A Scoping Review on COVID-19's Early Detection Using Deep Learning Model and Computed Tomography and Ultrasound
TRAITEMENT DU SIGNAL(2022)
摘要
Since the end of 2019, a COVID-19 outbreak has put healthcare systems worldwide on edge. In rural areas, where traditional testing is unfeasible, innovative computer-aided diagnostic approaches must deliver speedy and cost-effective screenings. Conducting a full scoping review is essential for academics despite several studies on the use of Deep Learning (DL) to combat COVID-19. This review examines the application of DL techniques in CT and ULS images for the early detection of COVID-19. In this review, the PRISMA literature review approach was followed. All studies are retrieved from IEEE, ACM, Medline, and Science Direct. Performance metrics were highlighted for each study to measure the proposed solutions' performance and conceptualization; A set of publicly available datasets were appointed; DL architectures based on more than one image modality such as CT and ULS are explored. Out of 32 studies, the combined U-Net segmentation and 3D classification VGG19 network had the best F1 score (98%) on ultrasound images, while ResNet-101 had the best accuracy (99.51%) on CT images for COVID-19 detection. Hence, data augmentation techniques such as rotation, flipping, and shifting were frequently used. Grad-CAM was used in eight studies to identify anomalies on the lung surface. Our research found that transfer learning outperformed all other AI-based prediction approaches. Using a UNET with a predefined backbone, like VGG19, a practical computer-assisted COVID-19 screening approach can be developed. More collaboration is required from healthcare professionals and the computer science community to provide an efficient deep learning framework for the early detection of COVID-19.
更多查看译文
关键词
COVID-19, deep learning, computed tomography CT, ultrasound ULS, early detection
AI 理解论文
溯源树
样例
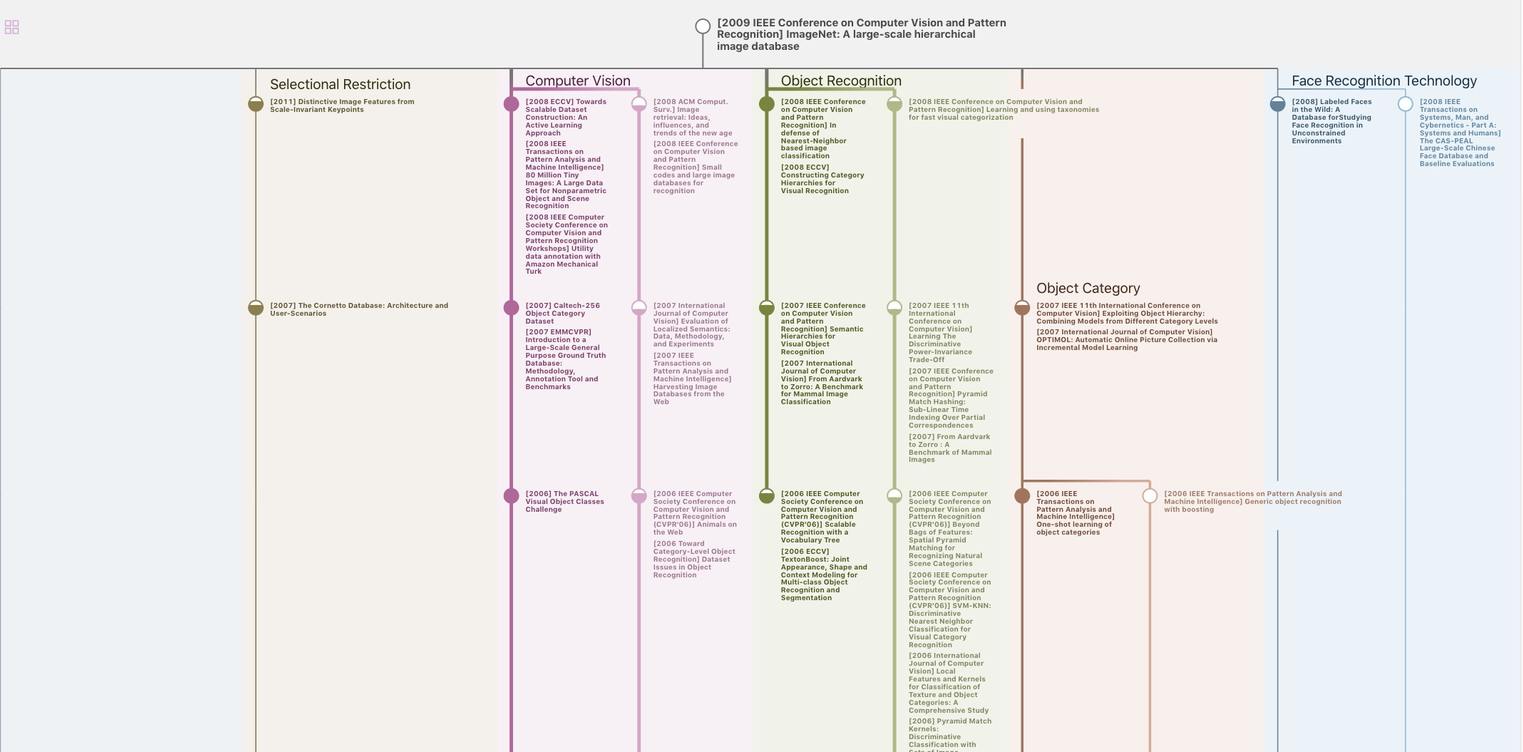
生成溯源树,研究论文发展脉络
Chat Paper
正在生成论文摘要