SYSTEMATIC REVIEW OF LITERATURE EVALUATING MACHINE LEARNING ALGORITHMS TO DEVELOP OUTCOME PREDICTION MODELS IN GLIOMA USING MOLECULAR IMAGING WITH AMINO ACID PET
NEURO-ONCOLOGY(2021)
Abstract
Abstract PURPOSE Machine learning (ML) algorithms demonstrate accurate prediction of tumor segmentation, molecular pathology, and outcomes in gliomas using MRI and recently application of ML tools has expanded into molecular imaging with PET. We performed a systematic review to evaluate the role and applications of ML in characterization of gliomas with PET. METHODS Four databases were searched by medical school librarian and confirmed by an independent librarian: Ovid Embase, Ovid MEDLINE, Cochrane trials (CENTRAL), and Web of Science-Core Collection. The search strategy used keywords and controlled vocabulary combining the terms for: artificial intelligence, machine learning, deep learning, radiomics, magnetic resonance imaging, glioma, and related terms. All articles were reviewed by at least 2 independent reviewers at abstract screening, full text review, data extraction, and bias analysis using TRIPOD. RESULTS An initial 11,727 publications were imported to Covidence for screening. After review, 1135 studies moved to full-text review and 715 articles were included. Twelve publications included PET imaging of gliomas. All publications used single-center databases (3-73 patients) with distribution of tracers being [18F]-FDG (1), [18F]-FET (6), [11C]-MET (3), [18F]-FDOPA (1), and [18F]-AMP (1). All but 2 papers used supervised machine learning algorithms. Number of features ranged from 4-19,284. Nine papers manually extracted semiquantitative features TBRmax, TBRmean, SUV, TTP, in addition to demographics. Study outcomes included prediction of treatment response, survival, molecular subtypes, tumor grade, segmentation, and accuracy of image fusion. Accuracy ranged from 0.64-0.95 with AUC 0.43-0.9. CONCLUSION ML can be used on small datasets of PET imaging of brain tumors. While majority of the clinical scans are performed with FDG-PET, the machine learning approaches are being applied to mostly amino acid tracers. Extending ML approaches to FDG-PET, which is more common in clinical practice, is recommended. Overall, ML has potential as a useful tool for predicting patient outcomes and improving image postprocessing.
MoreTranslated text
Key words
Cancer Imaging,Predictive Modeling,Machine Learning,Medical Imaging,Imaging
AI Read Science
Must-Reading Tree
Example
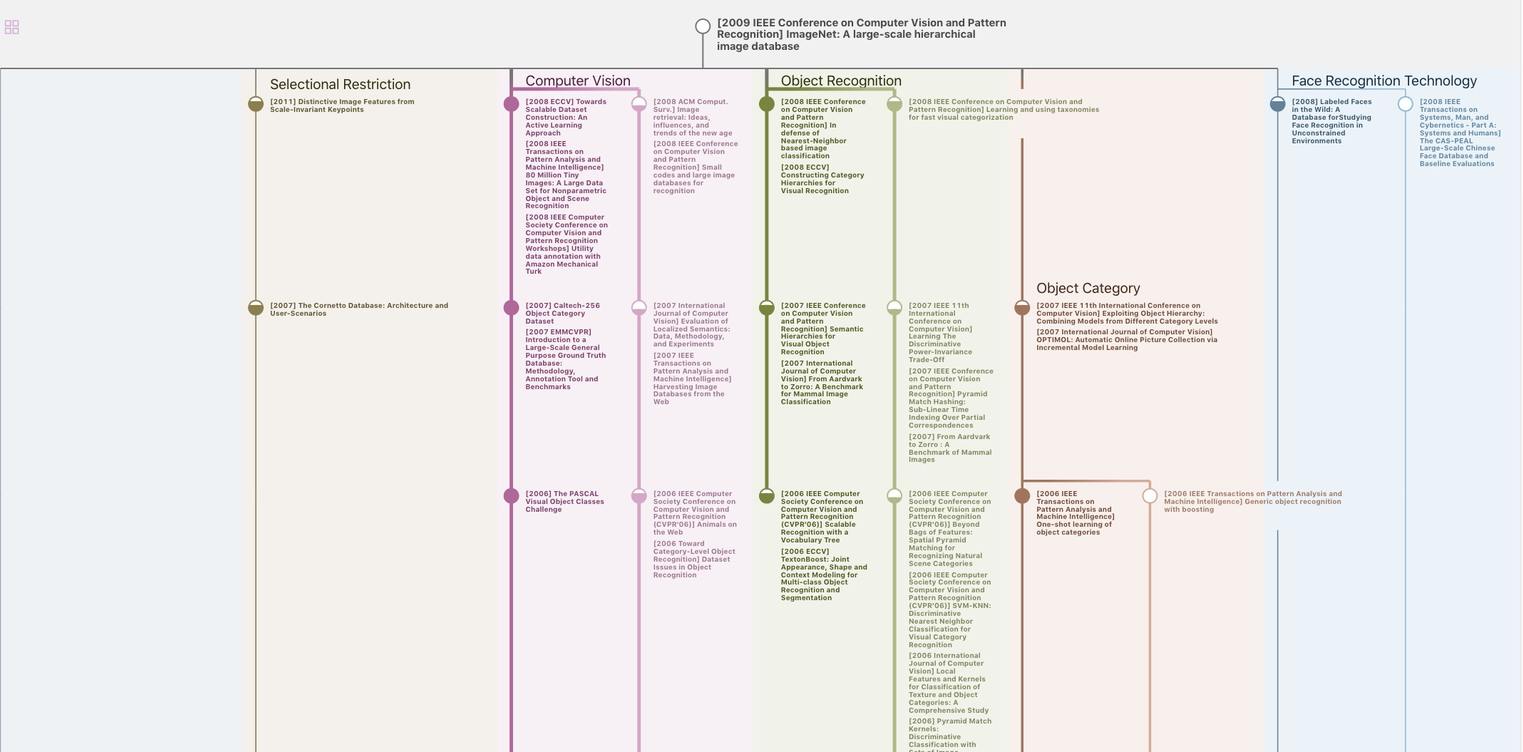
Generate MRT to find the research sequence of this paper
Chat Paper
Summary is being generated by the instructions you defined