Multivariate skew Laplace normal distribution for modeling skewness and heavy-tailedness in multivariate data sets
STATISTICS AND ITS INTERFACE(2022)
Abstract
Modeling both skewness and heavy-tailedness in multivariate data sets is a challenging problem. The main goal of this paper is to introduce a multivariate skew Laplace normal (MSLN) distribution to deal with the issue by providing a flexible model for modeling skewness and heavy-tailedness simultaneously. This distribution will be an alternative to some multivariate skew distributions including the multivariate skew-t-normal (MSTN) distribution introduced by [28]. This is due to the fact that the MSLN distribution has fewer parameters than most of these distributions, which causes computationally advantageous for the MSLN distribution over these distributions. The definition, some distributional properties of this distribution are studied. The maximum likelihood (ML) estimators for the parameters of the MSLN distribution are obtained via the expectation-maximization (EM) algorithm. A simulation study and a real data example are also provided to illustrate the capability of the MSLN distribution for modeling data sets in multivariate settings.
MoreTranslated text
Key words
EM algorithm, ML estimation, MSGLN, MSLN, MSTN
AI Read Science
Must-Reading Tree
Example
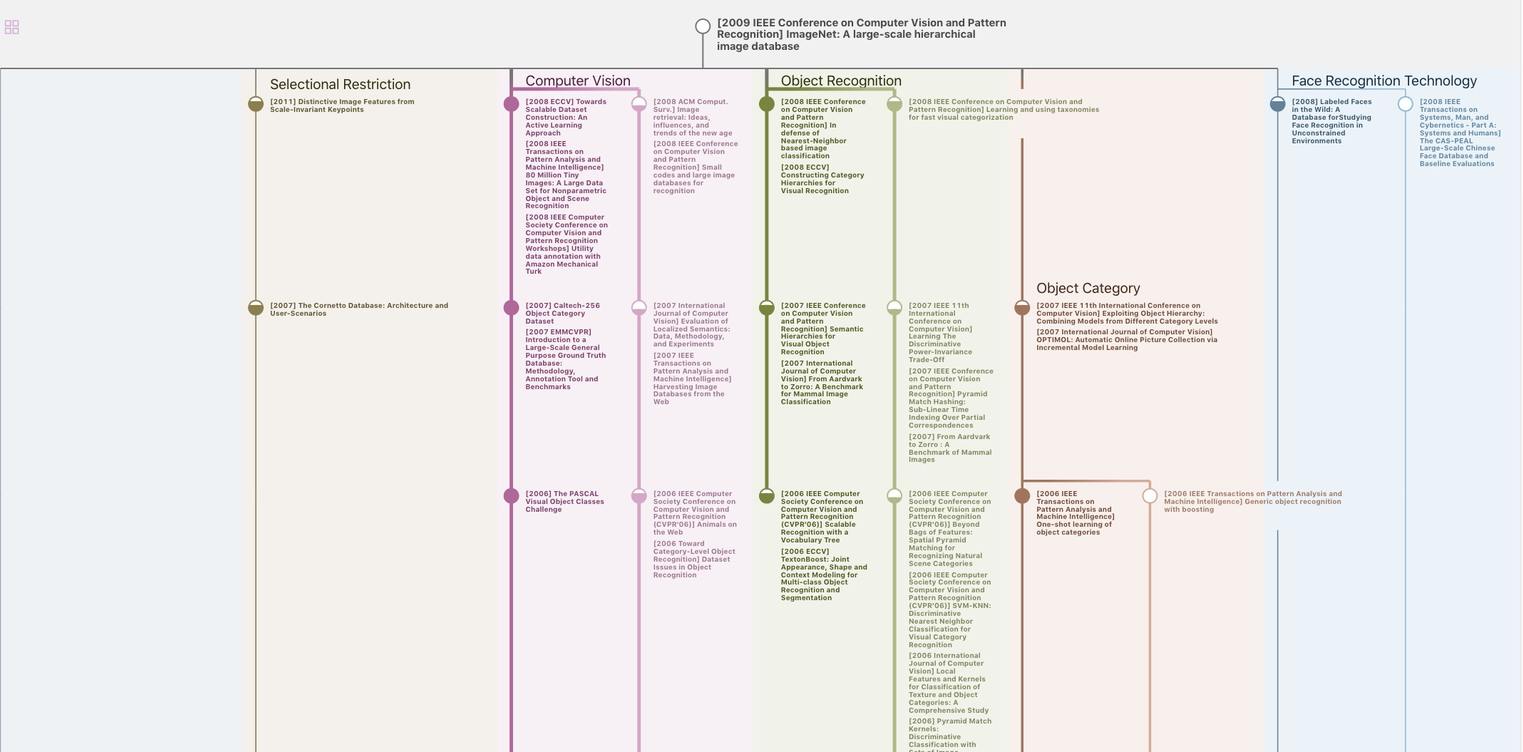
Generate MRT to find the research sequence of this paper
Chat Paper
Summary is being generated by the instructions you defined