GPR image denoising with NSST-UNET and an improved BM3D
DIGITAL SIGNAL PROCESSING(2022)
摘要
To suppress random noise while preserving effective information in the edge areas of ground penetrating radar (GPR) images, this paper proposes a novel denoising method by making use of a deep neural network called NSST-UNET and an improved BM3D. At first, NSST-UNET is designed with a nonsubsampled shearlet transform (NSST) coding layer and a skip connection based on a multi-scale convolution module and applied to identify the edge and smooth areas of noisy GPR images. Then, the denoising is accomplished with the improved BM3D in two steps. In the first step, a larger search range for similar blocks and a soft threshold are used to denoise the edge and smooth areas, respectively. In the second step, the Wiener filter optimized by mean square error and the Wiener filter optimized by structural similarity are utilized to denoise the smooth and unsmooth areas, respectively. Finally, the excellent denoising performance of the proposed method is verified by qualitative and quantitative analysis with simulation and field exploration data. (C)& nbsp;2022 Elsevier Inc. All rights reserved.
更多查看译文
关键词
GPR, Image denoising, Deep neural network, BM3D, Non-subsampled shearlet transform
AI 理解论文
溯源树
样例
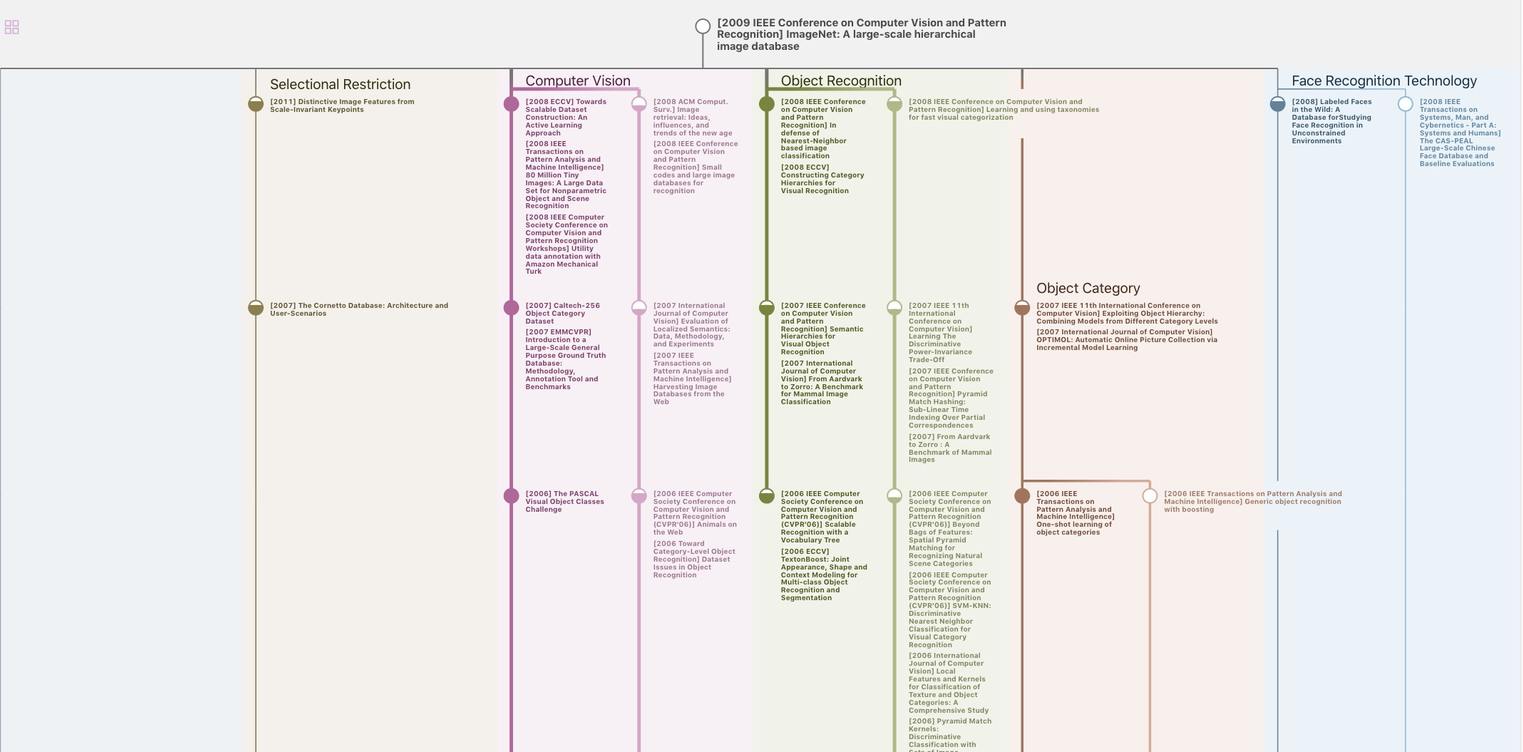
生成溯源树,研究论文发展脉络
Chat Paper
正在生成论文摘要