Crack segmentation in high-resolution images using cascaded deep convolutional neural networks and Bayesian data fusion
SMART STRUCTURES AND SYSTEMS(2022)
Abstract
Manual inspection of steel box girders on long span bridges is time-consuming and labor-intensive. The quality of inspection relies on the subjective judgements of the inspectors. This study proposes an automated approach to detect and segment cracks in high-resolution images. An end-to-end cascaded framework is proposed to first detect the existence of cracks using a deep convolutional neural network (CNN) and then segment the crack using a modified U-Net encoder-decoder architecture. A Naive Bayes data fusion scheme is proposed to reduce the false positives and false negatives effectively. To generate the binary crack mask, first, the original images are divided into 448 x 448 overlapping image patches where these image patches are classified as cracks versus non-cracks using a deep CNN. Next, a modified U-Net is trained from scratch using only the crack patches for segmentation. A customized loss function that consists of binary cross entropy loss and the Dice loss is introduced to enhance the segmentation performance. Additionally, a Naive Bayes fusion strategy is employed to integrate the crack score maps from different overlapping crack patches and to decide whether a pixel is crack or not. Comprehensive experiments have demonstrated that the proposed approach achieves an 81.71% mean intersection over union (mIoU) score across 5 different training/test splits, which is 7.29% higher than the baseline reference implemented with the original U-Net.
MoreTranslated text
Key words
Bayesian data fusion, crack detection, deep learning, semantic segmentation, structural health monitoring
AI Read Science
Must-Reading Tree
Example
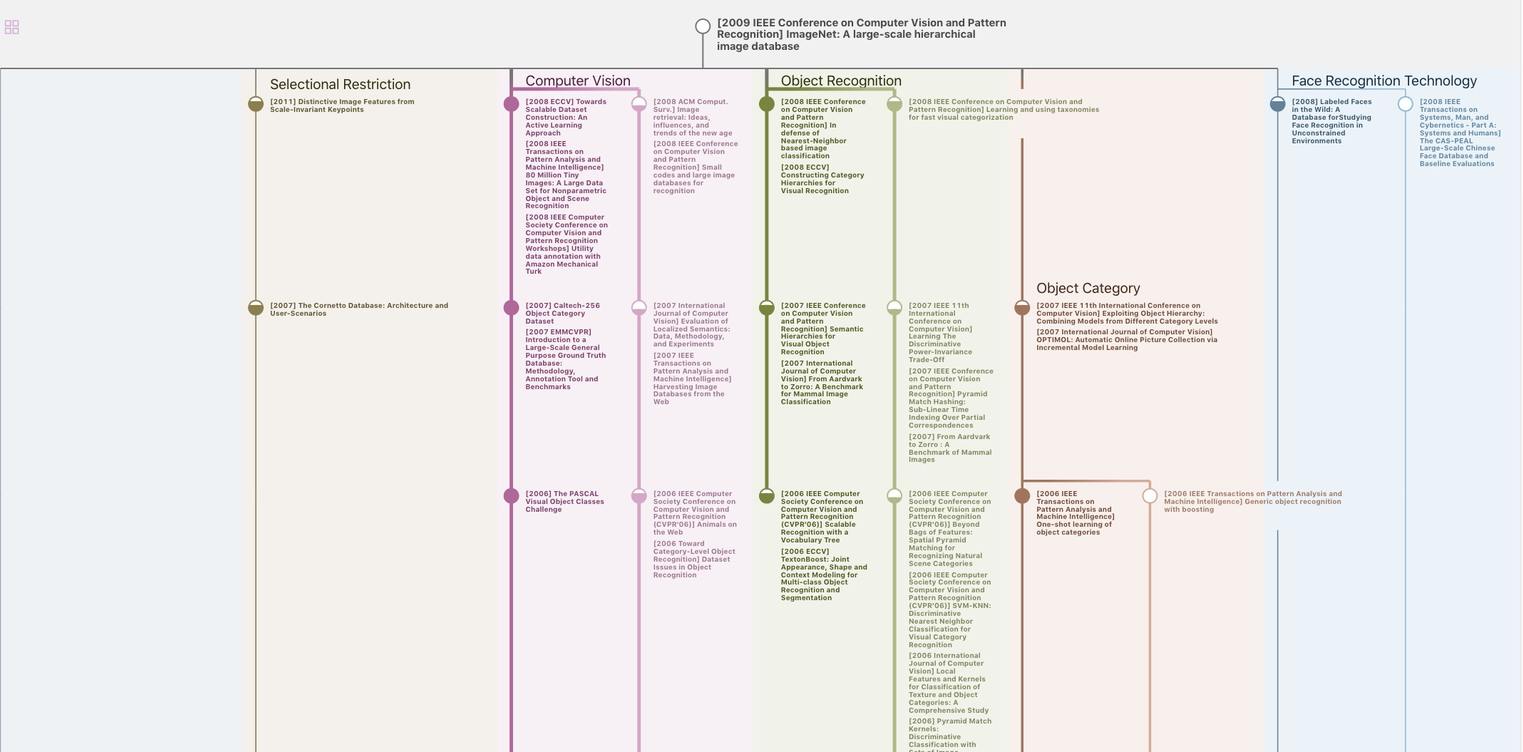
Generate MRT to find the research sequence of this paper
Chat Paper
Summary is being generated by the instructions you defined