Carton dataset synthesis method for loading-and-unloading carton detection based on deep learning
The International Journal of Advanced Manufacturing Technology(2022)
摘要
The efficiency of truck loading-and-unloading tasks has become one of the major problems in logistics transportation, and intelligent loading-and-unloading robots are considered as effective solutions to this problem. Recently, carton detection with deep learning is widely used for those robots; however, lack of large annotated datasets is one of major impediments for rapidly deploying carton detection models. We currently have presented the Sacked Carton Dataset (SCD) that contains carton images from four logistics scenarios, such as Comprehensive Pharmaceutical Logistics Company (CPLC), E-commerce Logistics Company (ECLC), Fruit Market (FM), and Large Furniture Market (LFM). However, due to the domain shift, the carton detection model trained with source domain (which are annotated) has poor generalization ability when applied to the target domain (which are not annotated and from new logistics scenarios). To solve this problem, a novel image synthesis method is proposed to quickly acquire a labeled dataset, which replace the foreground texture of the source datasets with the texture of the target datasets. In this paper, a Local Surfaces Segmentation Algorithm is proposed to extract the carton stacking skeleton and an Invisible Contour Reconstruction Algorithm is proposed to complete the contour of the carton. Our method can keep the context relationship of foreground objects and backgrounds unchanged, and it also can greatly augment the target datasets. Code is available here .
更多查看译文
关键词
Domain adaptation,Synthetic data,Carton detection,Intelligent logistics robot
AI 理解论文
溯源树
样例
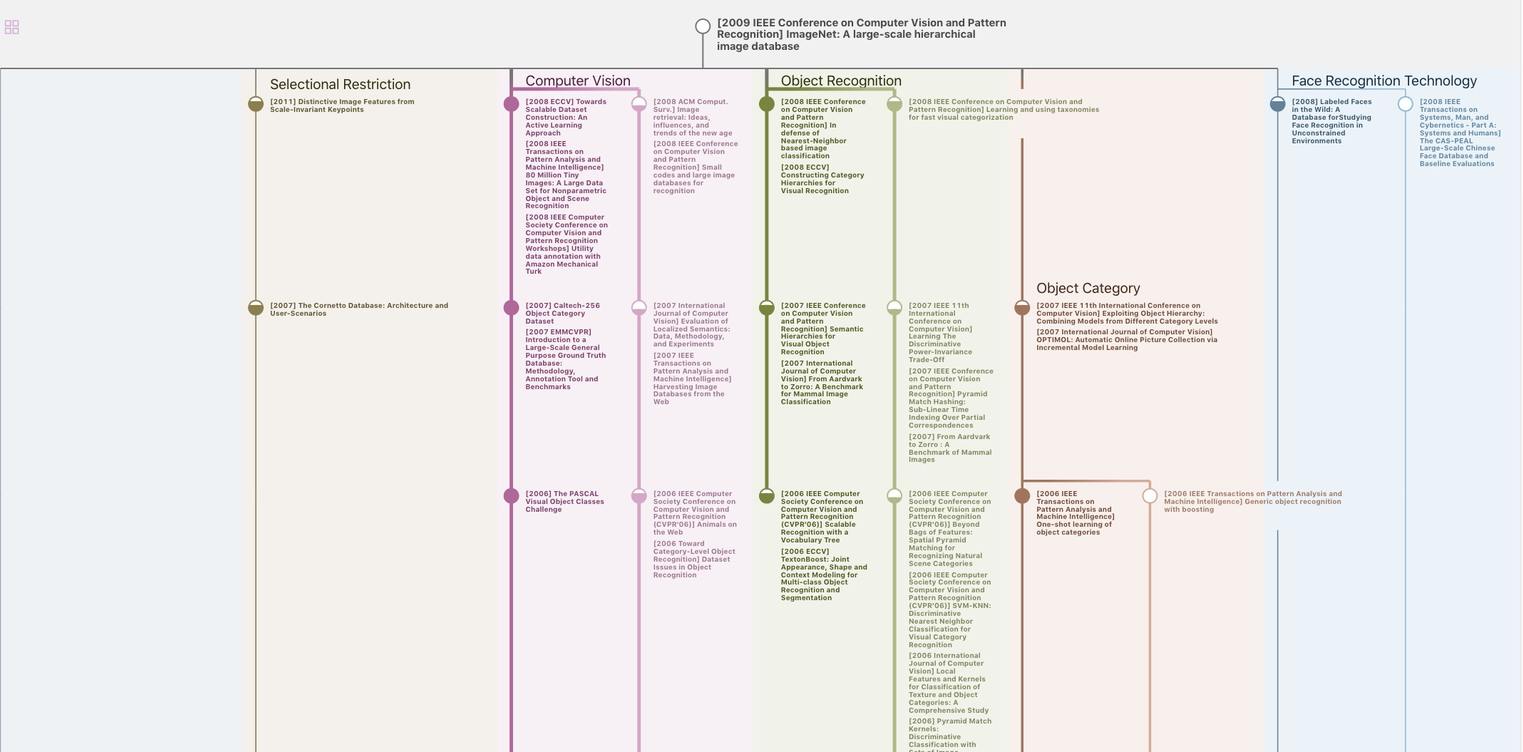
生成溯源树,研究论文发展脉络
Chat Paper
正在生成论文摘要