Data-Driven Machine Learning Model for Aircraft Icing Severity Evaluation
JOURNAL OF AEROSPACE INFORMATION SYSTEMS(2021)
摘要
No AccessTechnical NotesData-Driven Machine Learning Model for Aircraft Icing Severity EvaluationSibo Li, Jingkun Qin and Roberto PaoliSibo LiUniversity of Illinois at Chicago, Chicago, Illinois 60607*Ph.D. Candidate, Department of Mechanical and Industrial Engineering.Search for more papers by this author, Jingkun QinChina Literature Limited, 100105 Beijing, People’s Republic of China†Data Scientist.Search for more papers by this author and Roberto Paoli https://orcid.org/0000-0003-2158-8870University of Illinois at Chicago, Chicago, Illinois 60607‡Research Assistant Professor, Department of Mechanical and Industrial Engineering; also Leadership Computing Facility, Argonne National Laboratory, Lemont, Illinois 60439. Senior Member AIAA.Search for more papers by this authorPublished Online:14 Jul 2021https://doi.org/10.2514/1.I010978SectionsRead Now ToolsAdd to favoritesDownload citationTrack citations ShareShare onFacebookTwitterLinked InRedditEmail About References [1] Mclean J., “Determining the Effects of Weather in Aircraft Accident Investigations,” 24th Aerospace Sciences Meeting, AIAA Paper 1986-0323, 1986. https://doi.org/10.2514/6.1986-323 LinkGoogle Scholar[2] Bourgault Y., Boutanios Z. and Habashi W. G., “Three-Dimensional Eulerian Approach to Droplet Impingement Simulation Using FENSAP-ICE, Part 1: Model, Algorithm, and Validation,” Journal of Aircraft, Vol. 37, No. 1, 2000, pp. 95–103. https://doi.org/10.2514/2.2566 LinkGoogle Scholar[3] Cao Y., Tan W. and Wu Z., “Aircraft Icing: An Ongoing Threat to Aviation Safety,” Aerospace Science and Technology, Vol. 75, April 2018, pp. 353–385. https://doi.org/10.1016/j.ast.2017.12.028 CrossrefGoogle Scholar[4] Li S., Qin J., He M. and Paoli R., “Fast Evaluation of Aircraft Icing Severity Using Machine Learning Based on XGBoost,” Aerospace, Vol. 7, No. 4, 2020, pp. 36–54. https://doi.org/10.3390/aerospace7040036 CrossrefGoogle Scholar[5] Ogretim E., Huebsch W. and Shinn A., “Aircraft Ice Accretion Prediction Based on Neural Networks,” Journal of Aircraft, Vol. 43, No. 1, 2006, pp. 233–240. https://doi.org/10.2514/1.16241 LinkGoogle Scholar[6] McCann D. W., “NNICE—A Neural Network Aircraft Icing Algorithm,” Environmental Modelling and Software, Vol. 20, No. 10, 2005, pp. 1335–1342. https://doi.org/10.1016/j.envsoft.2004.09.027 CrossrefGoogle Scholar[7] Zhan Z., Habashi W. G. and Fossati M., “Local Reduced-Order Modeling and Iterative Sampling for Parametric Analyses of Aero-Icing Problems,” AIAA Journal, Vol. 53, No. 8, 2015, pp. 2174–2185. https://doi.org/10.2514/1.J053654 LinkGoogle Scholar[8] de Mello R. F. and Ponti M. A., “Statistical Learning Theory,” Machine Learning, Springer, New York, 2018. https://doi.org/10.1007/978-3-319-94989-5 CrossrefGoogle Scholar[9] Friedman J. H., “Greedy Function Approximation: A Gradient Boosting Machine,” Annals of Statistics, Vol. 29, No. 5, 2001, pp. 1189–1232. https://doi.org/10.1214/aos/1013203451 CrossrefGoogle Scholar[10] Chen T. and Guestrin C., “XGBoost: A Scalable Tree Boosting System,” Proceedings of the 22nd ACM SIGKDD International Conference on Knowledge Discovery and Data Mining, Assoc. for Computing Machinery, New York, 2016, pp. 785–794. https://doi.org/10.1145/2939672.2939785 Google Scholar[11] Darlington R. B. and Hayes A. F., “Multicategorical Regressors,” Regression Analysis and Linear Models: Concepts, Applications, and Implementation, Guilford Publ., New York, 2016, https://www.guilford.com/books/Regression-Analysis-and-Linear-Models/Darlington-Hayes/9781462521135. Google Scholar[12] Harrell F., “Ordinal Logistic Regression,” Regression Modeling Strategies, Springer Series in Statistics, Springer, New York, 2015, pp. 311–325. https://doi.org/10.1007/978-3-319-19425-7 CrossrefGoogle Scholar[13] Li S. and Paoli R., “Modeling of Ice Accretion over Aircraft Wings Using a Compressible OpenFOAM Solver,” International Journal of Aerospace Engineering, Vol. 2019, June 2019, p. 11. https://doi.org/10.1155/2019/4864927 CrossrefGoogle Scholar[14] Li S. and Paoli R., “Numerical Study of Ice Accretion over Aircraft Wings Using Delayed Detached Eddy Simulation,” Bulletin of the American Physical Soc., No. Q23, 2019, https://meetings.aps.org/Meeting/DFD19/Session/Q23.9. Google Scholar[15] Grajski K. A., Breiman L., Prisco G. V. D. and Freeman W. J., “Classification of EEG Spatial Patterns with a Tree-Structured Methodology: CART,” IEEE Transactions on Biomedical Engineering, Vol. BME-33, No. 12, 1986, pp. 1076–1086. https://doi.org/10.1109/TBME.1986.325684 CrossrefGoogle Scholar[16] Nwachukwu A., Jeong H., Pyrcz M. and Lake L. W., “Fast Evaluation of Well Placements in Heterogeneous Reservoir Models Using Machine Learning,” Journal of Petroleum Science and Engineering, Vol. 163, April 2018, pp. 463–475. https://doi.org/10.1016/j.petrol.2018.01.019 CrossrefGoogle Scholar[17] Heinrich A., Ross R., Zumwalt G., Provorse J. and Padmanabhan V., “Aircraft Icing Handbook,” Vols. 1–3, Federal Aviation Administration TR DOT/FAA/CT–88/8–1, 1993, http://www.tc.faa.gov/its/worldpac/techrpt/ct888-1.pdf. Google Scholar[18] “Part 25—Airworthiness Standards: Transport Category Airplanes,” Federal Aviation Administration, 2013, https://www.ecfr.gov/cgi-bin/text-idx?node=14:1.0.1.3.11. Google Scholar[19] Cao Y., Huang J. and Yin J., “Numerical Simulation of Three-Dimensional Ice Accretion on an Aircraft Wing,” International Journal of Heat and Mass Transfer, Vol. 92, Jan. 2016, pp. 34–54. https://doi.org/10.1016/j.ijheatmasstransfer.2015.08.027 CrossrefGoogle Scholar[20] Hedde T. and Guffond D., “ONERA Three-Dimensional Icing Model,” AIAA Journal, Vol. 33, No. 6, 1995, pp. 1038–1045. https://doi.org/10.2514/3.12795 LinkGoogle Scholar Previous article FiguresReferencesRelatedDetailsCited byComparison of Machine Learning Models for Data-Driven Aircraft Icing Severity EvaluationSibo Li and Roberto Paoli 15 November 2021 | Journal of Aerospace Information Systems, Vol. 18, No. 12 What's Popular Volume 18, Number 11November 2021 Metrics CrossmarkInformationCopyright © 2021 by the American Institute of Aeronautics and Astronautics, Inc. All rights reserved. All requests for copying and permission to reprint should be submitted to CCC at www.copyright.com; employ the eISSN 2327-3097 to initiate your request. See also AIAA Rights and Permissions www.aiaa.org/randp. AcknowledgmentsThis work was supported by Argonne National Laboratory through grant number ANL 4J-30361-0030A, titled “Multiscale Modeling of Complex Flows,” and by National Science Foundation through grant number 1854815, titled “High-Performance Computing and Data-Driven Modeling of Aircraft Contrails,” awarded to R. Paoli.PDF Received12 February 2021Accepted3 June 2021Published online14 July 2021
更多查看译文
AI 理解论文
溯源树
样例
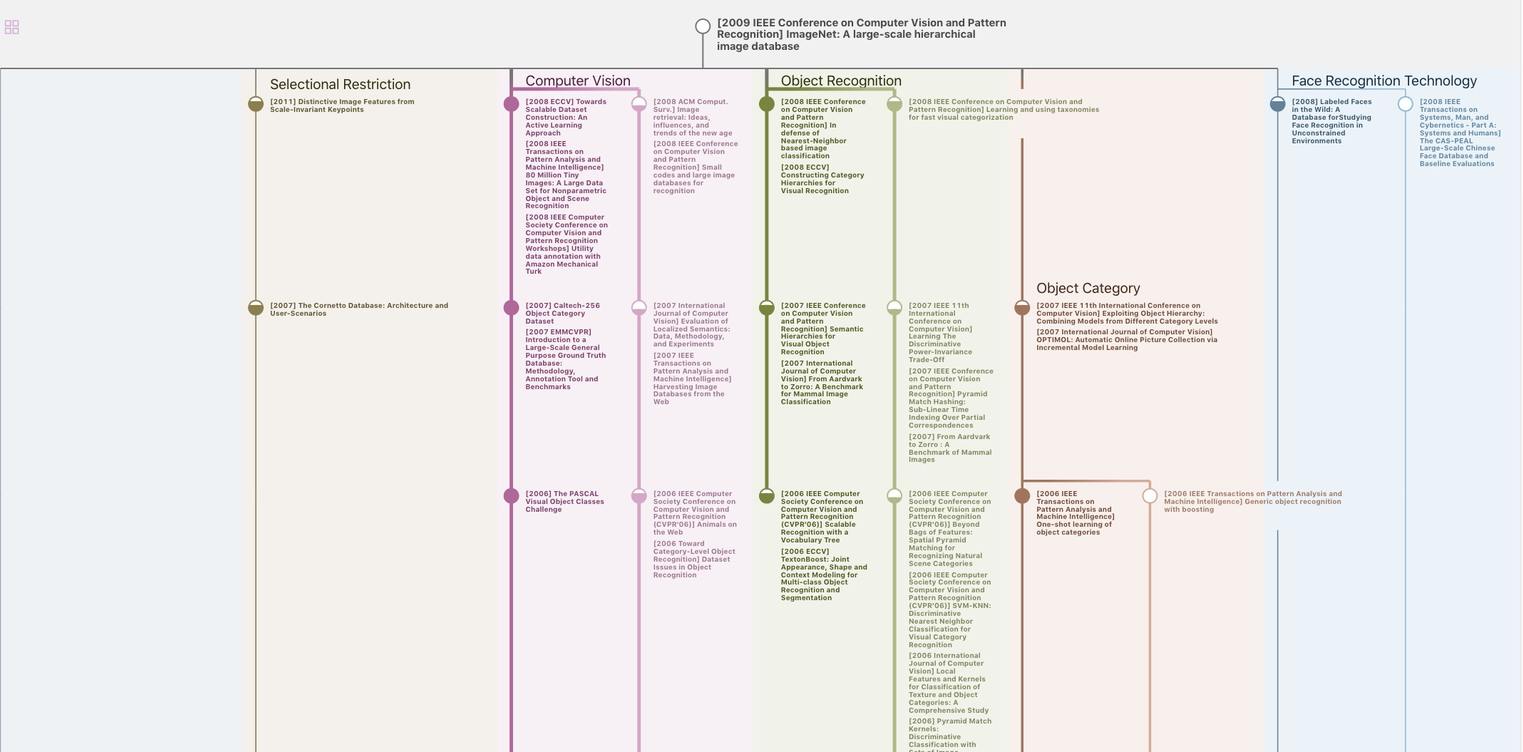
生成溯源树,研究论文发展脉络
Chat Paper
正在生成论文摘要