Large-scale structural health monitoring using composite recurrent neural networks and grid environments
COMPUTER-AIDED CIVIL AND INFRASTRUCTURE ENGINEERING(2023)
Abstract
The demand for resilient and smart structures has been rapidly increasing in recent decades. With the occurrence of the big data revolution, research on data-driven structural health monitoring (SHM) has gained traction in the civil engineering community. Unsupervised learning, in particular, can be directly employed solely using field-acquired data. However, the majority of unsupervised learning SHM research focuses on detecting damage in simple structures or components and possibly low-resolution damage localization. In this study, an unsupervised learning, novelty detection framework for detecting and localizing damage in large-scale structures is proposed. The framework relies on a 5D, time-dependent grid environment and a novel spatiotemporal composite autoencoder network. This network is a hybrid of autoencoder convolutional neural networks and long short-term memory networks. A 10-story, 10-bay, numerical structure is used to evaluate the proposed framework damage diagnosis capabilities. The framework was successful in diagnosing the structure health state with average accuracies of 93% and 85% for damage detection and localization, respectively.
MoreTranslated text
Key words
structural health monitoring,composite recurrent neural networks
AI Read Science
Must-Reading Tree
Example
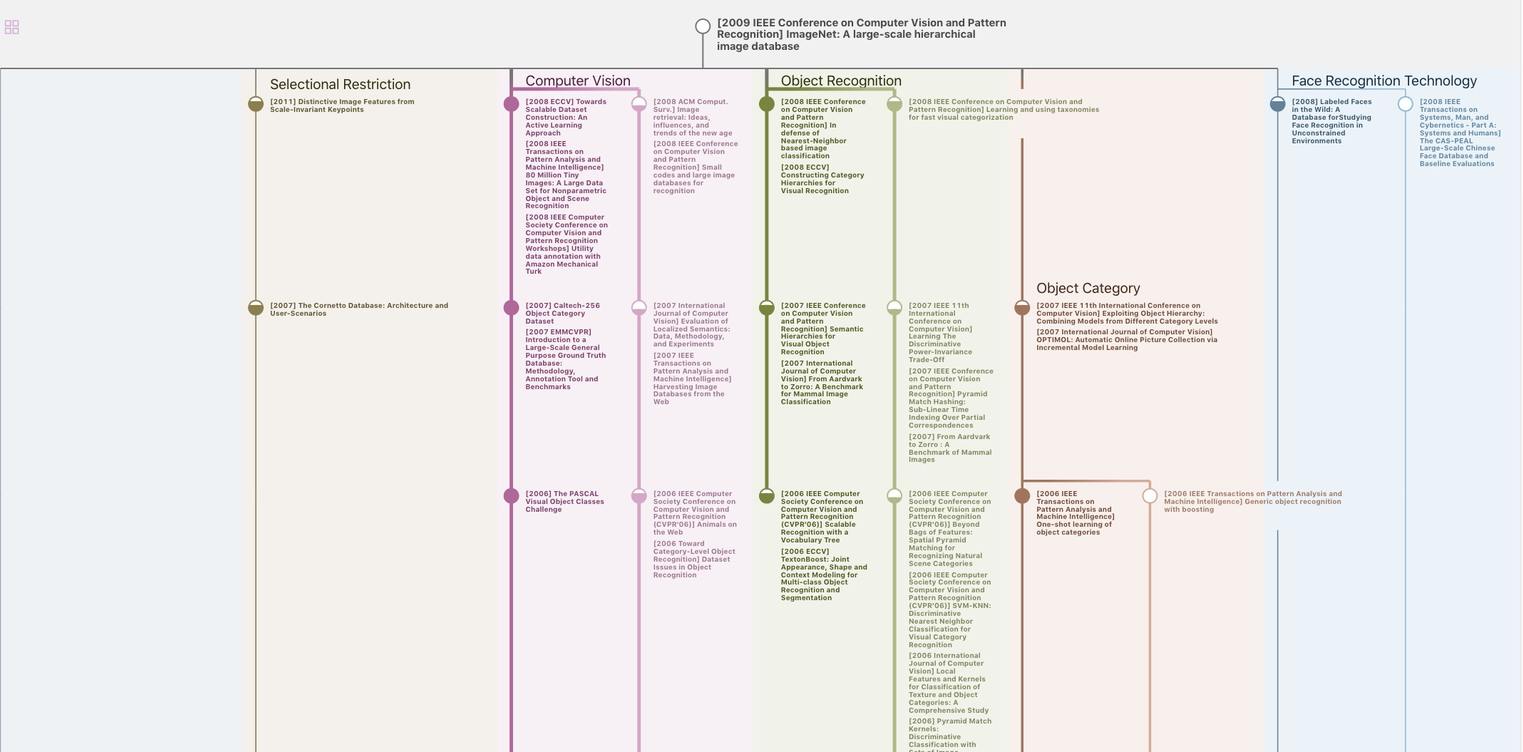
Generate MRT to find the research sequence of this paper
Chat Paper
Summary is being generated by the instructions you defined