Segmentation of Individual Leaves of Field Grown Sugar Beet Plant Based on 3D Point Cloud
AGRONOMY-BASEL(2022)
摘要
Accurate segmentation of individual leaves of sugar beet plants is of great significance for obtaining the leaf-related phenotypic data. This paper developed a method to segment the point clouds of sugar beet plants to obtain high-quality segmentation results of individual leaves. Firstly, we used the SFM algorithm to reconstruct the 3D point clouds from multi-view 2D images and obtained the sugar beet plant point clouds after preprocessing. We then segmented them using the multiscale tensor voting method (MSTVM)-based region-growing algorithm, resulting in independent leaves and overlapping leaves. Finally, we used the surface boundary filter (SBF) method to segment overlapping leaves and obtained all leaves of the whole plant. Segmentation results of plants with different complexities of leaf arrangement were evaluated using the manually segmented leaf point clouds as benchmarks. Our results suggested that the proposed method can effectively segment the 3D point cloud of individual leaves for field grown sugar beet plants. The leaf length and leaf area of the segmented leaf point clouds were calculated and compared with observations. The calculated leaf length and leaf area were highly correlated with the observations with R-2 (0.80-0.82). It was concluded that the MSTVM-based region-growing algorithm combined with SBF can be used as a basic segmentation step for high-throughput plant phenotypic data extraction of field sugar beet plants.
更多查看译文
关键词
3D point cloud, region-growing algorithm, multiscale tensor voting method (MSTVM), phenotyping
AI 理解论文
溯源树
样例
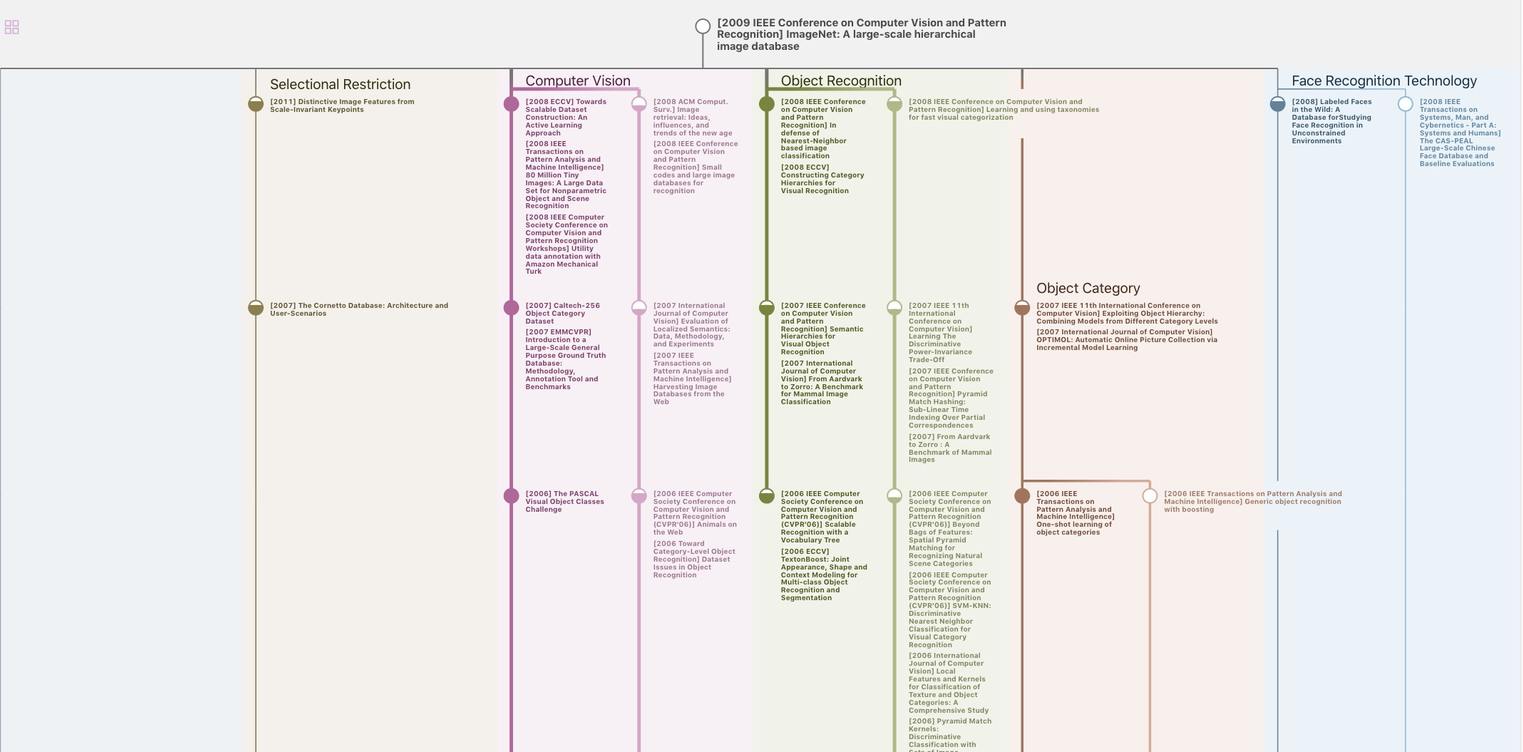
生成溯源树,研究论文发展脉络
Chat Paper
正在生成论文摘要