baselines. We also utilize multi-task learning to make ItemSage optimized for several engagement types, leading to a candidate generation system that is efficient for all of the engagement objectives of the end-to-end recommendation system. Extensive offline experiments are conducted to illustrate the effectiveness of our approach and results from online A/B experiments show substantial gains in key business metrics (up to +7% gross merchandise value/user and +11% click volume).
更多查看译文
关键词
learning product embeddings,shopping recommendations
AI 理解论文
溯源树
样例
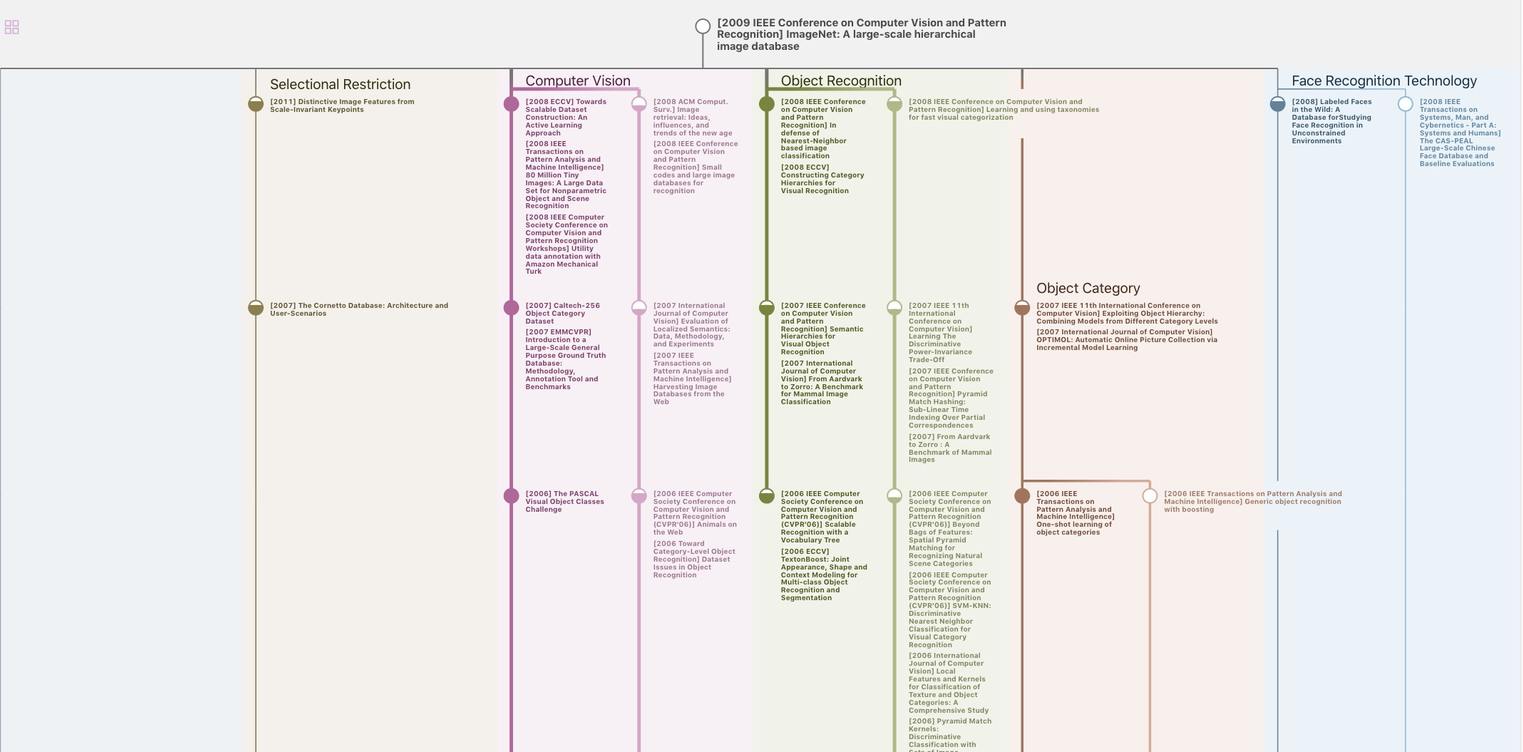
生成溯源树,研究论文发展脉络
Chat Paper
正在生成论文摘要