Prediction and multi-objective optimization of mechanical, economical, and environmental properties for strain-hardening cementitious composites (SHCC) based on automated machine learning and metaheuristic algorithms
Journal of Cleaner Production(2021)
Abstract
This study develops a framework for property prediction and multi-objective optimization of strain-hardening cementitious composites (SHCC) based on automated machine learning. Three machine learning models are developed to predict the compressive strength, tensile strength, and ductility of SHCC. A tree-based pipeline optimization method is enhanced and used to enable automatic configuration of machine learning models, which are trained using three datasets considering 14 mix design variables and achieve reasonable prediction accuracy. With the predictive models, five objective functions are formulated for mechanical properties, life-cycle cost, and carbon footprint of SHCC, and the five objective functions are optimized in six design scenarios. The objective functions are optimized using innovative optimization and decision-making techniques (Unified Non-dominated Sorting Genetic Algorithm III and Technique for Order of Preference by Similarity to Ideal Solution). This research will promote efficient development and applications of high-performance SHCC in concrete and construction industry.
MoreTranslated text
Key words
Automated machine learning,Carbon footprint,Evolutionary algorithm,Multi-objective optimization,Strain-hardening cementitious composite (SHCC),Tree-based pipeline optimization
AI Read Science
Must-Reading Tree
Example
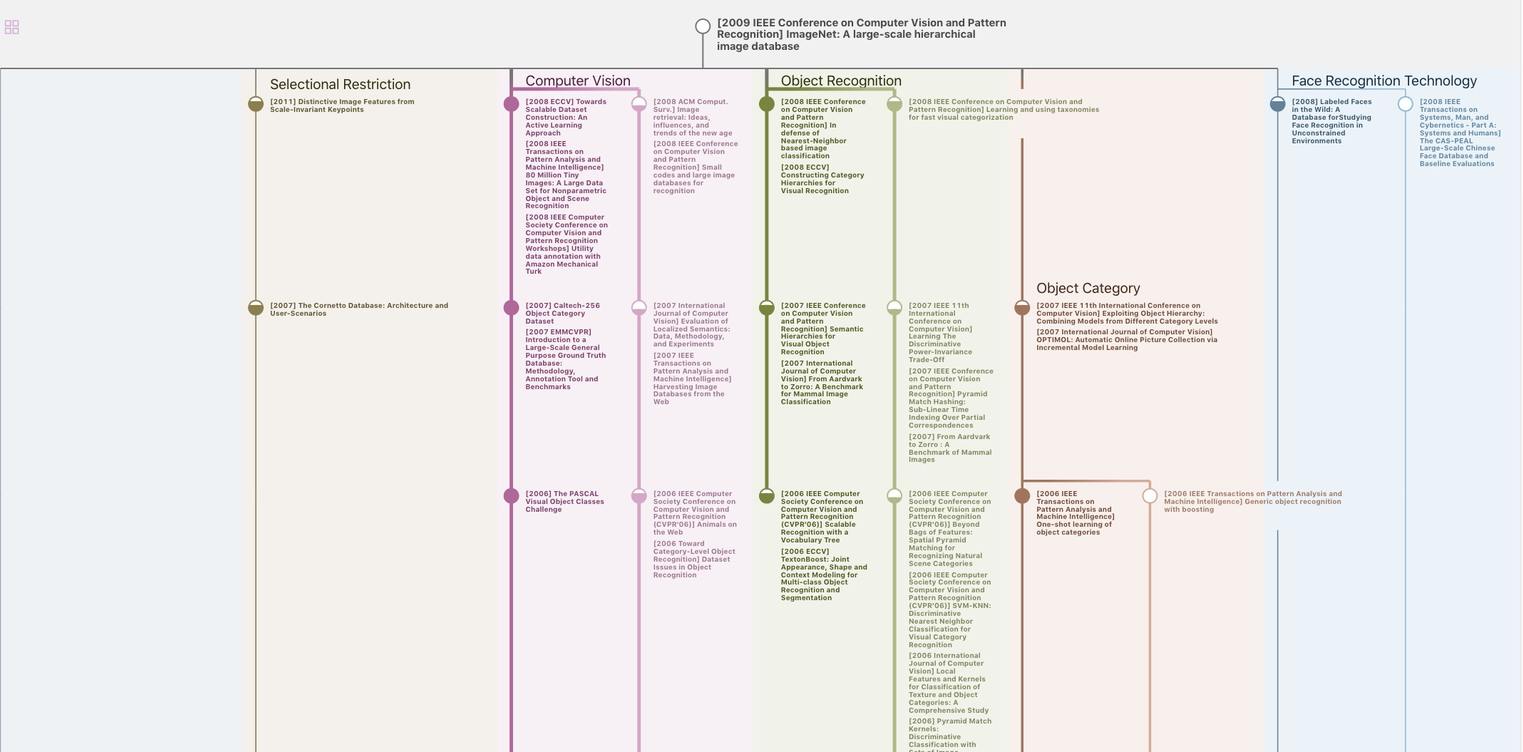
Generate MRT to find the research sequence of this paper
Chat Paper
Summary is being generated by the instructions you defined