Machine Learning Based Quantitative Damage Monitoring of Composite Structure
INTERNATIONAL JOURNAL OF SMART AND NANO MATERIALS(2022)
摘要
Composite materials have been widely used in many industries due to their excellent mechanical properties. It is difficult to analyze the integrity and durability of composite structures because of their own characteristics and the complexity of load and environments. Structural health monitoring (SHM) based on built-in sensor networks has been widely evaluated as a method to improve the safety and reliability of composite structures and reduce the operational cost. With the rapid development of machine learning, a large number of machine learning algorithms have been applied in many disciplines, and also are being applied in the field of SHM to avoid the limitations resulting from the need of physical models. In this paper, the damage monitoring technologies often used for composite structures are briefly outlined, and the applications of machine learning in damage monitoring of composite structures are concisely reviewed. Then, challenges and solutions for quantitative damage monitoring of composite structures based on machine learning are discussed, focusing on the complete acquisition of monitoring data, deep analysis of the correlation between sensor signal eigenvalues and composite structure states, and quantitative intelligent identification of composite delamination damage. Finally, the development trend of machine learning-based SHM for composite structures is discussed.
更多查看译文
关键词
Composite structure, structural health monitoring, machine learning, built-in sensor network, structural damage
AI 理解论文
溯源树
样例
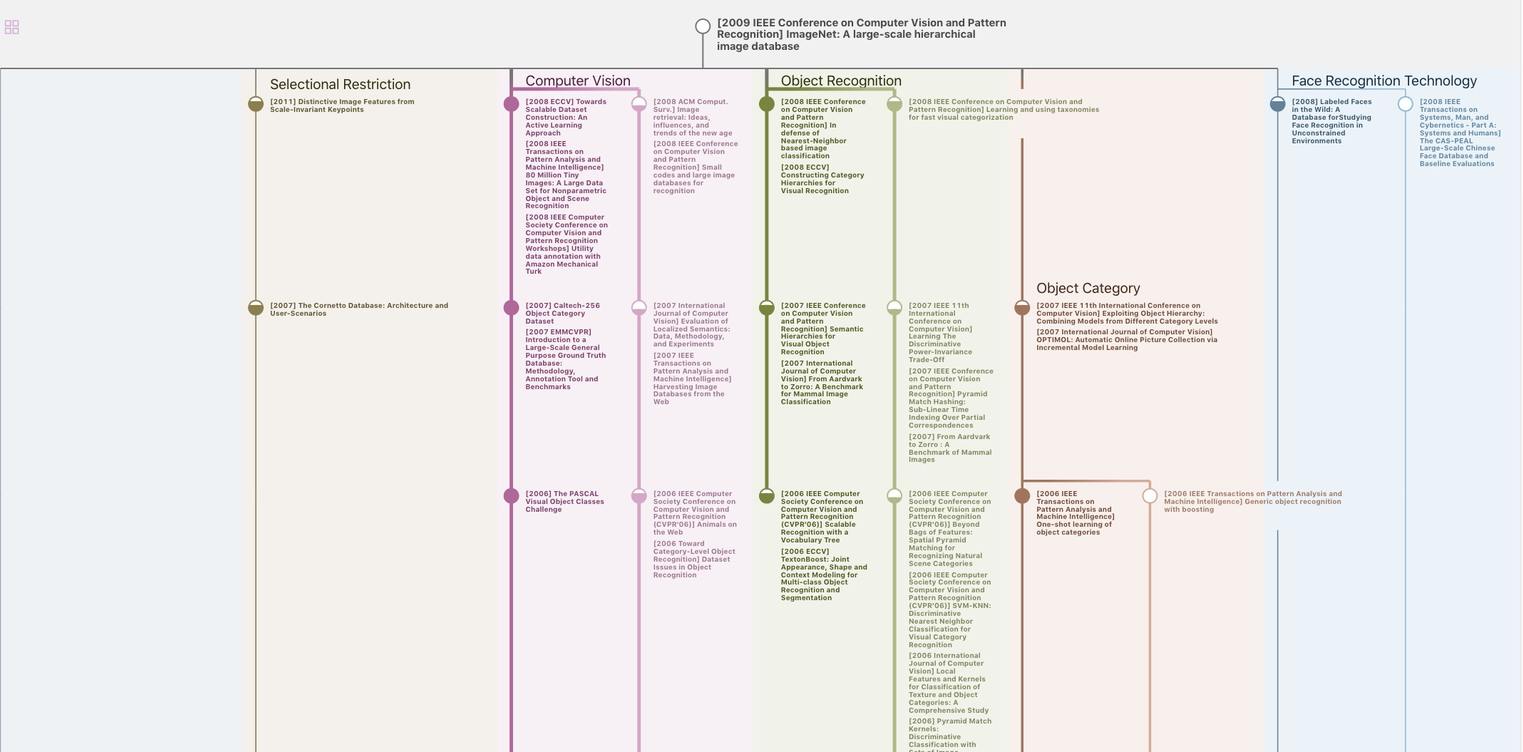
生成溯源树,研究论文发展脉络
Chat Paper
正在生成论文摘要